Advances in Process Safety and Protection of Cyber-Physical Systems (CPS)
A special issue of Processes (ISSN 2227-9717). This special issue belongs to the section "Automation Control Systems".
Deadline for manuscript submissions: closed (15 May 2023) | Viewed by 9025
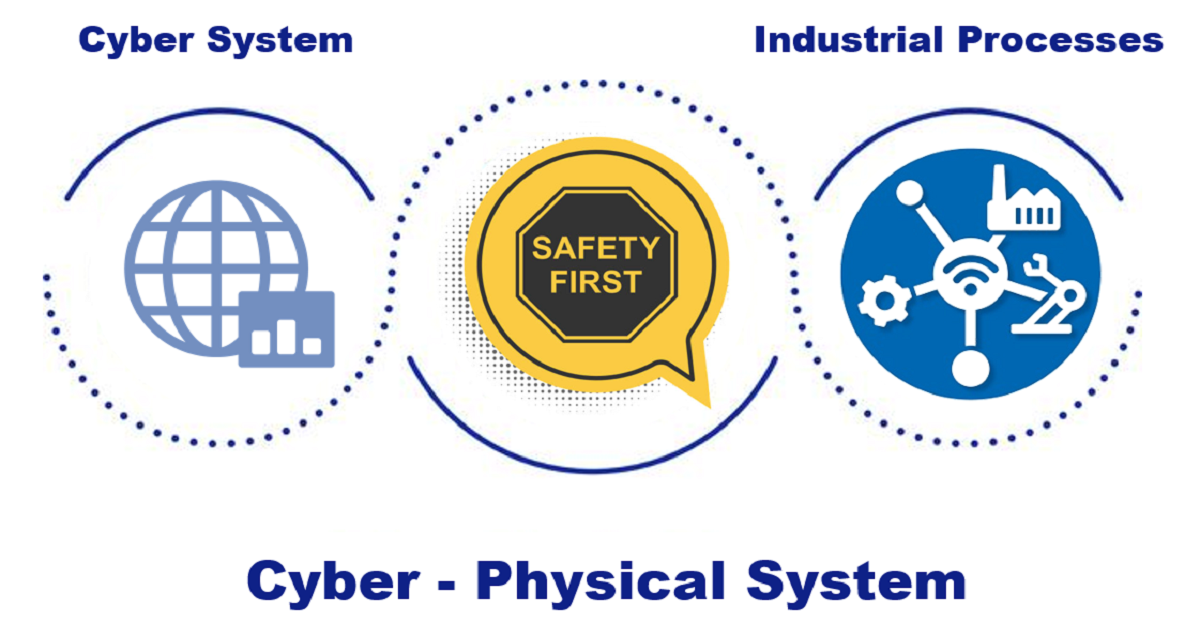
Special Issue Editors
Interests: cyber-physical systems; safety and security; risk assessment
Interests: process control; self-optimal control; process monitoring
Special Issue Information
Dear Colleagues,
Process safety has been a top priority in process systems engineering for decades. With the increase of process complexity and the involvement of the Internet in process industries, unintentional man-made disasters are happening more often and causing serious consequences. Fortunately, many innovative technologies are being deployed in real process industries, including mathematical-model-based approaches, machine learning algorithms, data driven approaches, etc. With the development of industrial Internet technologies, more and more traditional process industries are directly or indirectly connected with external networks, making them cyber-physical systems (CPSs)—that is, the “cyber” aspect is becoming a part of the industrial processes. Benefiting the advantages from the involvement of the Internet in CPSs, serious safety risks could be also created alongside the benefits. This Special Issue on “Advances in Process Safety and Protection of Cyber-Physical Systems” seeks high-quality works focusing on the latest developments in process safety and technologies of cyber-physical systems protection for general industrial processes. Topics include, but are not limited to:
- Advanced process hazard analysis;
- Model-based process risk assessment and prevention;
- Machine learning for process fault diagnosis;
- Process safety monitoring;
- Safe control systems design;
- Alarm management;
- Safety protection in cyber-physical systems;
- Safety requirements analysis;
- Inherent safety of cyber-physical systems;
- Endogenous safety of cyber-physical systems;
- Intrusion detection and attacker localization for cyber-physical systems.
Prof. Dr. Shuang-Hua Yang
Prof. Dr. Yi Cao
Dr. Yulong Ding
Guest Editors
Manuscript Submission Information
Manuscripts should be submitted online at www.mdpi.com by registering and logging in to this website. Once you are registered, click here to go to the submission form. Manuscripts can be submitted until the deadline. All submissions that pass pre-check are peer-reviewed. Accepted papers will be published continuously in the journal (as soon as accepted) and will be listed together on the special issue website. Research articles, review articles as well as short communications are invited. For planned papers, a title and short abstract (about 100 words) can be sent to the Editorial Office for announcement on this website.
Submitted manuscripts should not have been published previously, nor be under consideration for publication elsewhere (except conference proceedings papers). All manuscripts are thoroughly refereed through a single-blind peer-review process. A guide for authors and other relevant information for submission of manuscripts is available on the Instructions for Authors page. Processes is an international peer-reviewed open access monthly journal published by MDPI.
Please visit the Instructions for Authors page before submitting a manuscript. The Article Processing Charge (APC) for publication in this open access journal is 2400 CHF (Swiss Francs). Submitted papers should be well formatted and use good English. Authors may use MDPI's English editing service prior to publication or during author revisions.
Keywords
- process safety
- fault diagnosis
- operation monitoring
- cyber-physical systems
- risk analysis
- loss prevention