Topic Menu
► Topic MenuTopic Editors
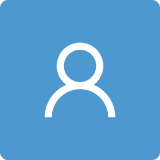
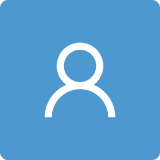
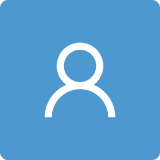
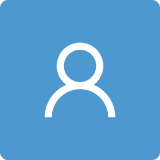
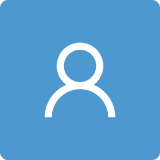

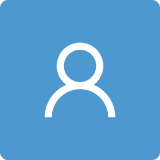
Advances in Biomedical Engineering from the Annual Conference of SEIB 2021
Topic Information
Dear Colleagues,
The Annual Congress of the Spanish Society of Biomedical Engineering, CASEIB2021, was held from November 25 to 26, 2021, and provided the opportunity for scholars to share their research work with other researchers, students, and professionals working in biomedical engineering.
As with previous editions, a Topic will be set up in the Entropy and Sensors journals and all works submitted to the contest for the José María Ferrero Corral awards will be eligible. This Topic will collect articles referring to the theoretical development and application of basic and advanced aspects of entropy, complexity, and information theory, among others. It will also collect articles related to sensor manufacturing technologies and processes, as well as their packaging and applications.
Topics of interest include, but are not limited to, the following:
- Entropy and complexity metrics;
- Information theory tools;
- Nonlinear analysis of temporal dynamics;
- Pattern recognition, encoding, and compression;
- Artificial intelligence, deep learning, machine learning, big data;
- New technologies for biosignal sensing;
- Lab-on-a-chip;
- Telemedicine and telemonitoring of patients;
- Sensor technology and applications in biomedical engineering;
- Micro and nanosensors in biomedical engineering;
- Wearable sensors, devices, and electronics;
- Signal processing, data fusion, and deep learning in sensor systems;
- Processing algorithms of medical imaging;
- New technologies in simulation, monitoring, and surgical planning;
- Localization and object tracking;
- Sensing and imaging.
The original article presented at CASEIB 2021 must be cited and the final manuscript must extend the original one, with both differing by at least 50%. The deadline for submitting papers to this Topic is August 30, 2022.
We hope that this initiative will be of interest to you and that we can help to disseminate the excellent works sent to CASEIB2021.
Prof. Dr. Raúl Alcaraz
Dr. Elisabete Aramendi
Dr. Raimon Jane
Dr. Gema García-Sáez
Dr. Gema Prats-Boluda
Dr. Javier Reina-Tosina
Prof. Dr. Roberto Hornero
Dr. Patricia Sánchez-González
Topic Editors
Participating Journals
Journal Name | Impact Factor | CiteScore | Launched Year | First Decision (median) | APC |
---|---|---|---|---|---|
![]()
Entropy
|
2.0 | 5.2 | 1999 | 21.8 Days | CHF 2600 |
![]()
Sensors
|
3.5 | 8.2 | 2001 | 19.7 Days | CHF 2600 |
![]()
Bioengineering
|
3.7 | 5.3 | 2014 | 19.2 Days | CHF 2700 |
![]()
Diagnostics
|
3.3 | 5.9 | 2011 | 21 Days | CHF 2600 |
Preprints.org is a multidisciplinary platform offering a preprint service designed to facilitate the early sharing of your research. It supports and empowers your research journey from the very beginning.
MDPI Topics is collaborating with Preprints.org and has established a direct connection between MDPI journals and the platform. Authors are encouraged to take advantage of this opportunity by posting their preprints at Preprints.org prior to publication:
- Share your research immediately: disseminate your ideas prior to publication and establish priority for your work.
- Safeguard your intellectual contribution: Protect your ideas with a time-stamped preprint that serves as proof of your research timeline.
- Boost visibility and impact: Increase the reach and influence of your research by making it accessible to a global audience.
- Gain early feedback: Receive valuable input and insights from peers before submitting to a journal.
- Ensure broad indexing: Web of Science (Preprint Citation Index), Google Scholar, Crossref, SHARE, PrePubMed, Scilit and Europe PMC.