Topic Menu
► Topic MenuTopic Editors
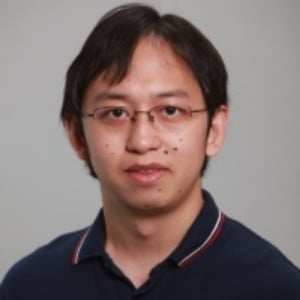
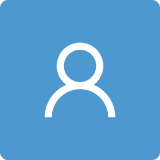
Research and Application of Artificial Intelligence in Wind and Wave Energy
Topic Information
Dear Colleagues,
Renewable energy utilization is widely recognized as one of the main strategies to reduce civilization’s carbon footprint and prevent the most deleterious impacts of climate change. Wind energy is a mature technology that in many locations offers the lowest cost for electric generation. Wind turbines are exponentially growing in power generation capacity in offshore locations, increasingly supplying a larger proportion of electricity consumption. Simultaneously, wave energy is being developed and tested at an accelerated pace in many locations, considering its capability of replacing large portions of current hydrocarbon electricity generation. Collocating harvesters for both wind and wave has been studied with great interest, providing significant benefits and synergies. However, many of the challenges presented by wind and wave energy are difficult to address by applying traditional methods. Selecting optimal placements, layouts, equipment, equipment mixture and collocation, operational times, deactivations, energy curtailing and maintenance strategies are in most cases NP-hard problems. Applications and development of artificial intelligence (AI) in wind and wave energy have shown a number of advantages, generating optimal solutions that can be implemented in real-world scenarios. Furthermore, these solutions may be implemented in diverse geographical locations and for diverse power generation demands with more flexibility. The aim of this Topic is to provide researchers, practitioners, developers, organizations, and governmental agencies with a platform to present the work they are performing in the research and application of artificial intelligence in wind and wave energy. This Topic is open to all lifecycle stages of wind and wave energy utilization, including prediction, design, manufacturing, harvesting, operation and control, and transmission, with the goal to advance more sustainable renewable energy generation and help to reduce greenhouse gases emission worldwide in an accelerated timeline.
Prof. Dr. Hua Li
Dr. Francisco Haces-Fernandez
Topic Editors
Keywords
- wind energy
- wave energy
- wind and wave energy collocation
- optimization
- artificial intelligence (AI)
- offshore renewable energy
- site selection
- layout optimization
Participating Journals
Journal Name | Impact Factor | CiteScore | Launched Year | First Decision (median) | APC |
---|---|---|---|---|---|
![]()
Energies
|
3.2 | 5.5 | 2008 | 16.1 Days | CHF 2600 |
![]()
Journal of Marine Science and Engineering
|
2.9 | 3.7 | 2013 | 15.4 Days | CHF 2600 |
![]()
Sci
|
- | 3.1 | 2019 | 47.7 Days | CHF 1200 |
![]()
Sustainability
|
3.9 | 5.8 | 2009 | 18.8 Days | CHF 2400 |
MDPI Topics is cooperating with Preprints.org and has built a direct connection between MDPI journals and Preprints.org. Authors are encouraged to enjoy the benefits by posting a preprint at Preprints.org prior to publication:
- Immediately share your ideas ahead of publication and establish your research priority;
- Protect your idea from being stolen with this time-stamped preprint article;
- Enhance the exposure and impact of your research;
- Receive feedback from your peers in advance;
- Have it indexed in Web of Science (Preprint Citation Index), Google Scholar, Crossref, SHARE, PrePubMed, Scilit and Europe PMC.