Topic Menu
► Topic MenuTopic Editors
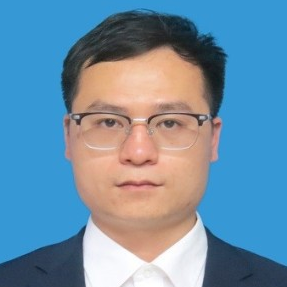




Remote Sensing and GIS for Monitoring Land Use Change and Its Ecological Effects
Topic Information
Dear Colleagues,
Land use change (LUC) is a cause and result of global changes in the environment. It provides essential food, feed, fuel, and ecosystem services for human social systems, while increasingly affecting the biogeochemical processes of the Earth, such as material exchange, energy balance, the carbon and water cycles, and climate change. In the current “Anthropocene” era, land use has undergone unprecedented changes and intensification to meet the demands of the growing population for goods and services and has had negative impacts in terms of deforestation, land degradation, habitat reduction, biodiversity loss, non-point source pollution, and greenhouse gas emissions. In order to alleviate these negative impacts and improve the efficiency and sustainability of land use, it is essential to optimize its structure, functions, and patterns. To achieve this goal, remote sensing (RS) and geographic information systems (GIS) can provide large-scale, real-time, accurate, and consistent ground information, as well as the high-performance capability to compute, analyze, and display multi-source data. These technologies have been widely used in monitoring LUC and its ecological effects; therefore, it is imperative to integrate RS and GIS to reveal the spatiotemporal processes, driving mechanisms, multi-functions, and optimization patterns of LUC. This will support the scientific basis and practical implications necessary for sustainable land use planning, environmental quality improvements, and coordinated human–earth system developments.
The aim of this Topic is to advance novel theories and methods that contribute new knowledge on various aspects of LUC. Specifically, this Topic seeks to (1) monitor the spatiotemporal patterns and processes of typical LUCs, including cropland reclamation and abandonment, crop type adjustment, rural construction and restructure, urban sprawl and compactness, and ecological land protection; (2) reveal the coupled natural and anthropogenic driving mechanisms of LUC; (3) quantify the various aspects associated with LUC, such as economic benefits, resident livelihoods, food production, agricultural non-point source pollution, ecosystem services, and ecological risks, and analyze their trade-offs and synergies; (4) assess the vulnerability, resilience, and sustainability of different land use patterns in the human–earth system; and (5) simulate and optimize LUC under different development scenarios to create adaptation strategies for future challenges. The original research articles and reviews presented in this Topic will offer scientific methodologies, systematic insights, and policy recommendations for effective land use management and regional sustainable development. By addressing these critical themes of LUC, this Topic will contribute to the advancement of knowledge and provide practical guidance for stakeholders and policymakers seeking to optimize land use for the benefits of society and the environment.
We look forward to receiving your submissions.
Dr. Yaqun Liu
Dr. Wei Song
Prof. Dr. Jieyong Wang
Dr. Kangwen Zhu
Dr. Xuanchang Zhang
Dr. Cong Ou
Topic Editors
Keywords
- land use change
- cropland reclamation and abandonment
- rural land consolidation
- urban land expansion
- ecological land protection
- agricultural non-point source pollution
- ecosystem services
- food and ecological security
- sustainable land management
- human–earth system
Participating Journals
Journal Name | Impact Factor | CiteScore | Launched Year | First Decision (median) | APC |
---|---|---|---|---|---|
![]()
Agriculture
|
3.6 | 3.6 | 2011 | 17.7 Days | CHF 2600 |
![]()
Foods
|
5.2 | 5.8 | 2012 | 13.1 Days | CHF 2900 |
![]()
Land
|
3.9 | 3.7 | 2012 | 14.8 Days | CHF 2600 |
![]()
Remote Sensing
|
5.0 | 7.9 | 2009 | 23 Days | CHF 2700 |
MDPI Topics is cooperating with Preprints.org and has built a direct connection between MDPI journals and Preprints.org. Authors are encouraged to enjoy the benefits by posting a preprint at Preprints.org prior to publication:
- Immediately share your ideas ahead of publication and establish your research priority;
- Protect your idea from being stolen with this time-stamped preprint article;
- Enhance the exposure and impact of your research;
- Receive feedback from your peers in advance;
- Have it indexed in Web of Science (Preprint Citation Index), Google Scholar, Crossref, SHARE, PrePubMed, Scilit and Europe PMC.