Journal Description
AgriEngineering
AgriEngineering
is an international, peer-reviewed, open access journal on the engineering science of agricultural and horticultural production, published monthly online by MDPI.
- Open Access— free for readers, with article processing charges (APC) paid by authors or their institutions.
- High Visibility: indexed within Scopus, ESCI (Web of Science), PubAg, FSTA, AGRIS, CAPlus / SciFinder, and other databases.
- Journal Rank: JCR - Q2 (Agricultural Engineering) / CiteScore - Q1 (Horticulture)
- Rapid Publication: manuscripts are peer-reviewed and a first decision is provided to authors approximately 21.8 days after submission; acceptance to publication is undertaken in 5 days (median values for papers published in this journal in the second half of 2024).
- Recognition of Reviewers: reviewers who provide timely, thorough peer-review reports receive vouchers entitling them to a discount on the APC of their next publication in any MDPI journal, in appreciation of the work done.
Impact Factor:
3.0 (2023);
5-Year Impact Factor:
3.1 (2023)
Latest Articles
Detection of Fusarium Head Blight in Wheat Using NDVI from Multispectral UAS Measurements and Its Correlation with DON Contamination
AgriEngineering 2025, 7(2), 37; https://doi.org/10.3390/agriengineering7020037 - 3 Feb 2025
Abstract
►
Show Figures
Fusarium head blight (FHB) is a serious fungal disease of wheat and other small cereal grains, significantly reducing grain yield and producing mycotoxins that affect food safety. There is a need for disease detection technologies to determine the right time to apply fungicides,
[...] Read more.
Fusarium head blight (FHB) is a serious fungal disease of wheat and other small cereal grains, significantly reducing grain yield and producing mycotoxins that affect food safety. There is a need for disease detection technologies to determine the right time to apply fungicides, as FHB infection begins before visible symptoms appear. Using multispectral remote sensing by an unmanned aircraft system (UAS), wheat plants were observed under field conditions infested with FHB and simultaneously protected with fungicides sprayed with four different types of nozzles, as well as corresponding control plots infested with FHB only. The results showed that the levels of deoxynivalenol (DON) differed significantly between the five treatments, indicating that the control had the highest DON concentration as no fungicide treatment was applied. This study revealed that the assessment of the normalized difference vegetation index (NDVI) after FHB infection could be useful for predicting DON accumulation in wheat, as a significant negative correlation between DON and NDVI values was measured 24 days after anthesis. The decreasing NDVI values at the end of the growth cycle were expected due to senescence and yellowing of the wheat spikes and leaves. Therefore, significant differences in the NDVI were observed between three measurement points on the 13th, 24th, and 45th day after anthesis. Additionally, the green normalized difference vegetation index (GNDVI) and normalized difference red-edge index (NDRE) were in significant positive correlation with the NDVI at 24th day after anthesis. The use of appropriate measurement points for the vegetation indices can offer the decisive advantage of enabling the evaluation of very large breeding trials or farmers’ fields where the timing of fungicide application is particularly important.
Full article
Open AccessArticle
A Novel Graph-Based Innovative Trend Analysis Technique for Studying the Crop Trends in Kerala, India
by
Chalissery Mincy Thomas and Archana Nair
AgriEngineering 2025, 7(2), 36; https://doi.org/10.3390/agriengineering7020036 - 3 Feb 2025
Abstract
This study introduces a novel graph-based innovative trend analysis (GBITA) technique for detecting trends in time series data, thus fundamentally challenging conventional assumptions in trend assessment. Unlike traditional methods, which often rely on the assumption of a serial correlation, the proposed methodology only
[...] Read more.
This study introduces a novel graph-based innovative trend analysis (GBITA) technique for detecting trends in time series data, thus fundamentally challenging conventional assumptions in trend assessment. Unlike traditional methods, which often rely on the assumption of a serial correlation, the proposed methodology only requires that the data values conform to a non-negative distribution. The effectiveness of GBITA was validated through 200 Monte Carlo simulations, and it was subsequently applied to analyze the productivity and cultivated area of paddy and coconut crops across Kerala and its 14 districts. The results indicate an upward trend in the productivity of both crops; however, the area under paddy cultivation is decreasing, while the coconut cultivation area is increasing. Notably, the southern districts of Kerala exhibited a declining trend in both the productivity and cultivated area for these crops. This innovative approach holds promise for broader applications across various crop varieties and regions, with potential implications for fields such as statistics, economics, and computer science.
Full article
(This article belongs to the Special Issue Recent Trends and Advances in Agricultural Engineering)
►▼
Show Figures
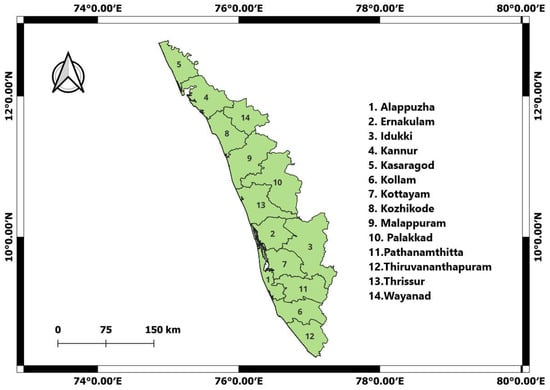
Figure 1
Open AccessArticle
Precision Monitoring of Dead Chickens and Floor Eggs with a Robotic Machine Vision Method
by
Xiao Yang, Jinchang Zhang, Bidur Paneru, Jiakai Lin, Ramesh Bahadur Bist, Guoyu Lu and Lilong Chai
AgriEngineering 2025, 7(2), 35; https://doi.org/10.3390/agriengineering7020035 - 3 Feb 2025
Abstract
Modern poultry and egg production is facing challenges such as dead chickens and floor eggs in cage-free housing. Precision poultry management strategies are needed to address those challenges. In this study, convolutional neural network (CNN) models and an intelligent bionic quadruped robot were
[...] Read more.
Modern poultry and egg production is facing challenges such as dead chickens and floor eggs in cage-free housing. Precision poultry management strategies are needed to address those challenges. In this study, convolutional neural network (CNN) models and an intelligent bionic quadruped robot were used to detect floor eggs and dead chickens in cage-free housing environments. A dataset comprising 1200 images was used to develop detection models, which were split into training, testing, and validation sets in a 3:1:1 ratio. Five different CNN models were developed based on YOLOv8 and the robot’s 360° panoramic depth perception camera. The final results indicated that YOLOv8m exhibited the highest performance, achieving a precision of 90.59%. The application of the optimal model facilitated the detection of floor eggs in dimly lit areas such as below the feeder area and in corner spaces, as well as the detection of dead chickens within the flock. This research underscores the utility of bionic robotics and convolutional neural networks for poultry management and precision livestock farming.
Full article
(This article belongs to the Section Livestock Farming Technology)
►▼
Show Figures
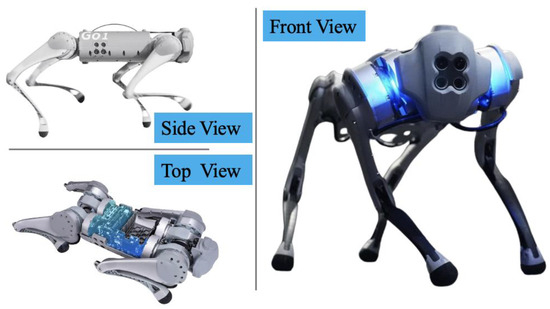
Figure 1
Open AccessArticle
An Integrated Statistical, Geostatistical and Hydrogeological Approach for Assessing and Modelling Groundwater Salinity and Quality in Nile Delta Aquifer
by
Sameh Shaddad, Annamaria Castrignanò, Diego Di Curzio, Sergio Rusi, Hend S. Abu Salem and Ahmed M. Nosair
AgriEngineering 2025, 7(2), 34; https://doi.org/10.3390/agriengineering7020034 - 31 Jan 2025
Abstract
The phenomenon of seawater intrusion is becoming increasingly problematic, particularly in low-lying coastal regions and areas that rely heavily on aquifers for their freshwater supply. It is, therefore, vital to address the causes and consequences of this phenomenon in order to ensure the
[...] Read more.
The phenomenon of seawater intrusion is becoming increasingly problematic, particularly in low-lying coastal regions and areas that rely heavily on aquifers for their freshwater supply. It is, therefore, vital to address the causes and consequences of this phenomenon in order to ensure the security of water resources and the sustainable use of water. The objective of this paper was twofold: firstly, to delineate zones with different salinization levels over time; secondly, to investigate the factors controlling seawater intrusion of the Nile Delta aquifer. Aquifer data were collected in Sharkia governorate, Egypt, over three historical periods of years: 1996, 2007, and 2018. The dataset used to create the linear model of coregionalization consisted of hydrogeological (water level), hydrodynamic (pH, EC, Na, Mg, K, Ca, HCO3, SO4), and auxiliary (distances from salt and freshwater sources) variables. Cokriging was applied to produce spatial thematic maps of the studied variables for the three years of the survey. In addition, factorial cokriging was applied to understand the processes beyond the change in the aquifer water quality and map the zones with similar characteristics. Results of mapping the first factor at long range over the three years indicated that there was an increase in seawater intrusion, especially in the northeastern part of the study area. The main cause of aquifer salinization over time was the depletion of the groundwater resource due to overexploitation.
Full article
Open AccessArticle
The Application of Machine Learning to Model the Impacts of Extreme Climatic Events on the Productivity of Dwarf Green Coconut Trees in the Eastern Amazon
by
Maryelle Kleyce M. Nery, Gabriel S. T. Fernandes, João V. de N. Pinto, Matheus L. Rua, Miguel Gabriel M. Santos, Luis Roberto T. Ribeiro, Leandro M. Navarro, Paulo Jorge O. P. de Souza and Glauco de S. Rolim
AgriEngineering 2025, 7(2), 33; https://doi.org/10.3390/agriengineering7020033 - 30 Jan 2025
Abstract
The coconut crop (Cocos nucifera L.) is essential in humid tropical regions, contributing to the economy and food security. However, its perennial nature makes it sensitive to climate variability, particularly extreme events that affect productivity. This study evaluated the impacts of extreme
[...] Read more.
The coconut crop (Cocos nucifera L.) is essential in humid tropical regions, contributing to the economy and food security. However, its perennial nature makes it sensitive to climate variability, particularly extreme events that affect productivity. This study evaluated the impacts of extreme climatic events on the productivity of dwarf green coconut in northeastern Pará, analyzing rainy (PC—December to July) and less rainy (PMC—August to November) periods between 2015 and 2023. Meteorological and experimental data were used, including extreme climate variables such as maximum temperature (HT) and precipitation (HEP), defined by the 90th percentiles, and low precipitation (LP, 10th percentile). Predictive models, such as Multiple Linear Regression (MLR) and Random Forest (RF), were developed. RF showed better performance, with an RMSE equivalent to 20% of the average productivity, while that of MLR exceeded 50%. However, RF struggled with generalization in the test set, likely due to overfitting. The inclusion of lagged productivity (productivity t-1) highlighted its significant influence. During the PC, extreme high precipitation (HEP) events and excessive water surplus (HE) occurring after the fifth month of inflorescence development contributed to increased productivity, whereas during the PMC, low-precipitation (LP) events led to productivity reductions. Notably, under certain circumstances, elevated precipitation can mitigate the negative impacts of low water availability. These findings underscore the need for adaptive management strategies to mitigate climatic impacts and promote stability in dwarf green coconut production.
Full article
(This article belongs to the Special Issue The Future of Artificial Intelligence in Agriculture)
►▼
Show Figures
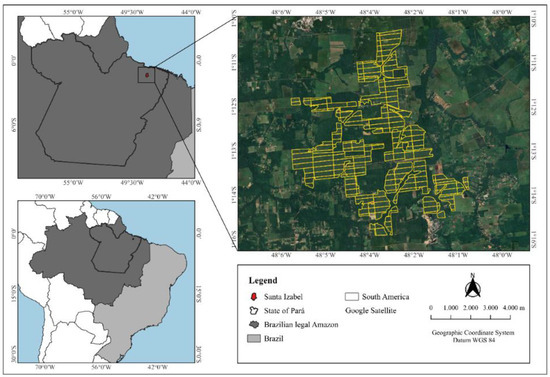
Figure 1
Open AccessArticle
Heatmap Regression-Based Context-Aware Learning for Tillage Boundary Detection
by
Gyu-Sung Ham and Kanghan Oh
AgriEngineering 2025, 7(2), 32; https://doi.org/10.3390/agriengineering7020032 - 30 Jan 2025
Abstract
In agricultural automation, autonomous tractors play a crucial role in enhancing farming efficiency, particularly through the navigation and identification of tillage boundaries. Traditional approaches rely on machine vision techniques to identify paths by distinguishing between tilled and untilled soil areas. Although recent advancements
[...] Read more.
In agricultural automation, autonomous tractors play a crucial role in enhancing farming efficiency, particularly through the navigation and identification of tillage boundaries. Traditional approaches rely on machine vision techniques to identify paths by distinguishing between tilled and untilled soil areas. Although recent advancements in convolutional neural networks (CNNs) have shown promising results in agricultural automation, they still face challenges in fully capturing the global context of tillage boundaries. This limitation stems mainly from CNNs’ small receptive fields, which often limit the network’s capacity to capture broader contextual information in agricultural landscapes, potentially causing inaccuracies in boundary detection. These methods rely significantly on local feature analysis and necessitate complex, computationally intensive heuristic post-processing to enhance detected tillage lines, thus limiting their real-time application efficacy. We propose a line-context-aware learning method that combines a heatmap regression with a transformer to more effectively learn and extract global contextual features. The proposed end-to-end method streamlines detection, enhancing real-time agricultural applications and improving the accuracy and reliability of autonomous tractor navigation and operation. The proposed method was evaluated on a custom dataset, demonstrating competitive performance in accurately detecting tillage boundaries and proving its capability to handle the intricate details and variations present in agricultural landscapes.
Full article
(This article belongs to the Section Computer Applications and Artificial Intelligence in Agriculture)
Open AccessArticle
An Intelligent Group Learning Framework for Detecting Common Tomato Diseases Using Simple and Weighted Majority Voting with Deep Learning Models
by
Seyed Mohamad Javidan, Yiannis Ampatzidis, Ahmad Banakar, Keyvan Asefpour Vakilian and Kamran Rahnama
AgriEngineering 2025, 7(2), 31; https://doi.org/10.3390/agriengineering7020031 - 28 Jan 2025
Abstract
Plant diseases pose significant economic challenges and may lead to ecological consequences. Although plant pathologists have a significant ability to diagnose plant diseases, rapid, accurate, and early diagnosis of plant diseases by intelligent systems could improve disease control and management. This study evaluates
[...] Read more.
Plant diseases pose significant economic challenges and may lead to ecological consequences. Although plant pathologists have a significant ability to diagnose plant diseases, rapid, accurate, and early diagnosis of plant diseases by intelligent systems could improve disease control and management. This study evaluates six efficient classification models (classifiers) based on deep learning to detect common tomato diseases by analyzing symptomatic patterns on leaves. Additionally, group learning techniques, including simple and weighted majority voting methods, were employed to enhance classification performance further. Six tomato leaf diseases, including Pseudomonas syringae pv. syringae bacterial spot, Phytophthora infestance late blight, Cladosporium fulvum leaf mold, Septoria lycopersici Septoria leaf spot, Corynespora cassiicola target spot, and Alternaria solani early blight, as well as healthy leaves, resulting in a total of seven classes, were utilized for the classification. Deep learning models, such as convolutional neural networks (CNNs), GoogleNet, ResNet-50, AlexNet, Inception v3, and MobileNet, were utilized, achieving classification accuracies of 65.8%, 84.9%, 93.4%, 89.4%, 93.4%, and 96%, respectively. Furthermore, applying the group learning approaches significantly improved the results, with simple majority voting achieving a classification accuracy of 99.5% and weighted majority voting achieving 100%. These findings highlight the effectiveness of the proposed deep ensemble learning models in accurately identifying and classifying tomato diseases, featuring their potential for practical applications in tomato disease diagnosis and management.
Full article
Open AccessArticle
Tracking Long-Distance Systematic Trajectories of Different Robot Mower Patterns with Enhanced Custom-Built Software
by
Sofia Matilde Luglio, Christian Frasconi, Lorenzo Gagliardi, Michele Raffaelli, Andrea Peruzzi, Stefano Pieri, Marco Volterrani, Simone Magni and Marco Fontanelli
AgriEngineering 2025, 7(2), 30; https://doi.org/10.3390/agriengineering7020030 - 28 Jan 2025
Abstract
►▼
Show Figures
Sustainable turfgrass management is essential for maintaining healthy and visually appealing green spaces. Autonomous mowers have emerged as an innovative solution, but the efficiency and quality of mowing operations depend on several factors. This study investigates the impact of mowing patterns and cutting
[...] Read more.
Sustainable turfgrass management is essential for maintaining healthy and visually appealing green spaces. Autonomous mowers have emerged as an innovative solution, but the efficiency and quality of mowing operations depend on several factors. This study investigates the impact of mowing patterns and cutting heights on the performance of an autonomous mower through updated custom-built software. Three different mowing patterns (vertical, diagonal, and horizontal) and two cutting heights (3 cm and 6 cm) were analyzed to analyze mowing efficiency, coverage, and cutting uniformity. The vertical pattern emerged as the most effective, maximizing speed (0.52 m/s) and efficiency (0.77), while minimizing overlap (4.27 cm) and uncut areas (0.014 m2). In contrast, the horizontal and diagonal patterns showed lower efficiency (0.71 and 0.76) and less coverage percentage (97.05% and 96.71%) compared to the vertical pattern (98.57%). Cutting height influenced performance, with higher heights sometimes requiring adjustments to prevent inefficiencies. The interaction between the mowing pattern and cutting height was critical for optimizing both operational efficiency and cutting quality. These findings highlight the importance of selecting an appropriate mowing pattern and cutting height tailored to the specific operational goals.
Full article
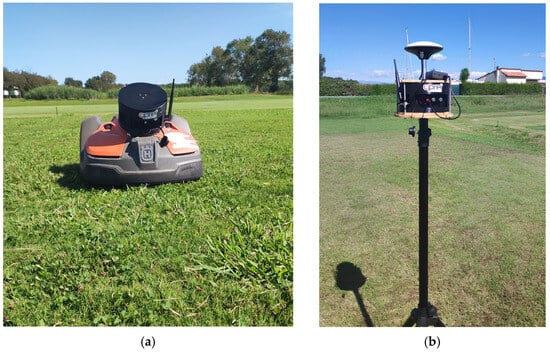
Figure 1
Open AccessArticle
AI-Driven Insect Detection, Real-Time Monitoring, and Population Forecasting in Greenhouses
by
Dimitrios Kapetas, Panagiotis Christakakis, Sofia Faliagka, Nikolaos Katsoulas and Eleftheria Maria Pechlivani
AgriEngineering 2025, 7(2), 29; https://doi.org/10.3390/agriengineering7020029 - 27 Jan 2025
Abstract
Insecticide use in agriculture has significantly increased over the past decades, reaching 774 thousand metric tons in 2022. This widespread reliance on chemical insecticides has substantial economic, environmental, and human health consequences, highlighting the urgent need for sustainable pest management strategies. Early detection,
[...] Read more.
Insecticide use in agriculture has significantly increased over the past decades, reaching 774 thousand metric tons in 2022. This widespread reliance on chemical insecticides has substantial economic, environmental, and human health consequences, highlighting the urgent need for sustainable pest management strategies. Early detection, insect monitoring, and population forecasting through Artificial Intelligence (AI)-based methods, can enable swift responsiveness, allowing for reduced but more effective insecticide use, mitigating traditional labor-intensive and error prone solutions. The main challenge is creating AI models that perform with speed and accuracy, enabling immediate farmer action. This study highlights the innovating potential of such an approach, focusing on the detection and prediction of black aphids under state-of-the-art Deep Learning (DL) models. A dataset of 220 sticky paper images was captured. The detection system employs a YOLOv10 DL model that achieved an accuracy of 89.1% (mAP50). For insect population prediction, random forests, gradient boosting, LSTM, and the ARIMA, ARIMAX, and SARIMAX models were evaluated. The ARIMAX model performed best with a Mean Square Error (MSE) of 75.61, corresponding to an average deviation of 8.61 insects per day between predicted and actual insect counts. For the visualization of the detection results, the DL model was embedded to a mobile application. This holistic approach supports early intervention strategies and sustainable pest management while offering a scalable solution for smart-agriculture environments.
Full article
(This article belongs to the Section Computer Applications and Artificial Intelligence in Agriculture)
►▼
Show Figures
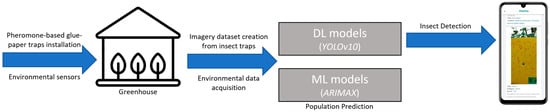
Figure 1
Open AccessArticle
Analysis of Olive Detachment Force to Improve Olive Shaker Efficiency Through Branch Modeling
by
Giuseppe Macoretta, Sofia Matilde Luglio, Federico Conforti, Michele Abruzzo, Lorenzo Gagliardi, Marco Fontanelli and Michele Raffaelli
AgriEngineering 2025, 7(2), 28; https://doi.org/10.3390/agriengineering7020028 - 27 Jan 2025
Abstract
Mechanical shaking enables efficient harvesting of olives, especially in hilly regions where automated farming is not feasible. This study delves into branch and olive detachment modeling to enhance the efficiency of a hand-held branch shaker. Shaking time, forces, accelerations, olive detachment forces and
[...] Read more.
Mechanical shaking enables efficient harvesting of olives, especially in hilly regions where automated farming is not feasible. This study delves into branch and olive detachment modeling to enhance the efficiency of a hand-held branch shaker. Shaking time, forces, accelerations, olive detachment forces and harvesting efficiency were experimentally measured. The fruit maturity index affected the force needed to detach the olive, with the highest value for olives at the C0 stage of maturity (5.93 N). No difference emerged among the tested shaking times (6 s and 12 s), neither in terms of harvest efficiency (mean 81.17%) nor in terms of damage (rate of 5.30). Therefore, the lower time was considered the most appropriate. Multibody and a Finite Element (FE) models were developed to investigate the branch response and the olive detachment condition. The stresses predicted by the FE harmonic analysis (about 8 MPa), based on the excitation force and shaking frequency measured during the tests, was in line with the measured olive detachment forces (3 to 8 MPa). The shaking frequency and the average branch acceleration in proximity to the shaker hook were 15 Hz and 50m/s2, respectively. Further studies could focus on the impact of the branch shaker on operator health, particularly risks from prolonged vibration exposure.
Full article
Open AccessArticle
Soil Structure Analysis with Attention: A Deep Deep-Learning-Based Method for 3D Pore Segmentation and Characterization
by
Italo Francyles Santos da Silva, Alan de Carvalho Araújo, João Dallyson Sousa de Almeida, Anselmo Cardoso de Paiva, Aristófanes Corrêa Silva and Deane Roehl
AgriEngineering 2025, 7(2), 27; https://doi.org/10.3390/agriengineering7020027 - 27 Jan 2025
Abstract
The pore structure plays a crucial role in soil systems. It affects a range of processes essential for soil ecological functions, such as the transport and retention of water and nutrients, as well as gas exchanges. The mechanical and hydrological characteristics of soil
[...] Read more.
The pore structure plays a crucial role in soil systems. It affects a range of processes essential for soil ecological functions, such as the transport and retention of water and nutrients, as well as gas exchanges. The mechanical and hydrological characteristics of soil are predominantly determined by the three-dimensional pore pore-space structure. A precise analysis of pore structure can help specialists understand how these shapes impact plant root activity, leading to better cultivation practices. X-ray computed tomography provides detailed information without destroying the sample. However, manually delineating pore structure and estimating porosity are challenging tasks. This work proposes an automated method for 3D pore segmentation and characterization using convolutional neural networks with attention mechanisms. The method introduces a novel approach that combines attention at both channel and spatial levels, enhancing the segmentation and property estimation, providing valuable insights for a more detailed study of soil conditions. In experiments conducted with a private dataset, the segmentation results achieved mean Dice values of 99.10% ± 0.0004 and mean IoU values of 98.23% ± 0.0008. Additionally, in tests with Phaeozem Albic, the automatic method provided porosity estimates comparable to those obtained by a method based on integral geometry and morphology.
Full article
Open AccessArticle
Treatment of Ferruginous Water in the Performance of Drip Irrigation Systems
by
Elio de Almeida Cordeiro, Everardo Chartuni Mantovani, Gustavo Haddad Souza Vieira, José Geraldo Ferreira da Silva, Ismail Ramalho Haddade and Paola Alfonsa Vieira Lo Monaco
AgriEngineering 2025, 7(2), 26; https://doi.org/10.3390/agriengineering7020026 - 24 Jan 2025
Abstract
One of the most important advantages of drip irrigation is the possibility of achieving a high uniformity of water distribution. However, this uniformity can be reduced when using water with high iron content, which can cause drippers to clog. This study aimed to
[...] Read more.
One of the most important advantages of drip irrigation is the possibility of achieving a high uniformity of water distribution. However, this uniformity can be reduced when using water with high iron content, which can cause drippers to clog. This study aimed to verify the efficiency of the chlorination, aeration, decantation and filtration processes carried out to remove the iron from irrigation water and to investigate the effect of iron on water distribution uniformity. Four similar irrigation systems with five models of drippers were installed. The results showed that (i) there was a significant difference in the drippers’ behavior in relation to susceptibility to clogging when using water with a high iron content; (ii) the use of disk filters alone was not able to promote significant reductions in the iron quantity as a way to prevent the clogging of drippers; and (iii) the use of aerators followed by sedimentation tanks made it possible to achieve a considerable improvement in the water application uniformity for drippers that were more sensitive to clogging caused by the use of water with high iron content.
Full article
(This article belongs to the Section Agricultural Irrigation Systems)
Open AccessArticle
Integrated Model to Reduce the Maneuver Time of the Harvester and Infield Wagon in Sugarcane Harvest
by
Lígia Negri Corrêa, Adão Felipe dos Santos, Carlos Eduardo Angeli Furlani, Glauco de Souza Rolim, Igor Cristian de Oliveira Vieira, Breno dos Santos Silva, Frederico Luiz Siansi and Rouverson Pereira da Silva
AgriEngineering 2025, 7(2), 25; https://doi.org/10.3390/agriengineering7020025 - 24 Jan 2025
Abstract
This study aims to enhance the efficiency of mechanized sugarcane (Saccharum spp.) harvesting by optimizing the maneuvers of the auxiliary vehicle (infield wagon) operating in tandem with the harvester. The optimization focuses on increasing productivity and reducing soil compaction in high-traffic maneuver
[...] Read more.
This study aims to enhance the efficiency of mechanized sugarcane (Saccharum spp.) harvesting by optimizing the maneuvers of the auxiliary vehicle (infield wagon) operating in tandem with the harvester. The optimization focuses on increasing productivity and reducing soil compaction in high-traffic maneuver areas. A comprehensive three-year experimental study was conducted in agricultural fields at a Brazilian mill, where extensive field data and economic parameters were collected to simulate and compare maneuvering techniques on both flat and sloped terrain. To evaluate the synchronization between the harvester and the infield wagon under non-optimized scenarios, a Maneuver Simultaneity Index (MSI) was developed. The results demonstrated that the optimized “P-optimized” technique, which utilizes dedicated maneuvering spaces, significantly increased economic returns per hour worked by reducing maneuver times across various terrain types. Additionally, fuel consumption decreased with the adoption of the optimized maneuver pattern, leading to lower operational costs and reduced environmental impact. These findings highlight the economic and operational feasibility of optimizing maneuver patterns, particularly in flat areas, and provide a sustainable framework adaptable for improving efficiency and productivity in diverse mechanized agricultural operations.
Full article
(This article belongs to the Special Issue Research Progress of Agricultural Machinery Testing)
Open AccessArticle
Advanced Deep Learning Methods for Multiple Behavior Classification of Cage-Free Laying Hens
by
Sachin Subedi, Ramesh Bahadur Bist, Xiao Yang, Guoming Li and Lilong Chai
AgriEngineering 2025, 7(2), 24; https://doi.org/10.3390/agriengineering7020024 - 23 Jan 2025
Abstract
The welfare of hens in cage-free systems is closely linked to their behaviors, such as feeding, drinking, pecking, perching, bathing, preening, and foraging. To monitor these behaviors, we developed and evaluated deep learning models based on YOLO (You Only Look Once), an advanced
[...] Read more.
The welfare of hens in cage-free systems is closely linked to their behaviors, such as feeding, drinking, pecking, perching, bathing, preening, and foraging. To monitor these behaviors, we developed and evaluated deep learning models based on YOLO (You Only Look Once), an advanced object detection technology known for its high accuracy, speed, and compact size. Three YOLO-based models—YOLOv5s_BH, YOLOv5x_BH, and YOLOv7_BH—were created to track and classify the behaviors of laying hens in cage-free environments. A dataset comprising 1500 training images, 500 validation images, and 50 test images was used to train and validate the models. The models successfully detected poultry behaviors in test images with bounding boxes and objectness scores ranging from 0 to 1. Among the models, YOLOv5s_BH demonstrated superior performance, achieving a precision of 78.1%, surpassing YOLOv5x_BH and YOLOv7_BH by 1.9% and 2.2%, respectively. It also achieved a recall of 71.7%, outperforming YOLOv5x_BH and YOLOv7_BH by 1.9% and 2.8%, respectively. Additionally, YOLOv5s_BH recorded a mean average precision (mAP) of 74.6%, exceeding YOLOv5x_BH by 2.6% and YOLOv7_BH by 9%. While all models demonstrated high detection precision, their performance was influenced by factors such as stocking density, varying light conditions, and obstructions from equipment like drinking lines, perches, and feeders. This study highlights the potential for the automated monitoring of poultry behaviors in cage-free systems, offering valuable insights for producers.
Full article
(This article belongs to the Section Livestock Farming Technology)
►▼
Show Figures
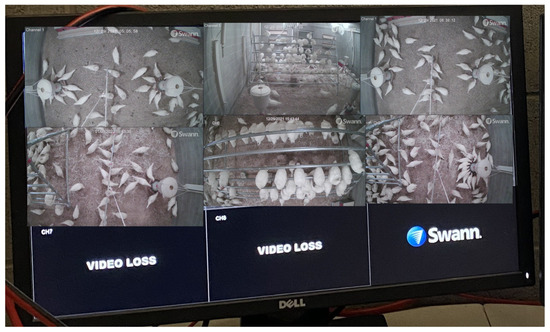
Figure 1
Open AccessArticle
Segmentation of Wheat Rust Disease Using Co-Salient Feature Extraction
by
Hirra Anwar, Haseeb Muhammad, Muhammad Mohsin Ghaffar, Muhammad Ali Afridi, Muhammad Jawad Khan, Christian Weis, Norbert Wehn and Faisal Shafait
AgriEngineering 2025, 7(2), 23; https://doi.org/10.3390/agriengineering7020023 - 23 Jan 2025
Abstract
Wheat Stripe Rust Disease (WRD) poses a significant threat to wheat crops, causing substantial yield losses and can result in total crop damage if not detected early. The localization of WRD-infected areas is a labor-intensive and time-consuming task due to the intricate and
[...] Read more.
Wheat Stripe Rust Disease (WRD) poses a significant threat to wheat crops, causing substantial yield losses and can result in total crop damage if not detected early. The localization of WRD-infected areas is a labor-intensive and time-consuming task due to the intricate and varied nature of the disease spread, especially for large plantations. Hence, segmentation of wheat crops is vital for early identification of the WRD-affected area, which allows for the implementation of targeted intervention measures. The state-of-the-art segmentation technique for WRD using the real-world semantic segmentation NWRD dataset is based on a UNet model with the Adaptive Patching with Feedback (APF) technique. However, this implementation is complex and requires significant resources and time for training due to the processing of each patch of the dataset. Our work in this paper improves the state-of-the-art by using a two-stage model: a Vision Transformer (ViT) classifier to distinguish between the rust and non-rust patches and a less complex co-salient object detection (Co-SOD) model for segmentation of the classified images. The Co-SOD model uses multiple rust patches to extract contextual features from a group of images. By analyzing multiple patches of wheat rust disease simultaneously, we can segment disease regions more accurately. Our results show that the proposed approach achieves a higher F1 score (0.638), precision (0.621), and recall (0.675) for the rust class with less training time as compared to the previous works.
Full article
(This article belongs to the Special Issue Implementation of Artificial Intelligence in Agriculture)
►▼
Show Figures
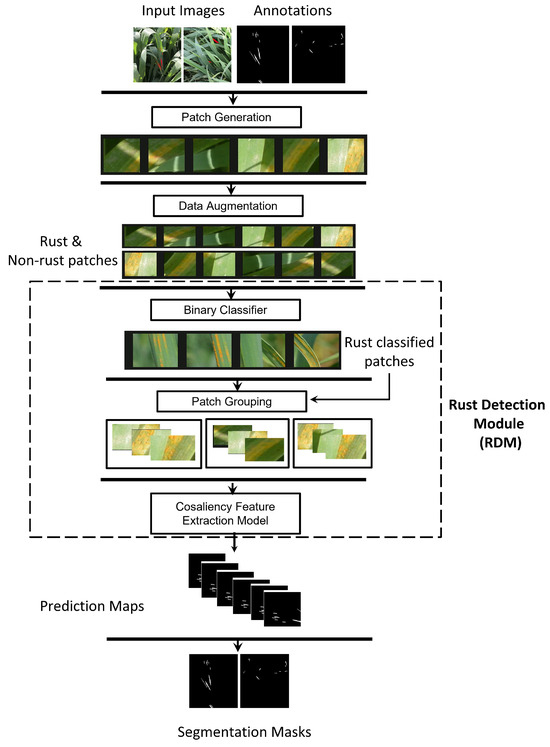
Figure 1
Open AccessArticle
Effects of Flight Heights and Nozzle Types on Spray Characteristics of Unmanned Aerial Vehicle (UAV) Sprayer in Common Field Crops
by
Saurav Ranabhat and Randy Price
AgriEngineering 2025, 7(2), 22; https://doi.org/10.3390/agriengineering7020022 - 21 Jan 2025
Abstract
►▼
Show Figures
Effective spraying is an important component of precision agriculture, directly influencing the efficiency of the spray materials. Despite their potential, optimal settings for sprayer drones remain underexplored due to limited research data. This study evaluates the effects of various flight heights and nozzle
[...] Read more.
Effective spraying is an important component of precision agriculture, directly influencing the efficiency of the spray materials. Despite their potential, optimal settings for sprayer drones remain underexplored due to limited research data. This study evaluates the effects of various flight heights and nozzle types on spray characteristics in cotton, soybean, and sugarcane crops using an unmanned aerial vehicle (UAV) sprayer. Three different flight heights and two or three nozzle types were evaluated for their impacts on spray deposition, coverage percentage, and droplet size distribution at three different canopy levels of these crops. The results indicated that lower flight heights significantly increased spray deposition and coverage in the upper canopy levels of cotton and sugarcane. Centrifugal nozzles consistently produced greater coverage and spray deposition in sugarcane. Some significant interactions among these factors were also explored. The findings highlight the potential for UAV sprayers to optimize spraying in crops with various morphologies by adjusting flight height and nozzle type.
Full article
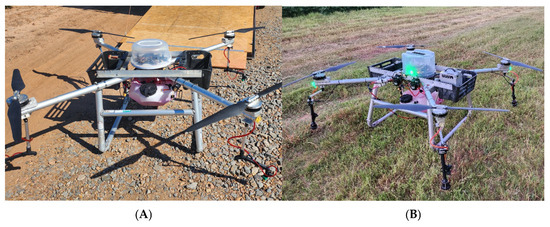
Figure 1
Open AccessArticle
Environmental Covariates for Sampling Optimization and Pest Prediction in Soybean Crops
by
Cenneya Lopes Martins, Maiara Pusch, Wesley Augusto Conde Godoy and Lucas Rios do Amaral
AgriEngineering 2025, 7(1), 21; https://doi.org/10.3390/agriengineering7010021 - 18 Jan 2025
Abstract
Insect pest infestations can vary due to spatial differences in microclimates and food availability within agroecosystems. Covariates can reflect these environmental conditions. This study tested whether using environmental covariates in two-phase sample optimization improved the spatial predictions for soybean insect pests. During the
[...] Read more.
Insect pest infestations can vary due to spatial differences in microclimates and food availability within agroecosystems. Covariates can reflect these environmental conditions. This study tested whether using environmental covariates in two-phase sample optimization improved the spatial predictions for soybean insect pests. During the 2021–2022 crop season, insect pest samples were collected at 50 georeferenced points in a commercial soybean field in Brazil, alongside data on environmental covariates such as vegetation indices, soil properties, terrain topography, and distances from riparian areas. Three covariates were selected using correlation and principal component analysis (PCA). In the 2022–2023 crop season, sample designs were optimized using the iterative algorithm optimization of sample configurations using spatial simulated annealing (SPSANN) using the selected covariates, resulting in two optimized designs that were compared to a regular grid. Data from the three sampling designs comprising 50 points were evaluated using geostatistical methods, regression analysis (pest abundance), and classification (pest presence or absence) via the random forest algorithm. The data showed no spatial dependence, making using geostatistical interpolators inappropriate. However, a multi-objective optimized sampling design, tailored to refine configurations for identifying and estimating variograms and spatial trends essential for spatial interpolation, produced the most accurate predictions. Therefore, a two-phase sample optimization with prior in situ selection of environmental covariates improves pest predictions in agricultural systems, contributing to more efficient and sustainable agricultural management.
Full article
(This article belongs to the Special Issue Research Progress and Challenges of Agricultural Information Technology)
►▼
Show Figures
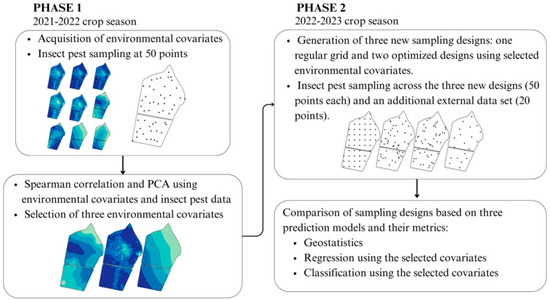
Figure 1
Open AccessArticle
Electronic Playback Devices to Reduce Ungulates’ Attendance in an Olive Grove Farm in the Province of Florence (Italy)
by
Leonardo Conti, Giulia Angeloni, Piernicola Masella, Caterina Sottili, Ferdinando Corti, Stefano Camiciottoli, Veronica Racanelli, Agnese Spadi, Francesco Garbati Pegna and Alessandro Parenti
AgriEngineering 2025, 7(1), 20; https://doi.org/10.3390/agriengineering7010020 - 17 Jan 2025
Abstract
►▼
Show Figures
(1) Background: Human–wildlife conflict can lead to adverse consequences for both parties, particularly in areas with a high concentration of wild ungulates. Ungulates cause frequent, severe plant damage by stripping the bark or browsing on the youngest plants. In the latter case, they
[...] Read more.
(1) Background: Human–wildlife conflict can lead to adverse consequences for both parties, particularly in areas with a high concentration of wild ungulates. Ungulates cause frequent, severe plant damage by stripping the bark or browsing on the youngest plants. In the latter case, they damage vegetative sprouts and leaves, which can cause a delay in growth or the plant’s death. Tuscany is notable for its significant population of wild boar, which cause substantial damage to vineyards and cereal crops, costing farmers millions annually. In Tuscany, given the highly cultivated landscape of olive trees, damage has also been recorded in these plants. Balancing human and wildlife needs is crucial for minimizing damage and ensuring coexistence. (2) Methods: This study tested innovative electronic playback devices using long-range radio technology (LoRa) to deter wild ungulates and prevent crop damage. These devices use sounds and lights to induce wild animals to be afraid and thus run away from the cultivated plot to be protected. The experiment was conducted on a farm in Chianti, Tuscany, involving four plots of land planted with olive trees: in two test areas, four playback devices and four camera traps were installed, and in the two control areas, only camera traps were installed. Playback devices aimed to deter wild ungulates and camera traps aimed to test their effectiveness. Data from the camera traps were analyzed statistically and behaviorally. (3) Results: Playback devices significantly reduced wild animal activity in the equipped areas. Statistical analysis revealed that the use of acoustic–luminous deterrent devices (PDs) significantly reduced wildlife visits to the olive groves. (4) Conclusion: The study’s findings, supported by heatmaps and frequency analyses, provide insights into wildlife activity patterns and guide the development of targeted, effective wildlife management strategies.
Full article
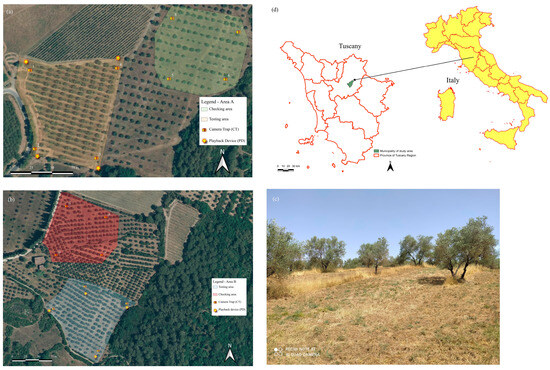
Figure 1
Open AccessArticle
Mixing Data Cube Architecture and Geo-Object-Oriented Time Series Segmentation for Mapping Heterogeneous Landscapes
by
Michel E. D. Chaves, Lívia G. D. Soares, Gustavo H. V. Barros, Ana Letícia F. Pessoa, Ronaldo O. Elias, Ana Claudia Golzio, Katyanne V. Conceição and Flávio J. O. Morais
AgriEngineering 2025, 7(1), 19; https://doi.org/10.3390/agriengineering7010019 - 17 Jan 2025
Abstract
The conflict between environmental conservation and agricultural production highlights the need for precise land use and land cover (LULC) mapping to support agro-environmental-related policies. Satellite image time series from the Moderate Resolution Image Spectroradiometer (MODIS) sensor are essential for current LULC mapping efforts.
[...] Read more.
The conflict between environmental conservation and agricultural production highlights the need for precise land use and land cover (LULC) mapping to support agro-environmental-related policies. Satellite image time series from the Moderate Resolution Image Spectroradiometer (MODIS) sensor are essential for current LULC mapping efforts. However, most approaches focus on pixel data, and studies exploring object-based spatiotemporal heterogeneity and correlation features in its time series are limited. The objective of this study is to mix the data cube architecture (analysis-ready data—ARD) and the geo-object-oriented time series segmentation via Geographic Object-Based Image Analysis (GEOBIA) to assess its performance in identifying natural vegetation and double-cropping practices over a crop season. The study area was the state of Mato Grosso, Brazil. Results indicate that, by combining GEOBIA and time series analysis (materialized by the multiresolution segmentation algorithm to derive spatiotemporal geo-objects of the MODIS data cube), representative training data collected after a quality control process, and the Support Vector Machine to classify the ARD, the overall accuracy was 0.95 and all users’ and producers’ accuracies were higher than 0.88. By considering the heterogeneity of Mato Grosso’s landscape, the results indicate the potential of the approach to provide accurate mapping.
Full article
(This article belongs to the Special Issue Research Progress and Challenges of Agricultural Information Technology)
►▼
Show Figures
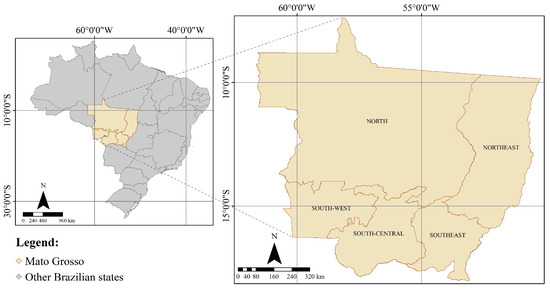
Figure 1
Open AccessArticle
Autonomous, Multisensory Soil Monitoring System
by
Valentina-Daniela Băjenaru, Simona-Elena Istrițeanu and Paul-Nicolae Ancuța
AgriEngineering 2025, 7(1), 18; https://doi.org/10.3390/agriengineering7010018 - 15 Jan 2025
Abstract
The research investigates the advantages of real-time soil quality monitoring for various land management applications. We emphasize the crucial role of soil modeling and mapping by visualizing and understanding aridity trends across different regions. The primary objective is to develop an innovative soil
[...] Read more.
The research investigates the advantages of real-time soil quality monitoring for various land management applications. We emphasize the crucial role of soil modeling and mapping by visualizing and understanding aridity trends across different regions. The primary objective is to develop an innovative soil monitoring system utilizing Internet of Things (IoT) technology. This system, equipped with intelligent sensors, will operate autonomously, collecting real-time data to identify key trends in soil conditions. Our system employs smart soil sensors to measure macronutrient values up to a depth of 80 cm. These sensors will transmit data wirelessly. Laboratory research involved a two-month evaluation of the system’s performance across three distinct soil types collected from diverse geographical locations. Analysis of the three soil types yielded a model accuracy estimate of 0.01. A strong positive linear correlation (0.92) between moisture and macronutrients has been observed in two out of the three soil types. The results, particularly related to soil moisture, were averaged over the testing period. While precipitation values were not directly integrated into the modeling framework, they were calculated in l/m2 to ensure accurate real-time estimates. The need for such advanced monitoring systems is critical for optimizing key soil macronutrients and enabling spatiotemporal mapping. This information is essential for developing effective strategies to mitigate soil aridification and prevent desertification.
Full article
(This article belongs to the Section Sensors Technology and Precision Agriculture)
►▼
Show Figures
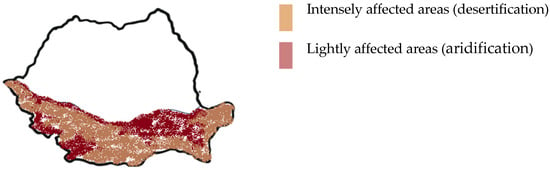
Figure 1
Highly Accessed Articles
Latest Books
E-Mail Alert
News
Topics
Topic in
Agriculture, AgriEngineering, Sustainability, Agronomy, Crops
Emerging Agricultural Engineering Sciences, Technologies, and Applications—2nd Edition
Topic Editors: Muhammad Sultan, Yuguang Zhou, Redmond R. Shamshiri, Muhammad ImranDeadline: 20 March 2025

Conferences
Special Issues
Special Issue in
AgriEngineering
Application of Geographic Information System and Remote Sensing Technology in Agricultural and Forestry Research
Guest Editors: Leonardo Conti, Gabriel Araújo e Silva Ferraz, Giuseppe RossiDeadline: 28 February 2025
Special Issue in
AgriEngineering
Precision Agriculture Meets IoT: Advanced Detection Systems for Crop Health
Guest Editors: Kyriakos Tsiakmakis, Argyrios Hatzopoulos, Stefanos StefanouDeadline: 28 February 2025
Special Issue in
AgriEngineering
Prospects of Microbial Engineering Technology in Agriculture
Guest Editors: Kaile Zhang, Laibin HuangDeadline: 31 March 2025
Special Issue in
AgriEngineering
Advancing Smart Farming through Agricultural Robots and Automation Technologies
Guest Editor: Simone PascuzziDeadline: 31 March 2025