Advances in Data, Models, and Their Applications in Agriculture
A special issue of Agronomy (ISSN 2073-4395). This special issue belongs to the section "Precision and Digital Agriculture".
Deadline for manuscript submissions: 31 October 2024 | Viewed by 9768
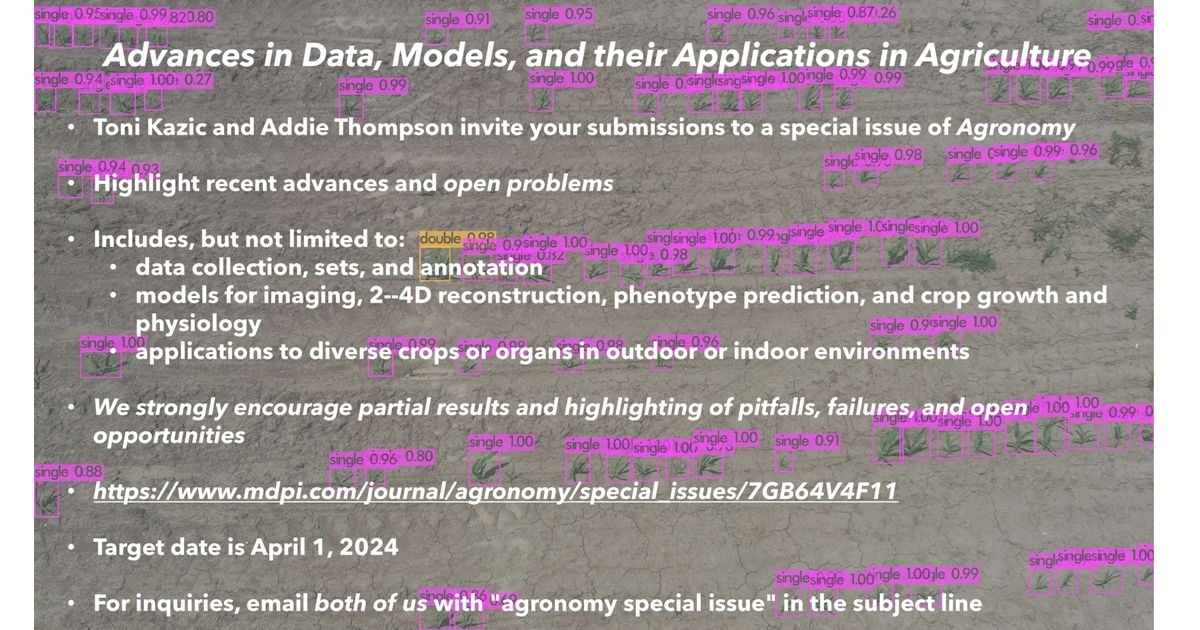
Special Issue Editors
Interests: complex phenotypes; maize lesions; computer vision; field phenomics
Special Issue Information
Dear Colleagues,
We invite you to submit your research to this Special Issue of Agronomy. The aim of this Special Issue is to highlight recent advances and open problems in agriculture, agronomy, and related fields. Areas of interest include, but are not limited to, the following:
- data—collection methods, datasets, and their validation and annotation, particularly at higher throughput and better resolution;
- models—computational, mathematical, and statistical models for imaging, morphological and developmental reconstruction, phenotypic prediction, and crop growth and physiology;
- applications to current and novel crops, especially for underserved crops, growing venues, and crop organs.
The state of the art in all of these areas is imperfect; thus, we strongly encourage the contribution of partial results and the highlighting of pitfalls, failures, and open opportunities.
Inquiries are welcome: please email both editors and include "Agronomy Special Issue" in the subject line.
Dr. Toni Kazic
Dr. Addie M. Thompson
Guest Editors
Manuscript Submission Information
Manuscripts should be submitted online at www.mdpi.com by registering and logging in to this website. Once you are registered, click here to go to the submission form. Manuscripts can be submitted until the deadline. All submissions that pass pre-check are peer-reviewed. Accepted papers will be published continuously in the journal (as soon as accepted) and will be listed together on the special issue website. Research articles, review articles as well as short communications are invited. For planned papers, a title and short abstract (about 100 words) can be sent to the Editorial Office for announcement on this website.
Submitted manuscripts should not have been published previously, nor be under consideration for publication elsewhere (except conference proceedings papers). All manuscripts are thoroughly refereed through a single-blind peer-review process. A guide for authors and other relevant information for submission of manuscripts is available on the Instructions for Authors page. Agronomy is an international peer-reviewed open access monthly journal published by MDPI.
Please visit the Instructions for Authors page before submitting a manuscript. The Article Processing Charge (APC) for publication in this open access journal is 2600 CHF (Swiss Francs). Submitted papers should be well formatted and use good English. Authors may use MDPI's English editing service prior to publication or during author revisions.
Keywords
- field phenomics
- crop modelling
- phenotypic prediction
- high throughput phenotyping
- data collection and validation
- annotation
Benefits of Publishing in a Special Issue
- Ease of navigation: Grouping papers by topic helps scholars navigate broad scope journals more efficiently.
- Greater discoverability: Special Issues support the reach and impact of scientific research. Articles in Special Issues are more discoverable and cited more frequently.
- Expansion of research network: Special Issues facilitate connections among authors, fostering scientific collaborations.
- External promotion: Articles in Special Issues are often promoted through the journal's social media, increasing their visibility.
- e-Book format: Special Issues with more than 10 articles can be published as dedicated e-books, ensuring wide and rapid dissemination.
Further information on MDPI's Special Issue polices can be found here.