New Trends in Image Processing III
A special issue of Applied Sciences (ISSN 2076-3417). This special issue belongs to the section "Computing and Artificial Intelligence".
Deadline for manuscript submissions: closed (20 August 2023) | Viewed by 25349
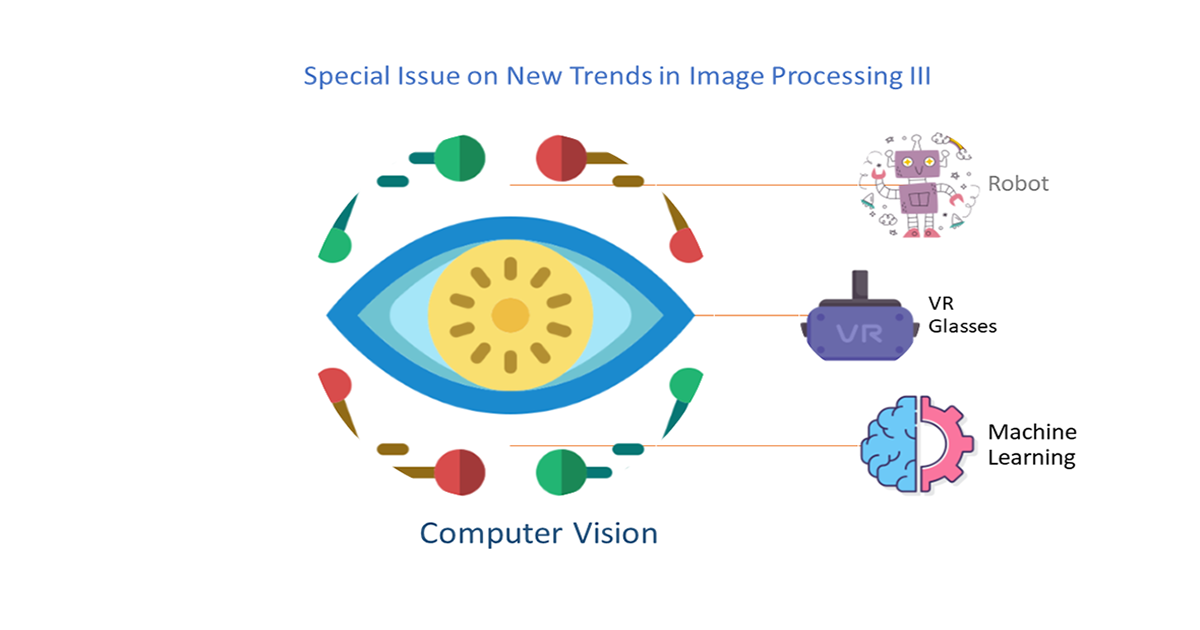
Special Issue Editors
Interests: big data; computer vision; pattern recognition; biometrics; deep learning
Special Issues, Collections and Topics in MDPI journals
Interests: visual surveillance; image and video processing; computer vision; artificial intelligence; deep learning; medical image processing; visual attention modeling; information summarization and retrieval
Special Issues, Collections and Topics in MDPI journals
Special Issue Information
Dear Colleagues,
In the last few years, the methodologies and technologies related to video processing, imaging processing, computer graphics, 3D modelling, and multimedia have seen significant implementation in the field of computer vision. The continuous development of these technologies has led to an introduction of new methodologies and applications in this field. Moreover, recent image-processing machine learning algorithms, especially deep learning, provide an opportunity to efficiently process massive datasets in order to extract information and develop new analysis procedures.
The aim of this Special Issue is two-fold: firstly, this Issue shows novel applications of modern devices for data acquisition and visualization (e.g., CCTV videos, 3D scanners, VR glasses, robots), and, secondly, it proposes new methodologies for huge dataset processing using modern pattern recognition and machine learning approaches (e.g., deep learning and hypergraph learning).
The proposed Special Issue, named “New Trends in Image Processing III”, includes (but is not limited to) the following topics:
- 3D models processing;
- Augmented and virtual reality applications;
- Robotic applications;
- RGBD analysis;
- Advanced image enhancement;
- De-noising and low-light enhancement;
- Advanced image classification and retrieval;
- Semantic segmentation;
- Image processing.
Prof. Dr. Hyeonjoon Moon
Dr. Irfan Mehmood
Guest Editors
Manuscript Submission Information
Manuscripts should be submitted online at www.mdpi.com by registering and logging in to this website. Once you are registered, click here to go to the submission form. Manuscripts can be submitted until the deadline. All submissions that pass pre-check are peer-reviewed. Accepted papers will be published continuously in the journal (as soon as accepted) and will be listed together on the special issue website. Research articles, review articles as well as short communications are invited. For planned papers, a title and short abstract (about 100 words) can be sent to the Editorial Office for announcement on this website.
Submitted manuscripts should not have been published previously, nor be under consideration for publication elsewhere (except conference proceedings papers). All manuscripts are thoroughly refereed through a single-blind peer-review process. A guide for authors and other relevant information for submission of manuscripts is available on the Instructions for Authors page. Applied Sciences is an international peer-reviewed open access semimonthly journal published by MDPI.
Please visit the Instructions for Authors page before submitting a manuscript. The Article Processing Charge (APC) for publication in this open access journal is 2400 CHF (Swiss Francs). Submitted papers should be well formatted and use good English. Authors may use MDPI's English editing service prior to publication or during author revisions.
Benefits of Publishing in a Special Issue
- Ease of navigation: Grouping papers by topic helps scholars navigate broad scope journals more efficiently.
- Greater discoverability: Special Issues support the reach and impact of scientific research. Articles in Special Issues are more discoverable and cited more frequently.
- Expansion of research network: Special Issues facilitate connections among authors, fostering scientific collaborations.
- External promotion: Articles in Special Issues are often promoted through the journal's social media, increasing their visibility.
- e-Book format: Special Issues with more than 10 articles can be published as dedicated e-books, ensuring wide and rapid dissemination.
Further information on MDPI's Special Issue polices can be found here.