Edge-Enabled Big Data Intelligence for B5G and IoT Applications
A special issue of Applied Sciences (ISSN 2076-3417). This special issue belongs to the section "Computing and Artificial Intelligence".
Deadline for manuscript submissions: closed (15 November 2023) | Viewed by 7951
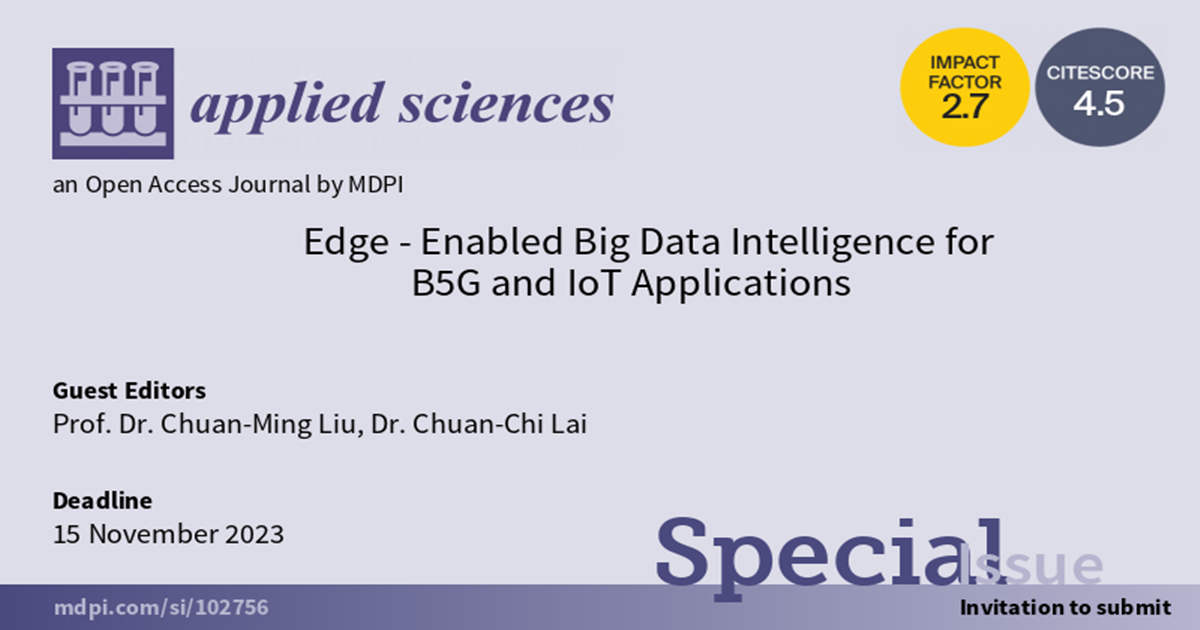
Special Issue Editors
Interests: big data management and processing; uncertain data management; data science; data management over edge computing; spatial data processing; data streams; ad hoc and sensor networks; location-based services
Special Issues, Collections and Topics in MDPI journals
Interests: UAV networks; edge intelligence; IoT data management
Special Issues, Collections and Topics in MDPI journals
Special Issue Information
Dear Colleagues,
The Internet of Things (IoT) plays a key role in the realization of smart cities, healthcare, and Industry 4.0. Massive implementations of the IoT continue to accumulate massive amounts of data. Big data processing and analysis technologies are playing an increasingly important role, making the world simpler, better, and smarter. The intelligence extracted from data processing and analysis especially provides unprecedented opportunities for a new wave of emerging applications. Meanwhile, it is well-known that big data processing and analysis require substantial computing and storage resources, which can be offered through cloud computing much easier than traditional IT. On the other hand, due to the needs of emerging 5G and B5G applications, there are many requirements in processing field data analysis to achieve low-latency response, such as unmanned driving and the intelligent control of manufacturing and transportation , which can be enabled through cloud/fog/edge computing. Therefore, we see the huge potential of cloud/fog/edge-based big data intelligence. This Special Issue aims to cover various aspects related to big data processing and analysis in conjunction with cloud/Fog/Edge computing for IoT, including, but not limited to, the following topics:
Prof. Dr. Chuan-Ming Liu
Dr. Chuan-Chi Lai
Guest Editors
Manuscript Submission Information
Manuscripts should be submitted online at www.mdpi.com by registering and logging in to this website. Once you are registered, click here to go to the submission form. Manuscripts can be submitted until the deadline. All submissions that pass pre-check are peer-reviewed. Accepted papers will be published continuously in the journal (as soon as accepted) and will be listed together on the special issue website. Research articles, review articles as well as short communications are invited. For planned papers, a title and short abstract (about 100 words) can be sent to the Editorial Office for announcement on this website.
Submitted manuscripts should not have been published previously, nor be under consideration for publication elsewhere (except conference proceedings papers). All manuscripts are thoroughly refereed through a single-blind peer-review process. A guide for authors and other relevant information for submission of manuscripts is available on the Instructions for Authors page. Applied Sciences is an international peer-reviewed open access semimonthly journal published by MDPI.
Please visit the Instructions for Authors page before submitting a manuscript. The Article Processing Charge (APC) for publication in this open access journal is 2400 CHF (Swiss Francs). Submitted papers should be well formatted and use good English. Authors may use MDPI's English editing service prior to publication or during author revisions.
Keywords
- cloud computing, fog computing, and edge computing in B5G and IoT
- novel theories, concepts, and paradigms of the convergence of AI, IoT, and Edge–Cloud
- Artificial Intelligence, machine learning, and data science in/for Edge–Cloud–IoT
- distributed computing architectures, algorithms, and models in B5G and IoT
- IoT data analytics models, algorithms, and applications
- edge-enabled big data intelligence in blockchain IoT
- explainable AI for IoT data processing
- big data intelligence for IoT security (authentication, access control, security models), privacy preservation, and data protection
- information integrity and fusion in IoT
- big data intelligence for IoT communications and networking
- multi-objective decision making/optimization in B5G and IoT applications
- application and case studies (healthcare, Industry 4.0, energy, smart city, finance, etc.)
Benefits of Publishing in a Special Issue
- Ease of navigation: Grouping papers by topic helps scholars navigate broad scope journals more efficiently.
- Greater discoverability: Special Issues support the reach and impact of scientific research. Articles in Special Issues are more discoverable and cited more frequently.
- Expansion of research network: Special Issues facilitate connections among authors, fostering scientific collaborations.
- External promotion: Articles in Special Issues are often promoted through the journal's social media, increasing their visibility.
- e-Book format: Special Issues with more than 10 articles can be published as dedicated e-books, ensuring wide and rapid dissemination.
Further information on MDPI's Special Issue policies can be found here.