Journal Description
BioMedInformatics
BioMedInformatics
is an international, peer-reviewed, open access journal on all areas of biomedical informatics, as well as computational biology and medicine, published quarterly online by MDPI.
- Open Access— free for readers, with article processing charges (APC) paid by authors or their institutions.
- High Visibility: indexed within Scopus, EBSCO, and other databases.
- Rapid Publication: manuscripts are peer-reviewed and a first decision is provided to authors approximately 21 days after submission; acceptance to publication is undertaken in 8.8 days (median values for papers published in this journal in the second half of 2023).
- Recognition of Reviewers: APC discount vouchers, optional signed peer review, and reviewer names published annually in the journal.
Latest Articles
Assaying and Classifying T Cell Function by Cell Morphology
BioMedInformatics 2024, 4(2), 1144-1154; https://doi.org/10.3390/biomedinformatics4020063 (registering DOI) - 26 Apr 2024
Abstract
Immune cell function varies tremendously between individuals, posing a major challenge to emerging cellular immunotherapies. This report pursues the use of cell morphology as an indicator of high-level T cell function. Short-term spreading of T cells on planar, elastic surfaces was quantified by
[...] Read more.
Immune cell function varies tremendously between individuals, posing a major challenge to emerging cellular immunotherapies. This report pursues the use of cell morphology as an indicator of high-level T cell function. Short-term spreading of T cells on planar, elastic surfaces was quantified by 11 morphological parameters and analyzed to identify effects of both intrinsic and extrinsic factors. Our findings identified morphological features that varied between T cells isolated from healthy donors and those from patients being treated for Chronic Lymphocytic Leukemia (CLL). This approach also identified differences between cell responses to substrates of different elastic modulus. Combining multiple features through a machine learning approach such as Decision Tree or Random Forest provided an effective means for identifying whether T cells came from healthy or CLL donors. Further development of this approach could lead to a rapid assay of T cell function to guide cellular immunotherapy.
Full article
(This article belongs to the Special Issue Editor's Choices Series for Methods in Biomedical Informatics Section)
►
Show Figures
Open AccessReview
Recent Advances in Large Language Models for Healthcare
by
Khalid Nassiri and Moulay A. Akhloufi
BioMedInformatics 2024, 4(2), 1097-1143; https://doi.org/10.3390/biomedinformatics4020062 - 16 Apr 2024
Abstract
Recent advances in the field of large language models (LLMs) underline their high potential for applications in a variety of sectors. Their use in healthcare, in particular, holds out promising prospects for improving medical practices. As we highlight in this paper, LLMs have
[...] Read more.
Recent advances in the field of large language models (LLMs) underline their high potential for applications in a variety of sectors. Their use in healthcare, in particular, holds out promising prospects for improving medical practices. As we highlight in this paper, LLMs have demonstrated remarkable capabilities in language understanding and generation that could indeed be put to good use in the medical field. We also present the main architectures of these models, such as GPT, Bloom, or LLaMA, composed of billions of parameters. We then examine recent trends in the medical datasets used to train these models. We classify them according to different criteria, such as size, source, or subject (patient records, scientific articles, etc.). We mention that LLMs could help improve patient care, accelerate medical research, and optimize the efficiency of healthcare systems such as assisted diagnosis. We also highlight several technical and ethical issues that need to be resolved before LLMs can be used extensively in the medical field. Consequently, we propose a discussion of the capabilities offered by new generations of linguistic models and their limitations when deployed in a domain such as healthcare.
Full article
(This article belongs to the Special Issue Feature Papers in Clinical Informatics Section)
►▼
Show Figures
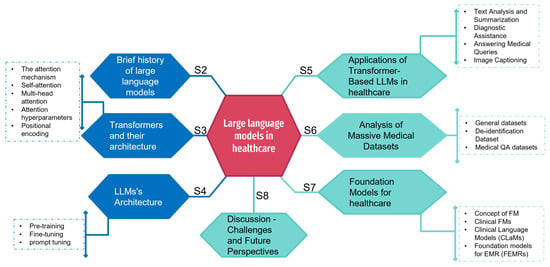
Figure 1
Open AccessProject Report
Investigating the Effectiveness of an IMU Portable Gait Analysis Device: An Application for Parkinson’s Disease Management
by
Nikos Tsotsolas, Eleni Koutsouraki, Aspasia Antonakaki, Stefanos Pizanias, Marios Kounelis, Dimitrios D. Piromalis, Dimitrios P. Kolovos, Christos Kokkotis, Themistoklis Tsatalas, George Bellis, Dimitrios Tsaopoulos, Paris Papaggelos, George Sidiropoulos and Giannis Giakas
BioMedInformatics 2024, 4(2), 1085-1096; https://doi.org/10.3390/biomedinformatics4020061 - 10 Apr 2024
Abstract
As part of two research projects, a small gait analysis device was developed for use inside and outside the home by patients themselves. The project PARMODE aims to record accurate gait measurements in patients with Parkinson’s disease (PD) and proceed with an in-depth
[...] Read more.
As part of two research projects, a small gait analysis device was developed for use inside and outside the home by patients themselves. The project PARMODE aims to record accurate gait measurements in patients with Parkinson’s disease (PD) and proceed with an in-depth analysis of the gait characteristics, while the project CPWATCHER aims to assess the quality of hand movement in cerebral palsy patients. The device was mainly developed to serve the first project with additional offline processing, including machine learning algorithms that could potentially be used for the second aim. A key feature of the device is its small size (36 mm × 46 mm × 16 mm, weight: 14 g), which was designed to meet specific requirements in terms of device consumption restrictions due to the small size of the battery and the need for autonomous operation for more than ten hours. This research work describes, on the one hand, the new device with an emphasis on its functions, and on the other hand, its connection with a web platform for reading and processing data from the devices placed on patients’ feet to record the gait characteristics of patients on a continuous basis.
Full article
(This article belongs to the Special Issue Deep Learning Methods and Application for Bioinformatics and Healthcare)
►▼
Show Figures
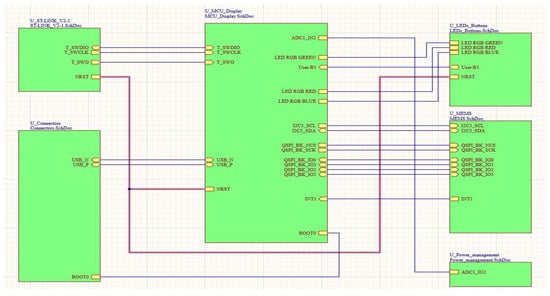
Figure 1
Open AccessArticle
Analyzing Patterns of Service Utilization Using Graph Topology to Understand the Dynamic of the Engagement of Patients with Complex Problems with Health Services
by
Jonas Bambi, Yudi Santoso, Ken Moselle, Stan Robertson, Abraham Rudnick, Ernie Chang and Alex Kuo
BioMedInformatics 2024, 4(2), 1071-1084; https://doi.org/10.3390/biomedinformatics4020060 - 09 Apr 2024
Abstract
Background: Providing care to persons with complex problems is inherently difficult due to several factors, including the impacts of proximal determinants of health, treatment response, the natural emergence of comorbidities, and service system capacity to provide timely required services. Providing visibility into the
[...] Read more.
Background: Providing care to persons with complex problems is inherently difficult due to several factors, including the impacts of proximal determinants of health, treatment response, the natural emergence of comorbidities, and service system capacity to provide timely required services. Providing visibility into the dynamics of patients’ engagement can help to optimize care for patients with complex problems. Method: In a previous work, graph machine learning and NLP methods were used to model the products of service system dynamics as atemporal entities, using a data model that collapsed patient encounter events across time. In this paper, the order of events is put back into the data model to provide topological depictions of the dynamics that are embodied in patients’ movement across a complex healthcare system. Result: The results show that directed graphs are well suited to the task of depicting the way that the diverse components of the system are functionally coupled—or remain disconnected—by patient journeys. Conclusion: By setting the resolution on the graph topology visualization, important characteristics can be highlighted, including highly prevalent repeating sequences of service events readily interpretable by clinical subject matter experts. Moreover, this methodology provides a first step in addressing the challenge of locating potential operational problems for patients with complex issues engaging with a complex healthcare service system.
Full article
(This article belongs to the Special Issue Feature Papers in Clinical Informatics Section)
►▼
Show Figures
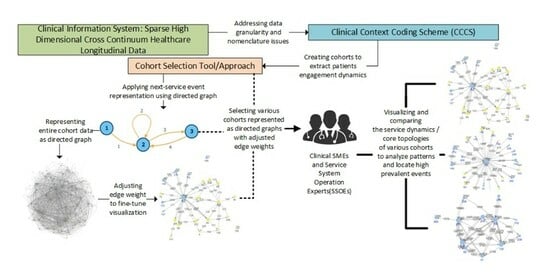
Graphical abstract
Open AccessArticle
Utilizing Generative Adversarial Networks for Acne Dataset Generation in Dermatology
by
Aravinthan Sankar, Kunal Chaturvedi, Al-Akhir Nayan, Mohammad Hesam Hesamian, Ali Braytee and Mukesh Prasad
BioMedInformatics 2024, 4(2), 1059-1070; https://doi.org/10.3390/biomedinformatics4020059 - 09 Apr 2024
Abstract
Background: In recent years, computer-aided diagnosis for skin conditions has made significant strides, primarily driven by artificial intelligence (AI) solutions. However, despite this progress, the efficiency of AI-enabled systems remains hindered by the scarcity of high-quality and large-scale datasets, primarily due to privacy
[...] Read more.
Background: In recent years, computer-aided diagnosis for skin conditions has made significant strides, primarily driven by artificial intelligence (AI) solutions. However, despite this progress, the efficiency of AI-enabled systems remains hindered by the scarcity of high-quality and large-scale datasets, primarily due to privacy concerns. Methods: This research circumvents privacy issues associated with real-world acne datasets by creating a synthetic dataset of human faces with varying acne severity levels (mild, moderate, and severe) using Generative Adversarial Networks (GANs). Further, three object detection models—YOLOv5, YOLOv8, and Detectron2—are used to evaluate the efficacy of the augmented dataset for detecting acne. Results: Integrating StyleGAN with these models, the results demonstrate the mean average precision (mAP) scores: YOLOv5: 73.5%, YOLOv8: 73.6%, and Detectron2: 37.7%. These scores surpass the mAP achieved without GANs. Conclusions: This study underscores the effectiveness of GANs in generating synthetic facial acne images and emphasizes the importance of utilizing GANs and convolutional neural network (CNN) models for accurate acne detection.
Full article
(This article belongs to the Special Issue Feature Papers in Applied Biomedical Data Science)
►▼
Show Figures
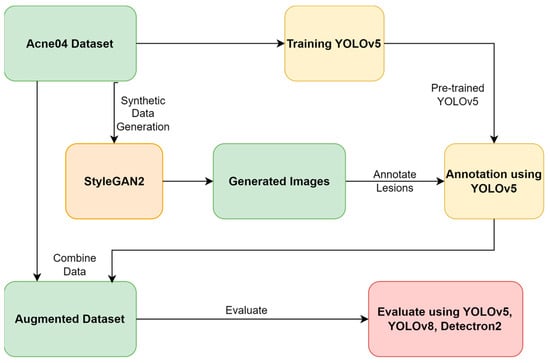
Figure 1
Open AccessArticle
A Comprehensive Analysis of Trapezius Muscle EMG Activity in Relation to Stress and Meditation
by
Mohammad Ahmed, Michael Grillo, Amirtaha Taebi, Mehmet Kaya and Peshala Thibbotuwawa Gamage
BioMedInformatics 2024, 4(2), 1047-1058; https://doi.org/10.3390/biomedinformatics4020058 - 09 Apr 2024
Abstract
Introduction: This study analyzes the efficacy of trapezius muscle electromyography (EMG) in discerning mental states, namely stress and meditation. Methods: Fifteen healthy participants were monitored to assess their physiological responses to mental stressors and meditation. Sensors were affixed to both the right and
[...] Read more.
Introduction: This study analyzes the efficacy of trapezius muscle electromyography (EMG) in discerning mental states, namely stress and meditation. Methods: Fifteen healthy participants were monitored to assess their physiological responses to mental stressors and meditation. Sensors were affixed to both the right and left trapezius muscles to capture EMG signals, while simultaneous electroencephalography (EEG) was conducted to validate cognitive states. Results: Our analysis of various EMG features, considering frequency ranges and sensor positioning, revealed significant changes in trapezius muscle activity during stress and meditation. Notably, low-frequency EMG features facilitated enhanced stress detection. For accurate stress identification, sensor configurations can be limited to the right trapezius muscle. Furthermore, the introduction of a novel method for determining asymmetry in EMG features suggests that applying sensors on bilateral trapezius muscles can improve the detection of mental states. Conclusion: This research presents a promising avenue for efficient cognitive state monitoring through compact and convenient sensing.
Full article
(This article belongs to the Special Issue Editor's Choices Series for Clinical Informatics Section)
►▼
Show Figures
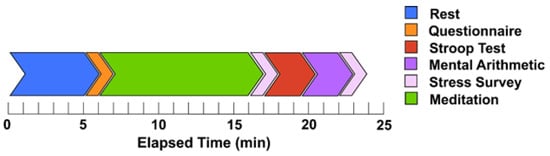
Figure 1
Open AccessArticle
Quantifying Inhaled Concentrations of Particulate Matter, Carbon Dioxide, Nitrogen Dioxide, and Nitric Oxide Using Observed Biometric Responses with Machine Learning
by
Shisir Ruwali, Shawhin Talebi, Ashen Fernando, Lakitha O. H. Wijeratne, John Waczak, Prabuddha M. H. Dewage, David J. Lary, John Sadler, Tatiana Lary, Matthew Lary and Adam Aker
BioMedInformatics 2024, 4(2), 1019-1046; https://doi.org/10.3390/biomedinformatics4020057 - 03 Apr 2024
Abstract
Introduction: Air pollution has numerous impacts on human health on a variety of time scales. Pollutants such as particulate matter—PM1 and PM2.5, carbon dioxide (CO2), nitrogen dioxide (NO2), and nitric oxide (NO) are exemplars of the
[...] Read more.
Introduction: Air pollution has numerous impacts on human health on a variety of time scales. Pollutants such as particulate matter—PM1 and PM2.5, carbon dioxide (CO2), nitrogen dioxide (NO2), and nitric oxide (NO) are exemplars of the wider human exposome. In this study, we adopted a unique approach by utilizing the responses of human autonomic systems to gauge the abundance of pollutants in inhaled air. Objective: To investigate how the human body autonomically responds to inhaled pollutants in microenvironments, including PM1, PM2.5, CO2, NO2, and NO, on small temporal and spatial scales by making use of biometric observations of the human autonomic response. To test the accuracy in predicting the concentrations of these pollutants using biological measurements of the participants. Methodology: Two experimental approaches having a similar methodology that employs a biometric suite to capture the physiological responses of cyclists were compared, and multiple sensors were used to measure the pollutants in the air surrounding them. Machine learning algorithms were used to estimate the levels of these pollutants and decipher the body’s automatic reactions to them. Results: We observed high precision in predicting PM1, PM2.5, and CO2 using a limited set of biometrics measured from the participants, as indicated with the coefficient of determination (R2) between the estimated and true values of these pollutants of 0.99, 0.96, and 0.98, respectively. Although the predictions for NO2 and NO were reliable at lower concentrations, which was observed qualitatively, the precision varied throughout the data range. Skin temperature, heart rate, and respiration rate were the common physiological responses that were the most influential in predicting the concentration of these pollutants. Conclusion: Biometric measurements can be used to estimate air quality components such as PM1, PM2.5, and CO2 with high degrees of accuracy and can also be used to decipher the effect of these pollutants on the human body using machine learning techniques. The results for NO2 and NO suggest a requirement to improve our models with more comprehensive data collection or advanced machine learning techniques to improve the results for these two pollutants.
Full article
(This article belongs to the Special Issue Feature Papers in Applied Biomedical Data Science)
►▼
Show Figures
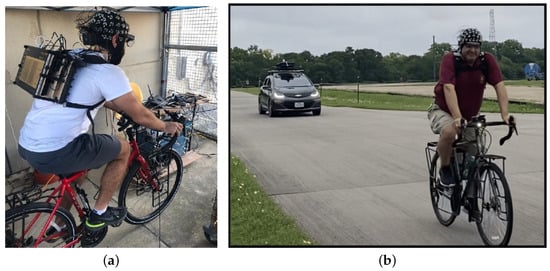
Figure 1
Open AccessArticle
Design and Modelling of an Induction Heating Coil to Investigate the Thermal Response of Magnetic Nanoparticles for Hyperthermia Applications
by
Philip Drake, Ali Algaddafi, Thomas Swift and Raed A. Abd-Alhameed
BioMedInformatics 2024, 4(2), 1006-1018; https://doi.org/10.3390/biomedinformatics4020056 - 02 Apr 2024
Abstract
Magnetic Field Hyperthermia is a technique where tumours are treated through an increase in local temperature upon exposure to alternating magnetic fields (AMFs) that are mediated by magnetic nano-particles (MNPs). In an AMF, these particles heat-up and kill the cells. The relationship between
[...] Read more.
Magnetic Field Hyperthermia is a technique where tumours are treated through an increase in local temperature upon exposure to alternating magnetic fields (AMFs) that are mediated by magnetic nano-particles (MNPs). In an AMF, these particles heat-up and kill the cells. The relationship between an AMF and the heating-rate is complex, leading to confusion when comparing data for different MNP and AMF conditions. This work allows for the thermal-response to be monitored at multiple AMF amplitudes while keeping other parameters constant. An induction-heating coil was designed based on a Zero-Voltage-Zero-Current (ZVZC) resonant circuit. The coil operates at 93 kHz with a variable DC drive-voltage (12–30 V). NEC4 software was used to model the magnetic field distribution, and MNPs were synthesised by the coprecipitation method. The magnetic field was found to be uniform at the centre of the coil and ranged from 1 kAm−1 to 12 kAm−1, depending on the DC drive-voltage. The MNPs were found to have a specific absorption rate (SAR) of 1.37 Wg−1[Fe] and 6.13 Wg−1[Fe] at 93 kHz and 2.1 kAm−1 and 12.6 kAm−1, respectively. The measured SAR value was found to be directly proportional to the product of the frequency and field-strength (SARα f Ho). This leads to the recommendation that, when comparing data from various groups, the SAR value should be normalized following this relationship and not using the more common relationship based on the square of the field intensity (SARα f Ho2).
Full article
(This article belongs to the Special Issue Feature Papers in Clinical Informatics Section)
►▼
Show Figures
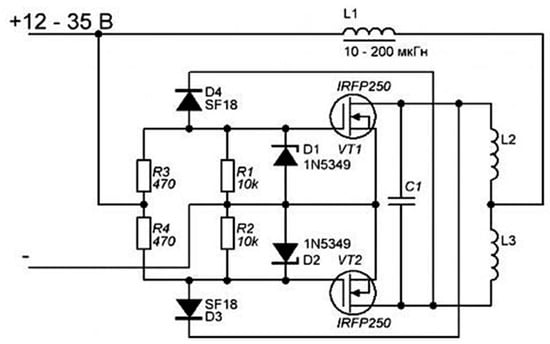
Figure 1
Open AccessArticle
Advancing Early Detection of Breast Cancer: A User-Friendly Convolutional Neural Network Automation System
by
Annie Dequit and Fatema Nafa
BioMedInformatics 2024, 4(2), 992-1005; https://doi.org/10.3390/biomedinformatics4020055 - 01 Apr 2024
Abstract
Background: Deep learning models have shown potential in improving cancer diagnosis and treatment. This study aimed to develop a convolutional neural network (CNN) model to predict Invasive Ductal Carcinoma (IDC), a common type of breast cancer. Additionally, a user-friendly interface was designed to
[...] Read more.
Background: Deep learning models have shown potential in improving cancer diagnosis and treatment. This study aimed to develop a convolutional neural network (CNN) model to predict Invasive Ductal Carcinoma (IDC), a common type of breast cancer. Additionally, a user-friendly interface was designed to facilitate the use of the model by healthcare professionals. Methods: The CNN model was trained and tested using a dataset of high-resolution microscopic images derived from 162 whole-mount slide images of breast cancer specimens. These images were meticulously scanned at 40× magnification using a state-of-the-art digital slide scanner to capture detailed information. Each image was then divided into 277,524 patches of 50 × 50 pixels, resulting in a diverse dataset containing 198,738 IDC-negative and 78,786 IDC-positive patches. Results: The model achieved an accuracy of 98.24% in distinguishing between benign and malignant cases, demonstrating its effectiveness in cancer detection. Conclusions: This study suggests that the developed CNN model has promising potential for clinical applications in breast cancer diagnosis and personalized treatment strategies. Our study further emphasizes the importance of accurate and reliable cancer detection methods for timely diagnosis and treatment. This study establishes a foundation for utilizing deep learning models in future cancer treatment research by demonstrating their effectiveness in analyzing large and complex datasets. This approach opens exciting avenues for further research and potentially improves our understanding of cancer and its treatment.
Full article
(This article belongs to the Special Issue Feature Papers in Clinical Informatics Section)
►▼
Show Figures
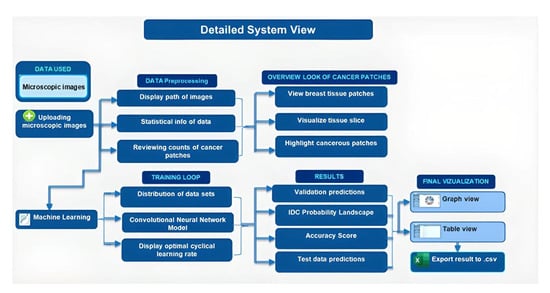
Graphical abstract
Open AccessArticle
Advancing Early Leukemia Diagnostics: A Comprehensive Study Incorporating Image Processing and Transfer Learning
by
Rezaul Haque, Abdullah Al Sakib, Md Forhad Hossain, Fahadul Islam, Ferdaus Ibne Aziz, Md Redwan Ahmed, Somasundar Kannan, Ali Rohan and Md Junayed Hasan
BioMedInformatics 2024, 4(2), 966-991; https://doi.org/10.3390/biomedinformatics4020054 - 01 Apr 2024
Abstract
Disease recognition has been revolutionized by autonomous systems in the rapidly developing field of medical technology. A crucial aspect of diagnosis involves the visual assessment and enumeration of white blood cells in microscopic peripheral blood smears. This practice yields invaluable insights into a
[...] Read more.
Disease recognition has been revolutionized by autonomous systems in the rapidly developing field of medical technology. A crucial aspect of diagnosis involves the visual assessment and enumeration of white blood cells in microscopic peripheral blood smears. This practice yields invaluable insights into a patient’s health, enabling the identification of conditions of blood malignancies such as leukemia. Early identification of leukemia subtypes is paramount for tailoring appropriate therapeutic interventions and enhancing patient survival rates. However, traditional diagnostic techniques, which depend on visual assessment, are arbitrary, laborious, and prone to errors. The advent of ML technologies offers a promising avenue for more accurate and efficient leukemia classification. In this study, we introduced a novel approach to leukemia classification by integrating advanced image processing, diverse dataset utilization, and sophisticated feature extraction techniques, coupled with the development of TL models. Focused on improving accuracy of previous studies, our approach utilized Kaggle datasets for binary and multiclass classifications. Extensive image processing involved a novel LoGMH method, complemented by diverse augmentation techniques. Feature extraction employed DCNN, with subsequent utilization of extracted features to train various ML and TL models. Rigorous evaluation using traditional metrics revealed Inception-ResNet’s superior performance, surpassing other models with F1 scores of 96.07% and 95.89% for binary and multiclass classification, respectively. Our results notably surpass previous research, particularly in cases involving a higher number of classes. These findings promise to influence clinical decision support systems, guide future research, and potentially revolutionize cancer diagnostics beyond leukemia, impacting broader medical imaging and oncology domains.
Full article
(This article belongs to the Special Issue Advances in Quantitative Imaging Analysis: From Theory to Practice)
►▼
Show Figures
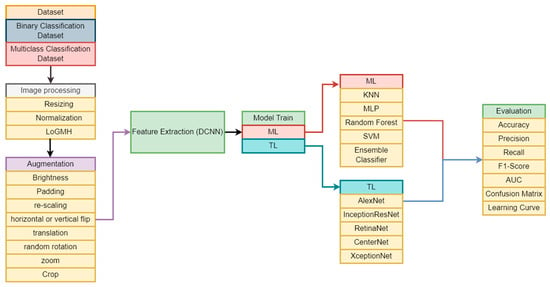
Figure 1
Open AccessArticle
A Methodological Approach to Extracting Patterns of Service Utilization from a Cross-Continuum High Dimensional Healthcare Dataset to Support Care Delivery Optimization for Patients with Complex Problems
by
Jonas Bambi, Yudi Santoso, Hanieh Sadri, Ken Moselle, Abraham Rudnick, Stan Robertson, Ernie Chang, Alex Kuo, Joseph Howie, Gracia Yunruo Dong, Kehinde Olobatuyi, Mahdi Hajiabadi and Ashlin Richardson
BioMedInformatics 2024, 4(2), 946-965; https://doi.org/10.3390/biomedinformatics4020053 - 01 Apr 2024
Cited by 1
Abstract
Background: Optimizing care for patients with complex problems entails the integration of clinically appropriate problem-specific clinical protocols, and the optimization of service-system-encompassing clinical pathways. However, alignment of service system operations with Clinical Practice Guidelines (CPGs) is far more challenging than the time-bounded alignment
[...] Read more.
Background: Optimizing care for patients with complex problems entails the integration of clinically appropriate problem-specific clinical protocols, and the optimization of service-system-encompassing clinical pathways. However, alignment of service system operations with Clinical Practice Guidelines (CPGs) is far more challenging than the time-bounded alignment of procedures with protocols. This is due to the challenge of identifying longitudinal patterns of service utilization in the cross-continuum data to assess adherence to the CPGs. Method: This paper proposes a new methodology for identifying patients’ patterns of service utilization (PSUs) within sparse high-dimensional cross-continuum health datasets using graph community detection. Result: The result has shown that by using iterative graph community detections, and graph metrics combined with input from clinical and operational subject matter experts, it is possible to extract meaningful functionally integrated PSUs. Conclusions: This introduces the possibility of influencing the reorganization of some services to provide better care for patients with complex problems. Additionally, this introduces a novel analytical framework relying on patients’ service pathways as a foundation to generate the basic entities required to evaluate conformance of interventions to cohort-specific clinical practice guidelines, which will be further explored in our future research.
Full article
(This article belongs to the Special Issue Feature Papers in Clinical Informatics Section)
►▼
Show Figures
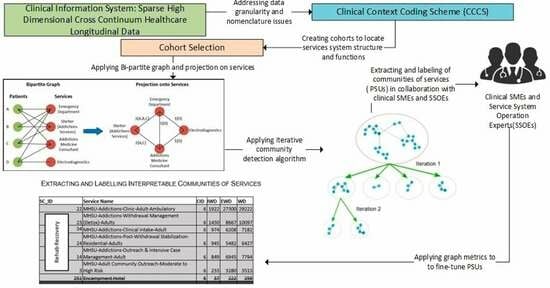
Graphical abstract
Open AccessArticle
Deep Segmentation Techniques for Breast Cancer Diagnosis
by
Storm Schutte and Jia Uddin
BioMedInformatics 2024, 4(2), 921-945; https://doi.org/10.3390/biomedinformatics4020052 - 01 Apr 2024
Abstract
Background: This research goes into in deep learning technologies within the realm of medical imaging, with a specific focus on the detection of anomalies in medical pathology, emphasizing breast cancer. It underscores the critical importance of segmentation techniques in identifying diseases and addresses
[...] Read more.
Background: This research goes into in deep learning technologies within the realm of medical imaging, with a specific focus on the detection of anomalies in medical pathology, emphasizing breast cancer. It underscores the critical importance of segmentation techniques in identifying diseases and addresses the challenges of scarce labelled data in Whole Slide Images. Additionally, the paper provides a review, cataloguing 61 deep learning architectures identified during the study. Objectives: The aim of this study is to present and assess a novel quantitative approach utilizing specific deep learning architectures, namely the Feature Pyramid Net-work and the Linknet model, both of which integrate a ResNet34 layer encoder to enhance performance. The paper also seeks to examine the efficiency of a semi-supervised training regimen using a dual model architecture, consisting of ‘Teacher’ and ‘Student’ models, in addressing the issue of limited labelled datasets. Methods: Employing a semi-supervised training methodology, this research enables the ‘Student’ model to learn from the ‘Teacher’ model’s outputs. The study methodically evaluates the models’ stability, accuracy, and segmentation capabilities, employing metrics such as the Dice Coefficient and the Jaccard Index for comprehensive assessment. Results: The investigation reveals that the Linknet model exhibits good performance, achieving an accuracy rate of 94% in the detection of breast cancer tissues utilizing a 21-seed parameter for the initialization of model weights. It further excels in generating annotations for the ‘Student’ model, which then achieves a 91% accuracy with minimal computational demands. Conversely, the Feature Pyramid Network model demonstrates a slightly lower accuracy of 93% in the Teacher model but exhibits improved and more consistent results in the ‘Student’ model, reaching 95% accuracy with a 42-seed parameter. Conclusions: This study underscores the efficacy and potential of the Feature Pyra-mid Network and Linknet models in the domain of medical image analysis, particularly in the detection of breast cancer, and suggests their broader applicability in various medical segmentation tasks related to other pathology disorders. Furthermore, the research enhances the understanding of the pivotal role that deep learning technologies play in advancing diagnostic methods within the field of medical imaging.
Full article
(This article belongs to the Special Issue Feature Papers in Computational Biology and Medicine)
►▼
Show Figures
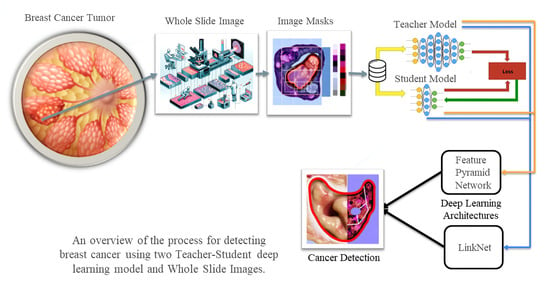
Graphical abstract
Open AccessArticle
Hearables: In-Ear Multimodal Data Fusion for Robust Heart Rate Estimation
by
Marek Żyliński, Amir Nassibi, Edoardo Occhipinti, Adil Malik, Matteo Bermond, Harry J. Davies and Danilo P. Mandic
BioMedInformatics 2024, 4(2), 911-920; https://doi.org/10.3390/biomedinformatics4020051 - 01 Apr 2024
Abstract
Background: Ambulatory heart rate (HR) monitors that acquire electrocardiogram (ECG) or/and photoplethysmographm (PPG) signals from the torso, wrists, or ears are notably less accurate in tasks associated with high levels of movement compared to clinical measurements. However, a reliable estimation of
[...] Read more.
Background: Ambulatory heart rate (HR) monitors that acquire electrocardiogram (ECG) or/and photoplethysmographm (PPG) signals from the torso, wrists, or ears are notably less accurate in tasks associated with high levels of movement compared to clinical measurements. However, a reliable estimation of HR can be obtained through data fusion from different sensors. These methods are especially suitable for multimodal hearable devices, where heart rate can be tracked from different modalities, including electrical ECG, optical PPG, and sounds (heart tones). Combined information from different modalities can compensate for single source limitations. Methods: In this paper, we evaluate the possible application of data fusion methods in hearables. We assess data fusion for heart rate estimation from simultaneous in-ear ECG and in-ear PPG, recorded on ten subjects while performing 5-min sitting and walking tasks. Results: Our findings show that data fusion methods provide a similar level of mean absolute error as the best single-source heart rate estimation but with much lower intra-subject variability, especially during walking activities. Conclusion: We conclude that data fusion methods provide more robust HR estimation than a single cardiovascular signal. These methods can enhance the performance of wearable devices, especially multimodal hearables, in heart rate tracking during physical activity.
Full article
(This article belongs to the Special Issue Editor's Choice Series for the Applied Biomedical Data Science Section)
►▼
Show Figures
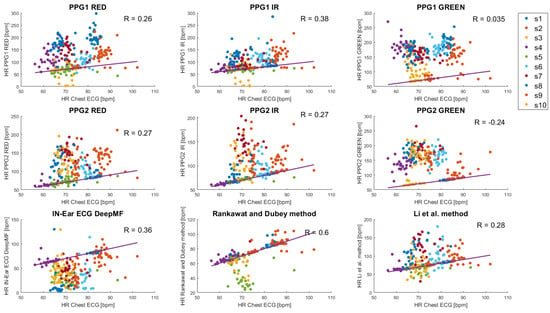
Figure 1
Open AccessArticle
Towards the Generation of Medical Imaging Classifiers Robust to Common Perturbations
by
Joshua Chuah, Pingkun Yan, Ge Wang and Juergen Hahn
BioMedInformatics 2024, 4(2), 889-910; https://doi.org/10.3390/biomedinformatics4020050 - 01 Apr 2024
Abstract
Background: Machine learning (ML) and artificial intelligence (AI)-based classifiers can be used to diagnose diseases from medical imaging data. However, few of the classifiers proposed in the literature translate to clinical use because of robustness concerns. Materials and methods: This study investigates how
[...] Read more.
Background: Machine learning (ML) and artificial intelligence (AI)-based classifiers can be used to diagnose diseases from medical imaging data. However, few of the classifiers proposed in the literature translate to clinical use because of robustness concerns. Materials and methods: This study investigates how to improve the robustness of AI/ML imaging classifiers by simultaneously applying perturbations of common effects (Gaussian noise, contrast, blur, rotation, and tilt) to different amounts of training and test images. Furthermore, a comparison with classifiers trained with adversarial noise is also presented. This procedure is illustrated using two publicly available datasets, the PneumoniaMNIST dataset and the Breast Ultrasound Images dataset (BUSI dataset). Results: Classifiers trained with small amounts of perturbed training images showed similar performance on unperturbed test images compared to the classifier trained with no perturbations. Additionally, classifiers trained with perturbed data performed significantly better on test data both perturbed by a single perturbation (p-values: noise = 0.0186; contrast = 0.0420; rotation, tilt, and blur = 0.000977) and multiple perturbations (p-values: PneumoniaMNIST = 0.000977; BUSI = 0.00684) than the classifier trained with unperturbed data. Conclusions: Classifiers trained with perturbed data were found to be more robust to perturbed test data than the unperturbed classifier without exhibiting a performance decrease on unperturbed test images, indicating benefits to training with data that include some perturbed images and no significant downsides.
Full article
(This article belongs to the Special Issue Advances in Quantitative Imaging Analysis: From Theory to Practice)
►▼
Show Figures
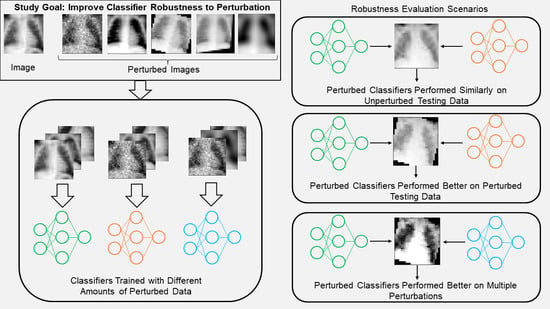
Graphical abstract
Open AccessReview
Exploring the Role of ChatGPT in Oncology: Providing Information and Support for Cancer Patients
by
Maurizio Cè, Vittoria Chiarpenello, Alessandra Bubba, Paolo Florent Felisaz, Giancarlo Oliva, Giovanni Irmici and Michaela Cellina
BioMedInformatics 2024, 4(2), 877-888; https://doi.org/10.3390/biomedinformatics4020049 - 25 Mar 2024
Abstract
Introduction: Oncological patients face numerous challenges throughout their cancer journey while navigating complex medical information. The advent of AI-based conversational models like ChatGPT (San Francisco, OpenAI) represents an innovation in oncological patient management. Methods: We conducted a comprehensive review of the literature on
[...] Read more.
Introduction: Oncological patients face numerous challenges throughout their cancer journey while navigating complex medical information. The advent of AI-based conversational models like ChatGPT (San Francisco, OpenAI) represents an innovation in oncological patient management. Methods: We conducted a comprehensive review of the literature on the use of ChatGPT in providing tailored information and support to patients with various types of cancer, including head and neck, liver, prostate, breast, lung, pancreas, colon, and cervical cancer. Results and Discussion: Our findings indicate that, in most instances, ChatGPT responses were accurate, dependable, and aligned with the expertise of oncology professionals, especially for certain subtypes of cancers like head and neck and prostate cancers. Furthermore, the system demonstrated a remarkable ability to comprehend patients’ emotional responses and offer proactive solutions and advice. Nevertheless, these models have also showed notable limitations and cannot serve as a substitute for the role of a physician under any circumstances. Conclusions: Conversational models like ChatGPT can significantly enhance the overall well-being and empowerment of oncological patients. Both patients and healthcare providers must become well-versed in the advantages and limitations of these emerging technologies.
Full article
(This article belongs to the Special Issue Editor's Choice Series for the Applied Biomedical Data Science Section)
►▼
Show Figures
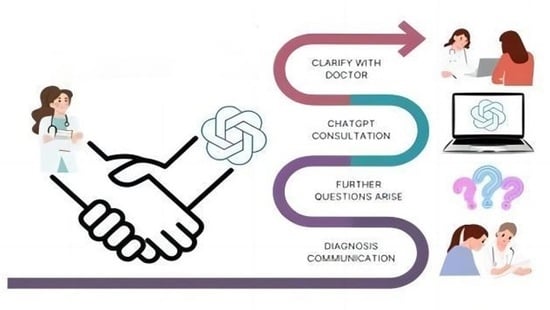
Graphical abstract
Open AccessArticle
Comparing ANOVA and PowerShap Feature Selection Methods via Shapley Additive Explanations of Models of Mental Workload Built with the Theta and Alpha EEG Band Ratios
by
Bujar Raufi and Luca Longo
BioMedInformatics 2024, 4(1), 853-876; https://doi.org/10.3390/biomedinformatics4010048 - 19 Mar 2024
Abstract
Background: Creating models to differentiate self-reported mental workload perceptions is challenging and requires machine learning to identify features from EEG signals. EEG band ratios quantify human activity, but limited research on mental workload assessment exists. This study evaluates the use of theta-to-alpha
[...] Read more.
Background: Creating models to differentiate self-reported mental workload perceptions is challenging and requires machine learning to identify features from EEG signals. EEG band ratios quantify human activity, but limited research on mental workload assessment exists. This study evaluates the use of theta-to-alpha and alpha-to-theta EEG band ratio features to distinguish human self-reported perceptions of mental workload. Methods: In this study, EEG data from 48 participants were analyzed while engaged in resting and task-intensive activities. Multiple mental workload indices were developed using different EEG channel clusters and band ratios. ANOVA’s F-score and PowerSHAP were used to extract the statistical features. At the same time, models were built and tested using techniques such as Logistic Regression, Gradient Boosting, and Random Forest. These models were then explained using Shapley Additive Explanations. Results: Based on the results, using PowerSHAP to select features led to improved model performance, exhibiting an accuracy exceeding 90% across three mental workload indexes. In contrast, statistical techniques for model building indicated poorer results across all mental workload indexes. Moreover, using Shapley values to evaluate feature contributions to the model output, it was noted that features rated low in importance by both ANOVA F-score and PowerSHAP measures played the most substantial role in determining the model output. Conclusions: Using models with Shapley values can reduce data complexity and improve the training of better discriminative models for perceived human mental workload. However, the outcomes can sometimes be unclear due to variations in the significance of features during the selection process and their actual impact on the model output.
Full article
(This article belongs to the Special Issue Feature Papers in Medical Statistics and Data Science Section)
►▼
Show Figures
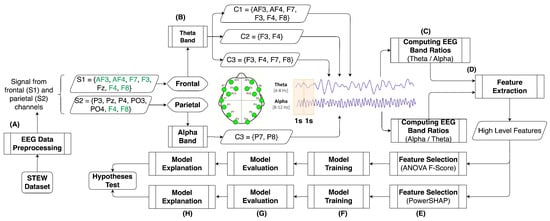
Figure 1
Open AccessReview
Generative Pre-Trained Transformer-Empowered Healthcare Conversations: Current Trends, Challenges, and Future Directions in Large Language Model-Enabled Medical Chatbots
by
James C. L. Chow, Valerie Wong and Kay Li
BioMedInformatics 2024, 4(1), 837-852; https://doi.org/10.3390/biomedinformatics4010047 - 14 Mar 2024
Abstract
This review explores the transformative integration of artificial intelligence (AI) and healthcare through conversational AI leveraging Natural Language Processing (NLP). Focusing on Large Language Models (LLMs), this paper navigates through various sections, commencing with an overview of AI’s significance in healthcare and the
[...] Read more.
This review explores the transformative integration of artificial intelligence (AI) and healthcare through conversational AI leveraging Natural Language Processing (NLP). Focusing on Large Language Models (LLMs), this paper navigates through various sections, commencing with an overview of AI’s significance in healthcare and the role of conversational AI. It delves into fundamental NLP techniques, emphasizing their facilitation of seamless healthcare conversations. Examining the evolution of LLMs within NLP frameworks, the paper discusses key models used in healthcare, exploring their advantages and implementation challenges. Practical applications in healthcare conversations, from patient-centric utilities like diagnosis and treatment suggestions to healthcare provider support systems, are detailed. Ethical and legal considerations, including patient privacy, ethical implications, and regulatory compliance, are addressed. The review concludes by spotlighting current challenges, envisaging future trends, and highlighting the transformative potential of LLMs and NLP in reshaping healthcare interactions.
Full article
(This article belongs to the Special Issue Computational Biology and Artificial Intelligence in Medicine)
►▼
Show Figures
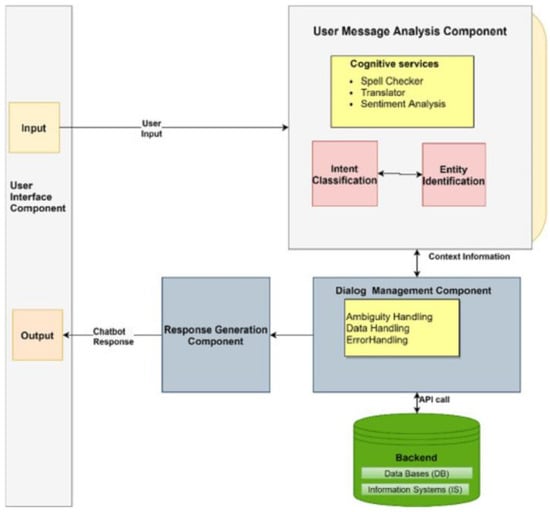
Figure 1
Open AccessArticle
Overall Survival Time Estimation for Epithelioid Peritoneal Mesothelioma Patients from Whole-Slide Images
by
Kleanthis Marios Papadopoulos, Panagiotis Barmpoutis, Tania Stathaki, Vahan Kepenekian, Peggy Dartigues, Séverine Valmary-Degano, Claire Illac-Vauquelin, Gerlinde Avérous, Anne Chevallier, Marie-Hélène Laverriere, Laurent Villeneuve, Olivier Glehen, Sylvie Isaac, Juliette Hommell-Fontaine, Francois Ng Kee Kwong and Nazim Benzerdjeb
BioMedInformatics 2024, 4(1), 823-836; https://doi.org/10.3390/biomedinformatics4010046 - 13 Mar 2024
Abstract
Background: The advent of Deep Learning initiated a new era in which neural networks relying solely on Whole-Slide Images can estimate the survival time of cancer patients. Remarkably, despite deep learning’s potential in this domain, no prior research has been conducted on image-based
[...] Read more.
Background: The advent of Deep Learning initiated a new era in which neural networks relying solely on Whole-Slide Images can estimate the survival time of cancer patients. Remarkably, despite deep learning’s potential in this domain, no prior research has been conducted on image-based survival analysis specifically for peritoneal mesothelioma. Prior studies performed statistical analysis to identify disease factors impacting patients’ survival time. Methods: Therefore, we introduce MPeMSupervisedSurv, a Convolutional Neural Network designed to predict the survival time of patients diagnosed with this disease. We subsequently perform patient stratification based on factors such as their Peritoneal Cancer Index and on whether patients received chemotherapy treatment. Results: MPeMSupervisedSurv demonstrates improvements over comparable methods. Using our proposed model, we performed patient stratification to assess the impact of clinical variables on survival time. Notably, the inclusion of information regarding adjuvant chemotherapy significantly enhances the model’s predictive prowess. Conversely, repeating the process for other factors did not yield significant performance improvements. Conclusions: Overall, MPeMSupervisedSurv is an effective neural network which can predict the survival time of peritoneal mesothelioma patients. Our findings also indicate that treatment by adjuvant chemotherapy could be a factor affecting survival time.
Full article
(This article belongs to the Special Issue Deep Learning Methods and Application for Bioinformatics and Healthcare)
►▼
Show Figures
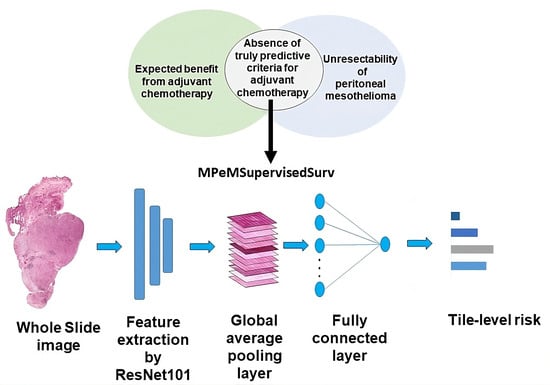
Graphical abstract
Open AccessArticle
Genetic Optimization in Uncovering Biologically Meaningful Gene Biomarkers for Glioblastoma Subtypes
by
Petros Paplomatas, Ioanna-Efstathia Douroumi, Panagiotis Vlamos and Aristidis Vrahatis
BioMedInformatics 2024, 4(1), 811-822; https://doi.org/10.3390/biomedinformatics4010045 - 08 Mar 2024
Abstract
Background: Glioblastoma multiforme (GBM) is a highly aggressive brain cancer known for its challenging survival rates; it is characterized by distinct subtypes, such as the proneural and mesenchymal states. The development of targeted therapies is critically dependent on a thorough understanding of these
[...] Read more.
Background: Glioblastoma multiforme (GBM) is a highly aggressive brain cancer known for its challenging survival rates; it is characterized by distinct subtypes, such as the proneural and mesenchymal states. The development of targeted therapies is critically dependent on a thorough understanding of these subtypes. Advances in single-cell RNA-sequencing (scRNA-seq) have opened new avenues for identifying subtype-specific gene biomarkers, which are essential for innovative treatments. Methods: This study introduces a genetic optimization algorithm designed to select a precise set of genes that clearly differentiate between the proneural and mesenchymal GBM subtypes. By integrating differential gene expression analysis with gene variability assessments, our dual-criterion strategy ensures the selection of genes that are not only differentially expressed between subtypes but also exhibit consistent variability patterns. This approach enhances the biological relevance of identified biomarkers. We applied this algorithm to scRNA-seq data from GBM samples, focusing on the discovery of subtype-specific gene biomarkers. Results: The application of our genetic optimization algorithm to scRNA-seq data successfully identified significant genes that are closely associated with the fundamental characteristics of GBM. These genes show a strong potential to distinguish between the proneural and mesenchymal subtypes, offering insights into the molecular underpinnings of GBM heterogeneity. Conclusions: This study introduces a novel approach for biomarker discovery in GBM that is potentially applicable to other complex diseases. By leveraging scRNA-seq data, our method contributes to the development of targeted therapies, highlighting the importance of precise biomarker identification in personalized medicine.
Full article
(This article belongs to the Special Issue Editor's Choice Series for the Applied Biomedical Data Science Section)
►▼
Show Figures
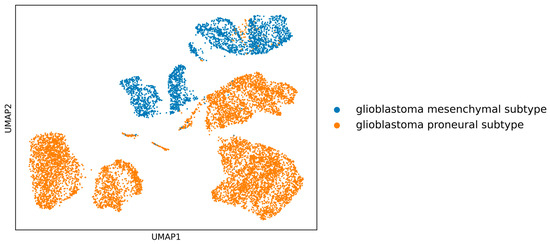
Figure 1
Open AccessArticle
Pediatric and Adolescent Seizure Detection: A Machine Learning Approach Exploring the Influence of Age and Sex in Electroencephalogram Analysis
by
Lan Wei and Catherine Mooney
BioMedInformatics 2024, 4(1), 796-810; https://doi.org/10.3390/biomedinformatics4010044 - 06 Mar 2024
Abstract
Background: Epilepsy, a prevalent neurological disorder characterized by recurrent seizures affecting an estimated 70 million people worldwide, poses a significant diagnostic challenge. EEG serves as an important tool in identifying these seizures, but the manual examination of EEGs by experts is time-consuming. To
[...] Read more.
Background: Epilepsy, a prevalent neurological disorder characterized by recurrent seizures affecting an estimated 70 million people worldwide, poses a significant diagnostic challenge. EEG serves as an important tool in identifying these seizures, but the manual examination of EEGs by experts is time-consuming. To expedite this process, automated seizure detection methods have emerged as powerful aids for expert EEG analysis. It is worth noting that while such methods are well-established for adult EEGs, they have been underdeveloped for pediatric and adolescent EEGs. This study sought to address this gap by devising an automatic seizure detection system tailored for pediatric and adolescent EEG data. Methods: Leveraging publicly available datasets, the TUH pediatric and adolescent EEG and CHB-MIT EEG datasets, the machine learning-based models were constructed. The TUH pediatric and adolescent EEG dataset was divided into training (n = 118), validation (n = 19), and testing (n = 37) subsets, with special attention to ensure a clear demarcation between the individuals in the training and test sets to preserve the test set’s independence. The CHB-MIT EEG dataset was used as an external test set. Age and sex were incorporated as features in the models to investigate their potential influence on seizure detection. Results: By leveraging 20 features extracted from both time and frequency domains, along with age as an additional feature, the method achieved an accuracy of 98.95% on the TUH test set and 64.82% on the CHB-MIT external test set. Our investigation revealed that age is a crucial factor for accurate seizure detection in pediatric and adolescent EEGs. Conclusion: The outcomes of this study hold substantial promise in supporting researchers and clinicians engaged in the automated analysis of seizures in pediatric and adolescent EEGs.
Full article
(This article belongs to the Special Issue Editor-in-Chief's Choices in Biomedical Informatics)
►▼
Show Figures
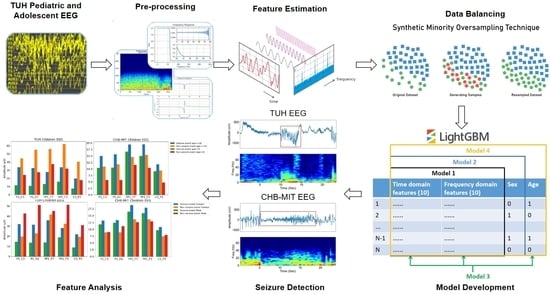
Graphical abstract
Highly Accessed Articles
Latest Books
E-Mail Alert
News
Topics
Topic in
BioMedInformatics, Cancers, Current Oncology, Hemato, Hematology Reports
Myeloma and Leukemia-Challenges and Current Treatment Options
Topic Editors: Giovanni Martinelli, Claudio CerchioneDeadline: 20 June 2024
Topic in
Algorithms, BDCC, BioMedInformatics, Information, Mathematics
Machine Learning Empowered Drug Screen
Topic Editors: Teng Zhou, Jiaqi Wang, Youyi SongDeadline: 31 August 2024
Topic in
Biology, BioMedInformatics, Cancers, Genes, IJMS
The 22nd International Conference on Bioinformatics (InCoB 2023): Translational Bioinformatics Transforming Life
Topic Editors: Jyotsna Batra, Srilakshmi Srinivasan, Shoba Ranganathan, Asif M. Khan, Harpreet SinghDeadline: 15 November 2024
Topic in
Biology, Cancers, Molecules, IJMS, BioMedInformatics, Current Oncology
Learning Machines and Drug Discovery: A New Era in Cancer
Topic Editors: Satwinderjeet Kaur, Atiah H. AlmalkiDeadline: 20 December 2024

Conferences
Special Issues
Special Issue in
BioMedInformatics
Features of Bioinformatic Analyses for SARS-CoV-2 Infections and Vaccination
Guest Editor: Yasunari MatsuzakaDeadline: 30 June 2024
Special Issue in
BioMedInformatics
Advances in Quantitative Imaging Analysis: From Theory to Practice
Guest Editors: Federico Mastroleo, Angela Ammirabile, Giulia MarvasoDeadline: 30 November 2024
Special Issue in
BioMedInformatics
Advances in Structural Bioinformatics and Next-Generation Sequence Analysis for Drug Design
Guest Editor: Alexandre G. De BrevernDeadline: 31 December 2024