Information Complexity in Structured Data
A special issue of Entropy (ISSN 1099-4300). This special issue belongs to the section "Complexity".
Deadline for manuscript submissions: closed (15 February 2022) | Viewed by 13144
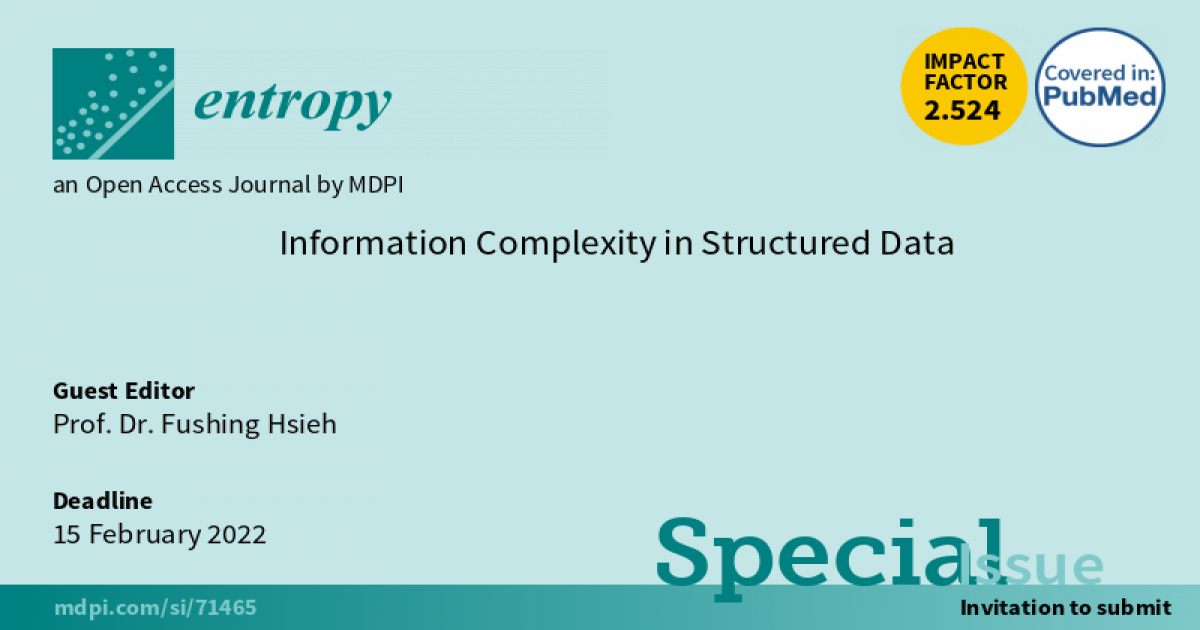
Special Issue Editor
Special Issue Information
Structured data, including high-dimensional time series, represented in a form of data matrix, are the most ubiquitous data format in data analysis. Nevertheless, fundamental questions in analyzing a data matrix remain widely open and yet to be resolved. For instance, Euclidean distance is too simplistic for similarity among subjects, while correlation only measures the linear relationship between two continuous features. It is not suitable for categorical features, which is considered the most fundamental data type. On the other hand, it is now well known that directional association measures based on conditional Shannon entropy are non-linear. Since they are good for all data types, such directional associations also allow us to replace linearity- or functionality-based modeling for inferential purposes, and at the same time allow us to accommodate multiple response variables. It is expected that computational approaches based on Shannon entropy could and would resolve the ultimate tasks in data analysis: finding out a data matrix’s full information content. Such information content surely contains dependency structures constituted by all involving features in a collective fashion. Further, patterns of such structural dependency could be identified and collected into a collection of system states. When their temporal coordinates are recovered, the data’s complexity can be evaluated through various complexity measures, such as Lampel–Ziv complexity. From this perspective, data analysis is free from any stationarity requirement.
Data analysis on a data matrix is far from being settled. There are diverse fundamental and interesting issues to be recognized and resolved from many real-world applications in sciences and in industry. By not ignoring the categorical nature of all data types, combinatorial information theory would become critically relevant. Specifically, computational approaches based on Shannon entropy would play critical roles in every aspect of data analysis. We emphasize on discovering visible and explainable pattern-based information content embraced by a data matrix. Contributions aiming for such a goal of data analysis would be very much welcome.
This Special Issue intends to be a forum for developing and applying computational techniques of combinatorial information theory for a better understanding of real-world complex systems. All pattern-discovering approaches based on Shannon information theory are considered to be within the scope of this Special Issue.
Prof. Dr. Fushing Hsieh
Guest Editor
Manuscript Submission Information
Manuscripts should be submitted online at www.mdpi.com by registering and logging in to this website. Once you are registered, click here to go to the submission form. Manuscripts can be submitted until the deadline. All submissions that pass pre-check are peer-reviewed. Accepted papers will be published continuously in the journal (as soon as accepted) and will be listed together on the special issue website. Research articles, review articles as well as short communications are invited. For planned papers, a title and short abstract (about 100 words) can be sent to the Editorial Office for announcement on this website.
Submitted manuscripts should not have been published previously, nor be under consideration for publication elsewhere (except conference proceedings papers). All manuscripts are thoroughly refereed through a single-blind peer-review process. A guide for authors and other relevant information for submission of manuscripts is available on the Instructions for Authors page. Entropy is an international peer-reviewed open access monthly journal published by MDPI.
Please visit the Instructions for Authors page before submitting a manuscript. The Article Processing Charge (APC) for publication in this open access journal is 2600 CHF (Swiss Francs). Submitted papers should be well formatted and use good English. Authors may use MDPI's English editing service prior to publication or during author revisions.
Benefits of Publishing in a Special Issue
- Ease of navigation: Grouping papers by topic helps scholars navigate broad scope journals more efficiently.
- Greater discoverability: Special Issues support the reach and impact of scientific research. Articles in Special Issues are more discoverable and cited more frequently.
- Expansion of research network: Special Issues facilitate connections among authors, fostering scientific collaborations.
- External promotion: Articles in Special Issues are often promoted through the journal's social media, increasing their visibility.
- e-Book format: Special Issues with more than 10 articles can be published as dedicated e-books, ensuring wide and rapid dissemination.
Further information on MDPI's Special Issue polices can be found here.