Applications of Information Theory in Statistics
A special issue of Entropy (ISSN 1099-4300). This special issue belongs to the section "Information Theory, Probability and Statistics".
Deadline for manuscript submissions: closed (20 June 2022) | Viewed by 24112
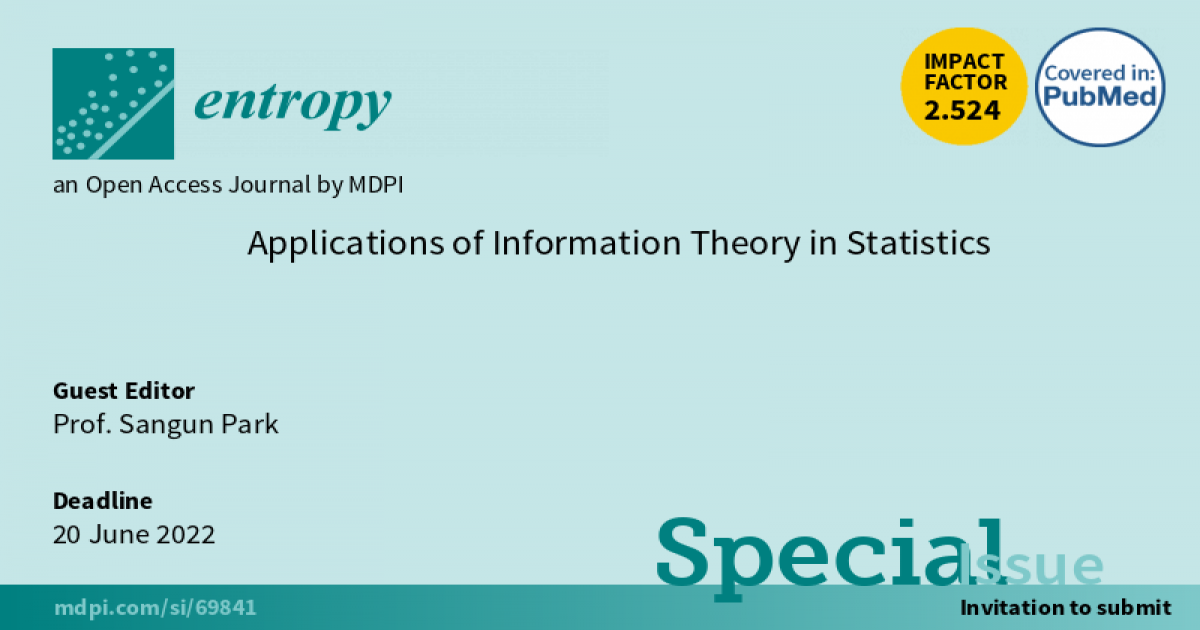
Special Issue Editor
Special Issue Information
Dear Colleagues,
It is well known that several important topics in statistics are highly related to information theory—maximum likelihood and cross entropy, Akaike information criterion and Kullback–Leibler information, sum of squares and mutual information, etc. Though Fisher information is the most important measure of information in parametric statistical inference and related science, information theory is a popular topic related to nonparametric statistical inference and machine learning methods in statistics today. Thus, gathering relevant works on the applications of information theory in statistics is of growing importance.
This Special Issue aims to serve as a forum for the interpretation of information theory in terms of statistics. All topics related to information theory and statistics, which include the applications of information theory in statistics or applications of statistical concepts to information theory, fall within the scope of this Special Issue.
Prof. Sangun Park
Guest Editor
Manuscript Submission Information
Manuscripts should be submitted online at www.mdpi.com by registering and logging in to this website. Once you are registered, click here to go to the submission form. Manuscripts can be submitted until the deadline. All submissions that pass pre-check are peer-reviewed. Accepted papers will be published continuously in the journal (as soon as accepted) and will be listed together on the special issue website. Research articles, review articles as well as short communications are invited. For planned papers, a title and short abstract (about 100 words) can be sent to the Editorial Office for announcement on this website.
Submitted manuscripts should not have been published previously, nor be under consideration for publication elsewhere (except conference proceedings papers). All manuscripts are thoroughly refereed through a single-blind peer-review process. A guide for authors and other relevant information for submission of manuscripts is available on the Instructions for Authors page. Entropy is an international peer-reviewed open access monthly journal published by MDPI.
Please visit the Instructions for Authors page before submitting a manuscript. The Article Processing Charge (APC) for publication in this open access journal is 2600 CHF (Swiss Francs). Submitted papers should be well formatted and use good English. Authors may use MDPI's English editing service prior to publication or during author revisions.
Keywords
- estimation and testing
- information theory
- statistics
- applications
- Fisher information
Benefits of Publishing in a Special Issue
- Ease of navigation: Grouping papers by topic helps scholars navigate broad scope journals more efficiently.
- Greater discoverability: Special Issues support the reach and impact of scientific research. Articles in Special Issues are more discoverable and cited more frequently.
- Expansion of research network: Special Issues facilitate connections among authors, fostering scientific collaborations.
- External promotion: Articles in Special Issues are often promoted through the journal's social media, increasing their visibility.
- e-Book format: Special Issues with more than 10 articles can be published as dedicated e-books, ensuring wide and rapid dissemination.
Further information on MDPI's Special Issue policies can be found here.