Topic Editors

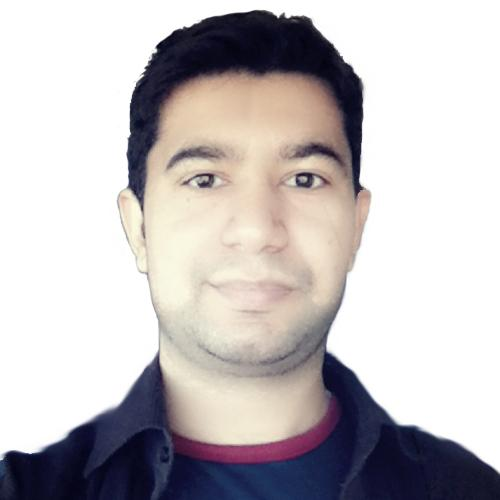
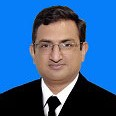
Multimodal Sentiment Analysis Based on Deep Learning Methods Such as Convolutional Neural Networks
Topic Information
Dear Colleagues,
This Special Issue is aimed at researchers who are working on large scale data with real-word problem solving. Every social media app is generating data in GBs that require researcher attention for identifying useful patterns and information. Due to globalization, every social media content is shared and commented on by diverse background users. The data contain several opinions in different languages on the similar topics. The classical approach for text classification, i.e., sentiment analysis, mainly relies on NLP techniques related to single language. However, it is important to propose a model that can learn features from multilingual data. Submissions on this issue focused on theoretical expansion and also the applications of sentiment analysis on our daily life are invited. Topics of interest include but are not limited to the following areas:
- Text classification;
- Opinion mining;
- Visualization of opinions;
- Social network analysis for sentiment analysis;
- Multi-model learning for text classification;
- Multi-lingual sentiment analysis;
- Applications for sentiment analysis;
- Explainable artificial intelligence for sentiment analysis;
- Aspect-based sentiment analysis;
- Hate speech detection;
- Sarcasm and irony detection.
Dr. Junaid Baber
Dr. Ali Shariq Imran
Prof. Dr. Doudpota Sher
Dr. Bakhtyar Maheen
Topic Editors
Keywords
- Â text classification
- Â opinion mining
- Â visualization of opinions
- Â social network analysis for sentiment analysis
- Â multi-model learning for text classification
- Â multi-lingual sentiment analysis
- Â applications for sentiment analysis
- Â explainable artificial intelligence for sentiment analysis
- Â deep learning for text classification
Participating Journals
Journal Name | Impact Factor | CiteScore | Launched Year | First Decision (median) | APC | |
---|---|---|---|---|---|---|
![]()
Algorithms
|
1.8 | 4.1 | 2008 | 15 Days | CHF 1600 | Submit |
![]()
Axioms
|
1.9 | - | 2012 | 21.8 Days | CHF 2400 | Submit |
![]()
Future Internet
|
2.8 | 7.1 | 2009 | 11.8 Days | CHF 1600 | Submit |
![]()
Mathematics
|
2.3 | 4.0 | 2013 | 16.9 Days | CHF 2600 | Submit |
![]()
Symmetry
|
2.2 | 5.4 | 2009 | 16.2 Days | CHF 2400 | Submit |
MDPI Topics is cooperating with Preprints.org and has built a direct connection between MDPI journals and Preprints.org. Authors are encouraged to enjoy the benefits by posting a preprint at Preprints.org prior to publication:
- Immediately share your ideas ahead of publication and establish your research priority;
- Protect your idea from being stolen with this time-stamped preprint article;
- Enhance the exposure and impact of your research;
- Receive feedback from your peers in advance;
- Have it indexed in Web of Science (Preprint Citation Index), Google Scholar, Crossref, SHARE, PrePubMed, Scilit and Europe PMC.