Topic Menu
► Topic MenuTopic Editors


Application of Remote Sensing in Forest Fire
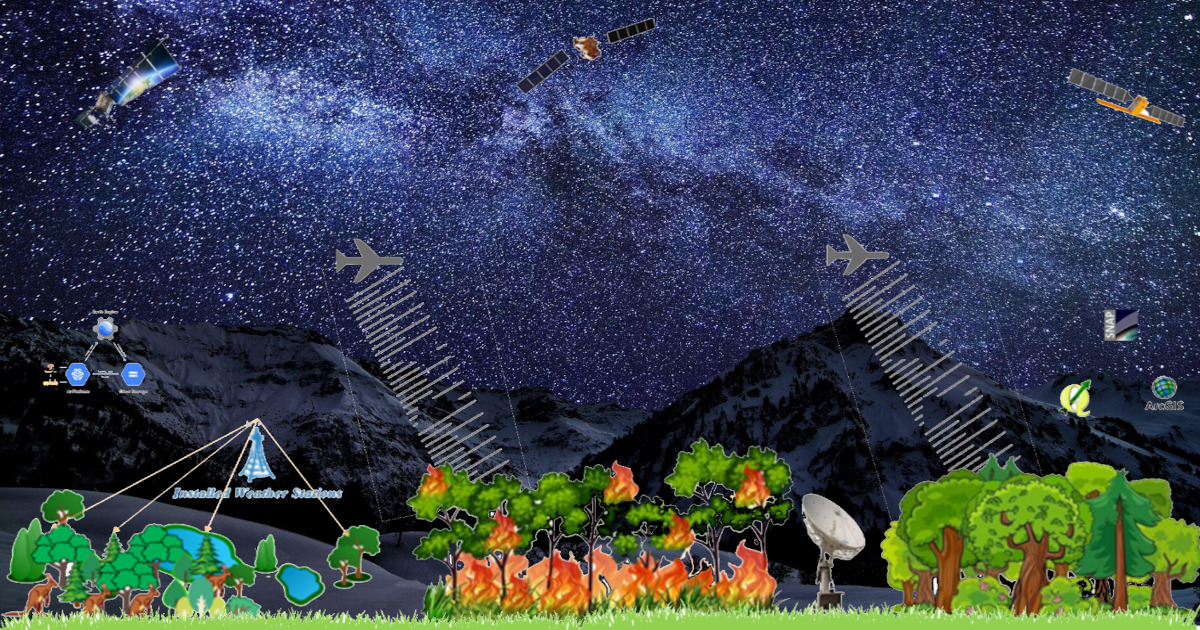
Topic Information
Dear Colleagues,
Forest fires are amongst the most prominent disturbance factors in most vegetation zones throughout the world, such as forests and grasslands. Forest fires present a challenge for ecosystem management because of their potential to be at once beneficial and harmful. Drones, unmanned aerial vehicle (UAV) applications and remote sensing technology can be incredibly valuable in assessing forest fire risk over large areas. For example, remote sensing can be used to monitor changes in vegetation that may be the result of invasive species or especially dry conditions. Technology plays an important role in preventing and responding to wildfires. For example, there are numerous remote sensing and geographic information systems (GIS) applications in forest fire management.
Fire detection is a critical stage of wildfire management, which is aimed at either fighting or monitoring the fire. For firefighting, early detection is essential; to date, fire detection for firefighting has been based on human observation, the use of fixed optical cameras to monitor the surrounding environment, or aerial survey. Forest fire managers do not consider the revisit time provided by current satellite sensors sufficient for firefighting operations. However, the monitoring of forest fire and forest fire effects for large territories is mainly based on satellite remote sensing. Mapping of burnt areas and assessment of forest fire effects is one of the most successful applications of satellite remote sensing. Satellite remote sensing provides the means to acquire comprehensive and harmonized information on wildfire effects over large territories at a low cost. For this purpose, burnt area mapping is performed with a wide variety of remote sensors and techniques. A wide variety of optical and radar sensors have been used for fire detection and burnt area mapping, from local to global scales. This section reviews the application of remote sensing in active fire detection and the assessment of fire damage through the mapping of the extent of burnt areas.
This Topic will include papers addressing various aspects of remote sensing applications in forest fires across the globe. It aspires to confront the specific challenges of various aspects of remote sensing applications in the monitoring of forest fire management systems effectively. It addresses the modest trends and benefits of application of remote sensing in forest fire.
Manuscripts should cover, but are not limited to, the following topics:
- Fire detection and burnt area mapping;
- Prediction and real-time monitoring of forest fires;
- Forest fire risk modeling and management;
- Fuel analysis, modeling and prevention;
- Mapping of fire risk zones;
- GIS applications in forest fire management;
- Regrowth of vegetation aftermath of ablaze;
- Monitoring fire-prone areas;
- UAVs to survey damage to plant life;
- Mapping of burnt scars for recovery;
- Radar images for pre-fire and post-fire conditions monitoring.
Dr. Aqil Tariq
Dr. Na Zhao
Topic Editors
Keywords
- remote sensing and GIS
- SAR
- forest fire
- wildfire
- postfire regeneration
- optical remote sensing
- fire severity
- fire mapping
- unmannaged aerial vehicles
Participating Journals
Journal Name | Impact Factor | CiteScore | Launched Year | First Decision (median) | APC |
---|---|---|---|---|---|
![]()
Drones
|
4.8 | 6.1 | 2017 | 17.9 Days | CHF 2600 |
![]()
Fire
|
3.2 | 3.5 | 2018 | 15 Days | CHF 2400 |
![]()
Forests
|
2.9 | 4.5 | 2010 | 16.9 Days | CHF 2600 |
![]()
Remote Sensing
|
5.0 | 7.9 | 2009 | 23 Days | CHF 2700 |
![]()
Sustainability
|
3.9 | 5.8 | 2009 | 18.8 Days | CHF 2400 |
MDPI Topics is cooperating with Preprints.org and has built a direct connection between MDPI journals and Preprints.org. Authors are encouraged to enjoy the benefits by posting a preprint at Preprints.org prior to publication:
- Immediately share your ideas ahead of publication and establish your research priority;
- Protect your idea from being stolen with this time-stamped preprint article;
- Enhance the exposure and impact of your research;
- Receive feedback from your peers in advance;
- Have it indexed in Web of Science (Preprint Citation Index), Google Scholar, Crossref, SHARE, PrePubMed, Scilit and Europe PMC.