Topic Editors
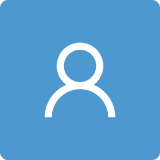
Visual Computing and Understanding: New Developments and Trends
Topic Information
Dear Colleagues,
We as humans have to handle massive amounts of visual information in our daily lives. As a result, there has been recent growing interest in the advancement of artificial intelligence-based perception and analysis algorithms in the field of computer vision and image processing.
Despite significant successes in visual computing and understanding in recent years, new developments and trends in the methods in which these achievements are made are still in their infancy, especially for many complex real-world applications.
The aim of this topic is to progress this field by collecting research on both the theorical and applied issues related to advances in visual computing and understanding. All interested authors are invited to submit their innovative manuscripts on (but are not limited to) the following topics:
- Image/video acquisition, fusion, and generation;
- Image/video coding, restoration, and quality assessment;
- Image/video classification, segmentation, and detection;
- Deep learning-based methods for image processing and analysis;
- Deep learning-based methods for video processing and analysis;
- Deep learning-based computer vision methods for 3D models;
- Intelligent vision methods for autonomous driving systems;
- Robotic vision and its applications;
- Biomedical vision analysis and applications;
- Advances in visual computing theories.
Dr. Wei Zhou
Dr. Guanghui Yue
Dr. Wenhan Yang
Topic Editors
Keywords
- image processing and video processing
- visual computing and deep learning
- computer vision and robotic vision
- autonomous driving
- biomedical vision
- image acquisition and image fusion
- generative models
- video coding and image restoration
- quality assessment
- visual understanding
- feature extraction and object detection
- image classification
- semantic segmentation
- saliency detection
- perception modelling
Participating Journals
Journal Name | Impact Factor | CiteScore | Launched Year | First Decision (median) | APC | |
---|---|---|---|---|---|---|
![]()
Applied Sciences
|
2.5 | 5.3 | 2011 | 18.4 Days | CHF 2400 | Submit |
![]()
Computers
|
2.6 | 5.4 | 2012 | 15.5 Days | CHF 1800 | Submit |
![]()
Electronics
|
2.6 | 5.3 | 2012 | 16.4 Days | CHF 2400 | Submit |
![]()
Information
|
2.4 | 6.9 | 2010 | 16.4 Days | CHF 1600 | Submit |
![]()
Journal of Imaging
|
2.7 | 5.9 | 2015 | 18.3 Days | CHF 1800 | Submit |
Preprints.org is a multidisciplinary platform offering a preprint service designed to facilitate the early sharing of your research. It supports and empowers your research journey from the very beginning.
MDPI Topics is collaborating with Preprints.org and has established a direct connection between MDPI journals and the platform. Authors are encouraged to take advantage of this opportunity by posting their preprints at Preprints.org prior to publication:
- Share your research immediately: disseminate your ideas prior to publication and establish priority for your work.
- Safeguard your intellectual contribution: Protect your ideas with a time-stamped preprint that serves as proof of your research timeline.
- Boost visibility and impact: Increase the reach and influence of your research by making it accessible to a global audience.
- Gain early feedback: Receive valuable input and insights from peers before submitting to a journal.
- Ensure broad indexing: Web of Science (Preprint Citation Index), Google Scholar, Crossref, SHARE, PrePubMed, Scilit and Europe PMC.