Topic Menu
► Topic MenuTopic Editors

2. Department of Electronics and Computer Engineering, Ngee Ann Polytechnic, Singapore 599489, Singapore
3. Department of Biomedical Engineering, School of Science and Technology, SUSS University, Singapore 599494, Singapore
4. Department of Biomedical Informatics and Medical Engineering, Asia University, Taichung 41354, Taiwan
5. School of Business (Information Systems), Faculty of Business, Education, Law & Arts, University of Southern Queensland, Toowoomba, QLD, Australia
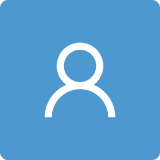
Long-Term Health Monitoring with Physiological Signals - Volume 2
Topic Information
Dear Colleagues,
The human body constantly produces physiological signals such as heat and electrical impulses from the muscles, brain, and other organs. Since the dawn of modern medicine, these signals have been used to assess and, in some cases, determine the cause of a health crisis. From these ancient roots, modern medicine has grown and diversified. However, the idea of crisis is still preserved. Like Hippocrates of Kos, modern medicine springs into action when a health crisis occurs. This event-driven setup is very resource efficient because health services are only used if there is reason to do so. However, sometimes a disease might have progressed beyond a point where effective treatment is available before symptoms trigger a diagnosis. In the past, resource efficiency by far outweighed the potential benefits of continuous physiological signal monitoring. However, in recent years, technological advances have meant that communication, storage, and processing resources have become almost omnipresent at a competitive price point. Having recognized the transformative nature of this technology, for this topic, entitled “Long-Term Health Monitoring with Physiological Signals”, we seek answers to the question: how can we use physiological signal measurements to translate the resource abundance into improved outcomes for patients? We invite papers that recognize the potential of gathering and analysing big physiological data for possible publication in one of the five journals: Diagnostics, Life, Healthcare, BioMed, or Signals. A possible application area is the creation of disease-specific solutions where physiological signals are analysed with advanced artificial intelligence algorithms. Examples include atrial fibrillation detection and sleep monitoring in the home environment. Another area of interest is long-term physiological signal analysis for rehabilitation tracking and geriatric care.
Prof. Dr. U Rajendra Acharya
Dr. Oliver Faust
Topic Editors
Keywords
- physiological signals
- internet of medical things
- mobile health
- long-term monitoring
- artificial intelligence
- hybrid medical decision support
- rehabilitation
- geriatric care
Participating Journals
Journal Name | Impact Factor | CiteScore | Launched Year | First Decision (median) | APC |
---|---|---|---|---|---|
![]()
BioMed
|
- | - | 2021 | 21.9 Days | CHF 1000 |
![]()
Diagnostics
|
3.0 | 4.7 | 2011 | 20.3 Days | CHF 2600 |
![]()
Healthcare
|
2.4 | 3.5 | 2013 | 20.3 Days | CHF 2700 |
![]()
Life
|
3.2 | 4.3 | 2011 | 17.8 Days | CHF 2600 |
![]()
Signals
|
- | 3.2 | 2020 | 28.3 Days | CHF 1000 |
Preprints.org is a multidisciplinary platform offering a preprint service designed to facilitate the early sharing of your research. It supports and empowers your research journey from the very beginning.
MDPI Topics is collaborating with Preprints.org and has established a direct connection between MDPI journals and the platform. Authors are encouraged to take advantage of this opportunity by posting their preprints at Preprints.org prior to publication:
- Share your research immediately: disseminate your ideas prior to publication and establish priority for your work.
- Safeguard your intellectual contribution: Protect your ideas with a time-stamped preprint that serves as proof of your research timeline.
- Boost visibility and impact: Increase the reach and influence of your research by making it accessible to a global audience.
- Gain early feedback: Receive valuable input and insights from peers before submitting to a journal.
- Ensure broad indexing: Web of Science (Preprint Citation Index), Google Scholar, Crossref, SHARE, PrePubMed, Scilit and Europe PMC.