Topic Menu
► Topic MenuTopic Editors
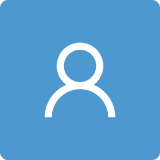
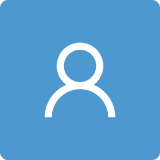
Decision-Making and Data Mining for Sustainable Computing
Topic Information
Dear Colleagues,
Statistical and machine learning (ML) approaches to artificial intelligence (AI) have been successfully implemented in the predictive applications of several domains of science and engineering in recent years. The ML algorithms of AI are vital components for the development of automated, accurate, and robust prediction systems after analysis of the data for each specific application. Improved accuracy of the statistical and ML approaches to AI is crucial in each of the applications of predictive modeling in many domains, including healthcare, agriculture, space, etc. The development of advanced ML approaches and their implementation in the analysis of experimental and simulated data is challenging research at present. With this objective, the present Issue invites researchers and academicians to submit their novel and unpublished research outcomes related to the current development of statistical and ML approaches to AI in science- and engineering-related predictive modeling applications. This Special Issue is the first in the fields of decision-making and data mining for sustainable computing, and it will cover a broad range of topics related to applications of ML approaches in the analysis of data, not limited to the following subtopics.
Dr. Sunil Jha
Dr. Malgorzata Rataj
Dr. Xiaorui Zhang
Topic Editors
Keywords
- machine learning
- data mining
- predictive modeling
- intelligent forecasting
- sustainable computing
- decision making
Participating Journals
Journal Name | Impact Factor | CiteScore | Launched Year | First Decision (median) | APC |
---|---|---|---|---|---|
![]()
Algorithms
|
1.8 | 4.1 | 2008 | 18.9 Days | CHF 1600 |
![]()
Data
|
2.2 | 4.3 | 2016 | 26.8 Days | CHF 1600 |
![]()
Information
|
2.4 | 6.9 | 2010 | 16.4 Days | CHF 1600 |
![]()
Mathematics
|
2.3 | 4.0 | 2013 | 18.3 Days | CHF 2600 |
![]()
Symmetry
|
2.2 | 5.4 | 2009 | 17.3 Days | CHF 2400 |
MDPI Topics is cooperating with Preprints.org and has built a direct connection between MDPI journals and Preprints.org. Authors are encouraged to enjoy the benefits by posting a preprint at Preprints.org prior to publication:
- Immediately share your ideas ahead of publication and establish your research priority;
- Protect your idea from being stolen with this time-stamped preprint article;
- Enhance the exposure and impact of your research;
- Receive feedback from your peers in advance;
- Have it indexed in Web of Science (Preprint Citation Index), Google Scholar, Crossref, SHARE, PrePubMed, Scilit and Europe PMC.