Topic Menu
► Topic MenuTopic Editors

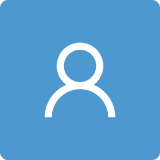
Applied Sciences and Technologies for Detection and Therapies of Pathologies in the Neuronal Environment
Topic Information
Dear Colleagues,
In the central nervous system (CNS), a part of the nervous system that involves the brain and spinal cord, various pathogenic factors, such as neurodegenerative diseases, neuro-oncological diseases, neurovascular disorders, and traumatic insults to vital neural structures, may lead to permanent neural conduction impairment, neuronal metabolic dysfunction, and eventually, the progressive loss of the structure or function of neural cells, including irreversible damage of neurons. Most patients with these neural pathologies display evidence of collapsed recognition, and can potentially become severely disabled, which can lead to increased costs associated with long-term health care.
The above-mentioned disease categories are usually accompanied by minor pathological alternations, such as chronic inflammatory responses in neural micro-environments. The act of detecting and targeting neural pathologies at specific time-points holds great promise for the application of multidisciplinary diagnostic strategies. These uses of applied science and technologies involve molecular imaging techniques, bioinformatics analysis, artificial intelligence (AI)-based strategies, as well as molecular therapeutic targeting with gene and cell therapy. Advancements in the early detection of disease progression would promote more precise treatments with satisfactory outcomes.
The aim of this Research Topic is to bring together leading research from a multidisciplinary perspective and updated insights into the most recent advances in the fundamental and applied issues that underlie the recent advances in neural pathologies. Reviews and original articles are welcome.
Potential topics include, but are not limited to, the following:
- Development and application of related biomarkers to the diagnosis, treatment, and clinical outcome of neural pathologies;
- Risk or protective factors, including neuroinflammation in cells, in innovative animal research for neural pathologies;
- Development of novel bench to bedside applications that are relevant to the diagnosis, treatment, and clinical outcome of neural pathologies;
- Structural or functional imaging that could reflect or predict the development of neural pathologies;
- Potential pharmacological/complementary/alternative medicine or other interventions that include traditional approaches for patients with neural pathologies;
- Population-based studies for the prediction of prognoses and respective pharmacological interventions that correlate with neural pathologies;
- Novel neuro-epidemiological modeling approaches for data interpretation in bioinformatics and computational biology;
- Meta-analyses or systemic reviews of various neural pathologies.
Dr. Muh-Shi Lin
Prof. Dr. Hong Jiang
Dr. Yu-Yo Sun
Topic Editors
Participating Journals
Journal Name | Impact Factor | CiteScore | Launched Year | First Decision (median) | APC |
---|---|---|---|---|---|
![]()
Brain Sciences
|
2.7 | 4.8 | 2011 | 15.6 Days | CHF 2200 |
![]()
Cells
|
5.1 | 9.9 | 2012 | 16.6 Days | CHF 2700 |
![]()
Diagnostics
|
3.0 | 4.7 | 2011 | 20.7 Days | CHF 2600 |
![]()
International Journal of Molecular Sciences
|
4.9 | 8.1 | 2000 | 16.3 Days | CHF 2900 |
![]()
Journal of Clinical Medicine
|
3.0 | 5.7 | 2012 | 17.9 Days | CHF 2600 |
![]()
Pathogens
|
3.3 | 6.4 | 2012 | 16.4 Days | CHF 2700 |
![]()
Pathophysiology
|
2.7 | 3.1 | 1994 | 22.6 Days | CHF 1400 |
MDPI Topics is cooperating with Preprints.org and has built a direct connection between MDPI journals and Preprints.org. Authors are encouraged to enjoy the benefits by posting a preprint at Preprints.org prior to publication:
- Immediately share your ideas ahead of publication and establish your research priority;
- Protect your idea from being stolen with this time-stamped preprint article;
- Enhance the exposure and impact of your research;
- Receive feedback from your peers in advance;
- Have it indexed in Web of Science (Preprint Citation Index), Google Scholar, Crossref, SHARE, PrePubMed, Scilit and Europe PMC.