Topic Editors
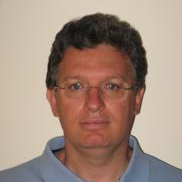
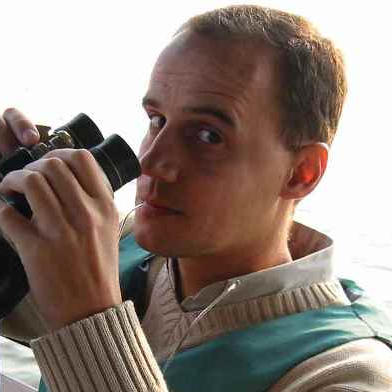

Advances in Crop Simulation Modelling
Topic Information
Dear Colleagues,
In contrast to statistical models, process-based crop simulation models consider dynamic interactions between environment, genotype, and management (including but not limited to agricultural water) factors, something that justifies their application in decision making, in the assessment of the impacts of climate change/variability, and management practices on productivity and environmental performance of alternative cropping systems, to promote better and sustainable agriculture. However, application of these models is often hindered by limited input data availability (such as climate, cultivar and soil characteristics, and management practices) for model calibration and testing and extensive computing time.
Although it is well recognized that the choice of model calibration strategy and incomplete/ poor in quality/not easily accessible input data have implications on the overall reliability of the crop model simulations, only few attempts have been made to quantify errors in crop simulation results related to the above-mentioned issues on a variety of spatial scales (from field to large area applications).
In this context, this Topic aims to highlight the challenges of producing locally relevant and climate informed results from crop simulation models, promoting this way their effective use, across various time frames (from seasonal to future climate change) for agriculture, under historical and future climate conditions.
Dr. Mavromatis Theodoros
Dr. Thomas Alexandridis
Dr. Vassilis Aschonitis
Topic Editors
Keywords
- crop simulation models
- calibration strategies
- input availability
- gridded data
- climate models
- cultivar and soil characteristics
- uncertainty assessment
- climate change scenarios
- remote sensing data assimilation
Participating Journals
Journal Name | Impact Factor | CiteScore | Launched Year | First Decision (median) | APC | |
---|---|---|---|---|---|---|
![]()
Agronomy
|
3.3 | 6.2 | 2011 | 15.5 Days | CHF 2600 | Submit |
![]()
Climate
|
3.0 | 5.5 | 2013 | 21.9 Days | CHF 1800 | Submit |
![]()
Earth
|
2.1 | 3.3 | 2020 | 21.7 Days | CHF 1200 | Submit |
![]()
Remote Sensing
|
4.2 | 8.3 | 2009 | 24.7 Days | CHF 2700 | Submit |
![]()
Water
|
3.0 | 5.8 | 2009 | 16.5 Days | CHF 2600 | Submit |
MDPI Topics is cooperating with Preprints.org and has built a direct connection between MDPI journals and Preprints.org. Authors are encouraged to enjoy the benefits by posting a preprint at Preprints.org prior to publication:
- Immediately share your ideas ahead of publication and establish your research priority;
- Protect your idea from being stolen with this time-stamped preprint article;
- Enhance the exposure and impact of your research;
- Receive feedback from your peers in advance;
- Have it indexed in Web of Science (Preprint Citation Index), Google Scholar, Crossref, SHARE, PrePubMed, Scilit and Europe PMC.