Topic Menu
► Topic MenuTopic Editors


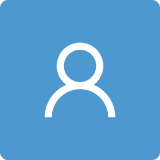
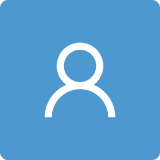

Artificial Intelligence and Computational Methods: Modeling, Simulations and Optimization of Complex Systems
Topic Information
Dear Colleagues,
Due to the increasing computational capability of current data processing systems, new opportunities emerge in the modeling, simulations, and optimization of complex systems and devices. Methods that are difficult to apply, highly demanding, and time-consuming may now be considered when developing complete and sophisticated models in many areas of science and technology. The combination of computational methods and AI algorithms allows conducting multi-threaded analyses to solve advanced and interdisciplinary problems. This article collection aims to bring together research on advances in modeling, simulations, and optimization issues of complex systems. Original research, as well as review articles and short communications, with a particular focus on (but not limited to) artificial intelligence and other computational methods, are welcomed.
Prof. Dr. Jaroslaw Krzywanski
Dr. Yunfei Gao
Dr. Marcin Sosnowski
Dr. Karolina Grabowska
Dr. Dorian Skrobek
Dr. Ghulam Moeen Uddin
Dr. Anna Kulakowska
Dr. Anna Zylka
Dr. Bachil El Fil
Topic Editors
Keywords
- artificial intelligence
- machine learning
- artificial neural networks
- deep learning
- genetic and evolutionary algorithms
- artificial immune systems
- fuzzy logic
- expert systems
- bio-inspired methods
- CFD
- modeling
- simulation
- optimization
- complex systems
Participating Journals
Journal Name | Impact Factor | CiteScore | Launched Year | First Decision (median) | APC |
---|---|---|---|---|---|
![]()
Entropy
|
2.7 | 4.7 | 1999 | 20.8 Days | CHF 2600 |
![]()
Algorithms
|
2.3 | 3.7 | 2008 | 15 Days | CHF 1600 |
![]()
Computation
|
2.2 | 3.3 | 2013 | 18 Days | CHF 1800 |
![]()
Machine Learning and Knowledge Extraction
|
3.9 | 8.5 | 2019 | 19.9 Days | CHF 1800 |
![]()
Energies
|
3.2 | 5.5 | 2008 | 16.1 Days | CHF 2600 |
![]()
Materials
|
3.4 | 5.2 | 2008 | 13.9 Days | CHF 2600 |
MDPI Topics is cooperating with Preprints.org and has built a direct connection between MDPI journals and Preprints.org. Authors are encouraged to enjoy the benefits by posting a preprint at Preprints.org prior to publication:
- Immediately share your ideas ahead of publication and establish your research priority;
- Protect your idea from being stolen with this time-stamped preprint article;
- Enhance the exposure and impact of your research;
- Receive feedback from your peers in advance;
- Have it indexed in Web of Science (Preprint Citation Index), Google Scholar, Crossref, SHARE, PrePubMed, Scilit and Europe PMC.