Topic Editors
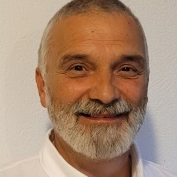
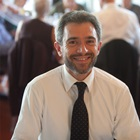
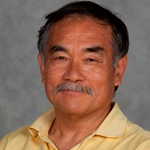
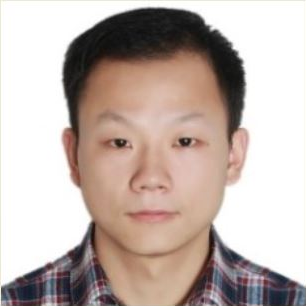
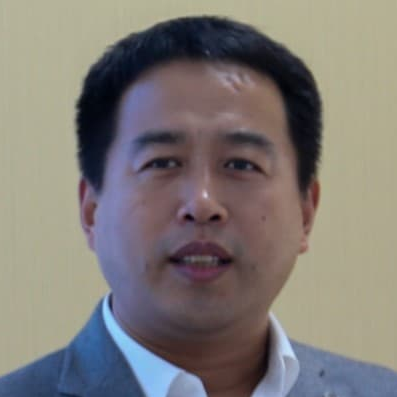
Recent Advances in Structural Health Monitoring, 2nd Volume
Topic Information
Dear Colleagues,
Following the great success of the Topic Proposal "Recent Advances in Structural Health Monitoring", which was closed on March 31, 2023, and in which 72 papers were published, we have decided to launch a second edition, which we hope will be as successful and provide as much insight as the first.
All the theoretical and technological aspects of structural control and health monitoring theory on materials and structures are covered within the concept of Structural Health Monitoring (SHM).
There are currently a number of highly effective, non-destructive evaluation tools available for SHM monitoring. Nondestructive testing (NDT) refers to a group of non-invasive inspection procedures that are used to assess material qualities, components, and complete process units. Damage mechanisms can also be detected, characterized, and measured using these techniques.
The emphasis of this Topical Collection is the crucial field of damage identification and maintenance of modern and historical buildings, as well as for aerospace and mechanical engineering structures and civil infrastructure.
Original contributions using analytical, numerical, and experimental methods are sought in the main areas of monitoring and control of materials and structures.
Topics will include the more classic areas of monitoring, such as data acquisition, signal processing, and sensor technology, by using acoustic emission damage detection or vibration-based identification methods. Furthermore, in the field of mechanics, passive, active, and semi-active schemes and implementations to perform systems control diagnostics are also desired topics.
Other areas of great interest are those of remote data analysis methodologies, such as wireless communications, control of monitoring systems, sensor–logger combinations for mobile applications, and those on multifunctional materials and structures or artificial intelligence tools.
Among others, the methodologies that involve the use of embedded N/MEMS sensors for local damage detection, corrosion sensors, optical fiber sensors, sonic–ultrasonic tests, digital image correlation, tomography techniques, Raman and terahertz spectroscopy, and electromagnetic analysis, which allow for the evaluation of the level of structural damage and its evolution over time, will be incorporated in this Topical Collection.
As a result, the aim of this new initiative is to bring together researchers working in the field of NDT-SHM, both at the material and structure scales. It is our desire to provide novel insights into the application of NDT to a wide variety of materials and structures in the fields of Civil Engineering and Architecture as well as in Mechanical Engineering.
Prof. Dr. Giuseppe Lacidogna
Dr. Alessandro Grazzini
Dr. Gianfranco Piana
Prof. Dr. Sanichiro Yoshida
Dr. Guang-Liang Feng
Prof. Dr. Jie Xu
Topic Editors
Keywords
- structural health monitoring
- damage evaluation
- cracking evolution
- acoustic emission
- sensors
- structural stability
- vibrations
- dynamic control
- optical fibre
- digital image correlation
- sonic–ultrasonic test
- tomography techniques
- impact test
- radar test
- electromagnetic analysis
Participating Journals
Journal Name | Impact Factor | CiteScore | Launched Year | First Decision (median) | APC | |
---|---|---|---|---|---|---|
![]()
Applied Sciences
|
2.5 | 5.3 | 2011 | 17.8 Days | CHF 2400 | Submit |
![]()
CivilEng
|
- | 2.8 | 2020 | 35.5 Days | CHF 1200 | Submit |
![]()
Materials
|
3.1 | 5.8 | 2008 | 15.5 Days | CHF 2600 | Submit |
![]()
Remote Sensing
|
4.2 | 8.3 | 2009 | 24.7 Days | CHF 2700 | Submit |
![]()
Sensors
|
3.4 | 7.3 | 2001 | 16.8 Days | CHF 2600 | Submit |
MDPI Topics is cooperating with Preprints.org and has built a direct connection between MDPI journals and Preprints.org. Authors are encouraged to enjoy the benefits by posting a preprint at Preprints.org prior to publication:
- Immediately share your ideas ahead of publication and establish your research priority;
- Protect your idea from being stolen with this time-stamped preprint article;
- Enhance the exposure and impact of your research;
- Receive feedback from your peers in advance;
- Have it indexed in Web of Science (Preprint Citation Index), Google Scholar, Crossref, SHARE, PrePubMed, Scilit and Europe PMC.