Topic Editors




2. Department of Soil and Crop Sciences, Texas A&M University, TAMU 2124, College Station, TX 77843, USA

Advances in Smart Agriculture with Remote Sensing as the Core and Its Applications in Crops Field
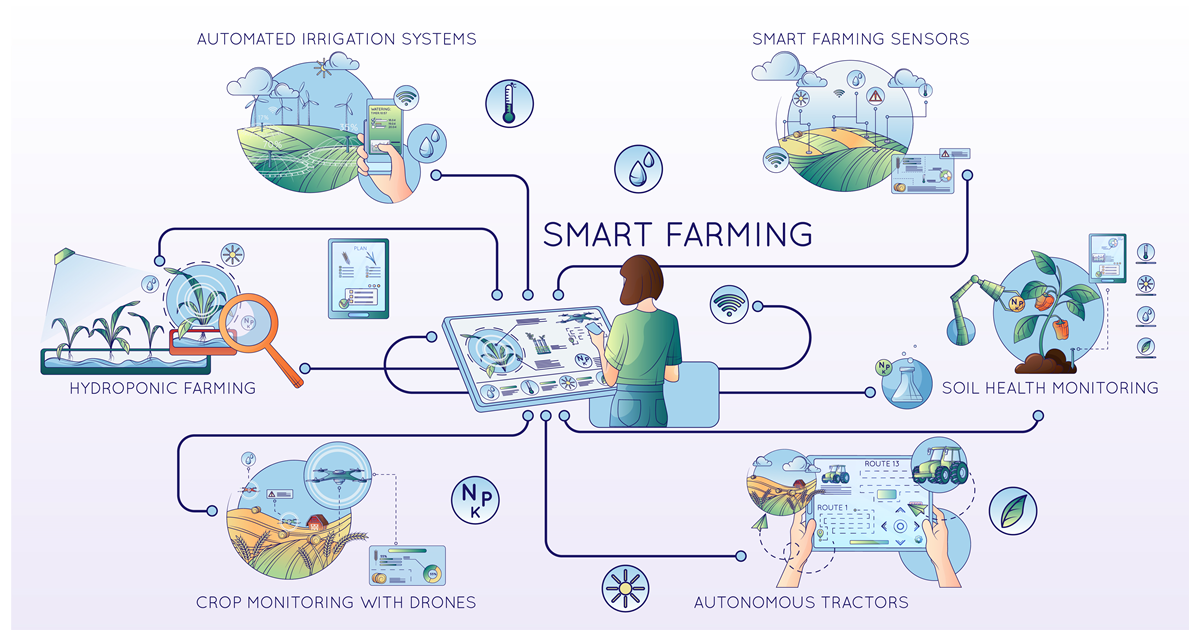
Topic Information
Dear Colleagues,
In recent years, smart agriculture with remote sensing and modeling technologies has brought significant benefits in crop fields and has also altered our understanding and management of crops. Remote sensing allows for crop growth monitoring on different scales such as “ground–low altitude–satellite”, while crop modeling provides predictive insights into crop growth and yield based on a diverse set of environmental parameters. Remote sensing and modeling are fully integrated into applications of crop growth, nutrition demands, irrigation management, and pest control in smart agriculture to optimize agricultural practices, enhance resource efficiency, and make substantial contributions to sustainable agricultural development. This research topic aims to seamlessly integrate remote sensing and modeling, essential components in smart agriculture, to address urgent challenges such as optimizing resource utilization and sustainable agricultural development with enhanced crop production.
The scope of this research topic encompasses a broad range of subjects including but not limited to:
- Integrating remote sensing data with plant traits into crop models to enhance prediction accuracy and decision support.
- Applying machine learning and AI algorithms in crop modeling for increased accuracy and adaptability.
- Utilizing the Internet of Things, sensors, and drones for real-time data collection and monitoring in smart agriculture.
We invite authors to contribute original research articles, perspectives, and reviews, providing valuable insights into the ”Advances in Smart Agriculture with Remote Sensing as the Core and Its Applications in Crops Field”.
Dr. Syed Tahir Ata-Ul-Karim
Dr. Yang Liu
Dr. Ben Zhao
Dr. Wenxuan Guo
Dr. Lei Zhang
Topic Editors
Keywords
- crop
- remote sensing
- crop modeling
- smart agriculture
- machine learning
Participating Journals
Journal Name | Impact Factor | CiteScore | Launched Year | First Decision (median) | APC | |
---|---|---|---|---|---|---|
![]()
Remote Sensing
|
4.2 | 8.3 | 2009 | 23.9 Days | CHF 2700 | Submit |
![]()
Agronomy
|
3.3 | 6.2 | 2011 | 17.6 Days | CHF 2600 | Submit |
![]()
Agriculture
|
3.3 | 4.9 | 2011 | 19.2 Days | CHF 2600 | Submit |
![]()
Crops
|
- | - | 2021 | 22.1 Days | CHF 1000 | Submit |
![]()
Plants
|
4.0 | 6.5 | 2012 | 18.9 Days | CHF 2700 | Submit |
Preprints.org is a multidisciplinary platform offering a preprint service designed to facilitate the early sharing of your research. It supports and empowers your research journey from the very beginning.
MDPI Topics is collaborating with Preprints.org and has established a direct connection between MDPI journals and the platform. Authors are encouraged to take advantage of this opportunity by posting their preprints at Preprints.org prior to publication:
- Share your research immediately: disseminate your ideas prior to publication and establish priority for your work.
- Safeguard your intellectual contribution: Protect your ideas with a time-stamped preprint that serves as proof of your research timeline.
- Boost visibility and impact: Increase the reach and influence of your research by making it accessible to a global audience.
- Gain early feedback: Receive valuable input and insights from peers before submitting to a journal.
- Ensure broad indexing: Web of Science (Preprint Citation Index), Google Scholar, Crossref, SHARE, PrePubMed, Scilit and Europe PMC.