Journal Description
Fire
Fire
is an international, peer-reviewed, open access journal about the science, policy, and technology of fires and how they interact with communities and the environment, published monthly online by MDPI. The Global Wildland Fire Network is affiliated with Fire.
- Open Access— free for readers, with article processing charges (APC) paid by authors or their institutions.
- High Visibility: indexed within Scopus, SCIE (Web of Science), AGRIS, PubAg, and other databases.
- Journal Rank: JCR - Q1 (Forestry) / CiteScore - Q2 (Forestry)
- Rapid Publication: manuscripts are peer-reviewed and a first decision is provided to authors approximately 15 days after submission; acceptance to publication is undertaken in 2.8 days (median values for papers published in this journal in the second half of 2023).
- Recognition of Reviewers: reviewers who provide timely, thorough peer-review reports receive vouchers entitling them to a discount on the APC of their next publication in any MDPI journal, in appreciation of the work done.
- Paper Types: in addition to regular articles we accept Perspectives, Case Studies, Data Descriptors, Technical Notes, and Monographs.
Impact Factor:
3.2 (2022);
5-Year Impact Factor:
4.0 (2022)
Latest Articles
Experimental Evaluation of Methanol/Jet-A Blends as Sustainable Aviation Fuels for Turbo-Engines: Performance and Environmental Impact Analysis
Fire 2024, 7(5), 155; https://doi.org/10.3390/fire7050155 - 26 Apr 2024
Abstract
This study offers a comprehensive examination, both theoretically and experimentally, of the potential of methanol (M) as a sustainable aviation fuel (SAF) assessed in combination with kerosene (Ke—Jet-A aviation fuel + 5% Aeroshell oil). Different blends of methanol and kerosene (10%, 20%, and
[...] Read more.
This study offers a comprehensive examination, both theoretically and experimentally, of the potential of methanol (M) as a sustainable aviation fuel (SAF) assessed in combination with kerosene (Ke—Jet-A aviation fuel + 5% Aeroshell oil). Different blends of methanol and kerosene (10%, 20%, and 30% vol. of (M) was added to Ke) were tested in an aviation micro turbo-engine under various operating regimes, such as idle, cruise, and maximum. Key engine parameters, including combustion temperature, fuel consumption, and thrust, were closely monitored during these trials. Essential performance indicators such as combustion efficiency, thermal efficiency, and specific consumption for all fuel blends under maximum operating conditions are also presented. Physical and chemical characteristics, such as viscosity, density, calorific value and flash point, were determined for each blend. Moreover, elemental analysis and FTIR spectroscopy were utilized to evaluate the chemical composition of the fuels. This study further investigated the air requirements for stoichiometric combustion and computed the resulting CO2 and H2O emissions. Experimental tests were conducted on the Jet Cat P80® micro turbo-engine, covering assessments of starting procedures, acceleration, deceleration, and pollutant emissions (CO and SO2) during various engine operating conditions. The results suggest that the examined fuel blends demonstrate stable engine performance at concentrations of 10% and 20% methanol. However, observations indicate that with an increase in methanol concentration, particularly at 30%, the stability of the engine at idle and, notably, at maximum speed decreases significantly. Specifically, at a 30% methanol concentration, the engine no longer operates stably, exhibiting significant rpm fluctuations, leading to the decision not to explore higher concentrations.
Full article
(This article belongs to the Special Issue Jet Fuel Combustion)
Open AccessArticle
Integrating Real-Time Meteorological Conditions into a Novel Fire Spread Model for Grasslands
by
Yakun Zhang, Huimin Yu, Wenjiang Huang, Tiecheng Huang, Meng Fan and Kun Wang
Fire 2024, 7(5), 154; https://doi.org/10.3390/fire7050154 - 26 Apr 2024
Abstract
Accurate comprehension of grassland fires is imperative for maintaining ecological stability. In this study, we propose a novel fire model that incorporates real-time meteorological conditions. Our methodology integrates key meteorological factors including relative humidity, temperature, degree of solidification of combustible materials, and wind
[...] Read more.
Accurate comprehension of grassland fires is imperative for maintaining ecological stability. In this study, we propose a novel fire model that incorporates real-time meteorological conditions. Our methodology integrates key meteorological factors including relative humidity, temperature, degree of solidification of combustible materials, and wind speed. These factors are embedded into a comprehensive function that determines both the downwind and upwind spreading speeds of the fire. Additionally, the model accommodates fire spread in the absence of wind by incorporating the direction perpendicular to the wind, with wind speed set to zero. By precisely determining wind speed, the model enables real-time calculation of fire spread speeds in all directions. Under stable wind conditions, the fire spread area typically adopts an elliptical shape. Leveraging ellipse properties, we define the aspect ratio as a function related to wind speed. Consequently, with knowledge of the fire duration, the model accurately estimates the area of fire spread. Our findings demonstrate the effectiveness of this model in predicting and evaluating fires in the Hulunbuir Grassland. The model offers an innovative method for quantifying grassland fires, contributing significantly to the understanding and management of grassland ecosystems.
Full article
(This article belongs to the Special Issue Fire Numerical Simulation)
►▼
Show Figures
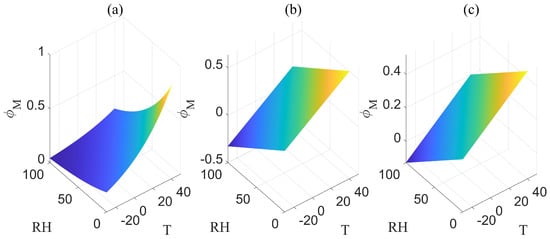
Figure 1
Open AccessArticle
Predicting Wildfire Ember Hot-Spots on Gable Roofs via Deep Learning
by
Mohammad Khaled Al-Bashiti, Dac Nguyen, M. Z. Naser and Nigel B. Kaye
Fire 2024, 7(5), 153; https://doi.org/10.3390/fire7050153 - 25 Apr 2024
Abstract
Ember accumulation on and around homes can lead to spot fires and home ignition. Post wildland fire assessments suggest that this mechanism is one of the leading causes of home destruction in wildland urban interface (WUI) fires. However, the process of ember deposition
[...] Read more.
Ember accumulation on and around homes can lead to spot fires and home ignition. Post wildland fire assessments suggest that this mechanism is one of the leading causes of home destruction in wildland urban interface (WUI) fires. However, the process of ember deposition and accumulation on and around houses remains poorly understood. Herein, we develop a deep learning (DL) model to analyze data from a series of ember-related wind tunnel experiments for a range of wind conditions and roof slopes. The developed model is designed to identify building roof regions where embers will remain in contact with the rooftop. Our results show that the DL model is capable of accurately predicting the position and fraction of the roof on which embers remain in place as a function of the wind speed, wind direction, roof slope, and location on the windward and leeward faces of the rooftop. The DL model was augmented with explainable AI (XAI) measures to examine the extent of the influence of these parameters on the rooftop ember coverage and potential ignition.
Full article
(This article belongs to the Special Issue Advanced Approaches to Wildfire Detection, Monitoring and Surveillance)
►▼
Show Figures
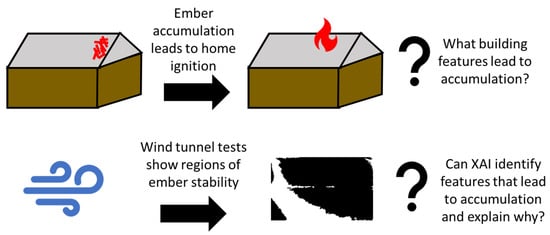
Figure 1
Open AccessArticle
Evaluating the Ceiling Gas Temperature in a Branched Tunnel Fire with a Sloped Mainline Region under Natural Ventilation
by
Ning Lu, Xiaolin Yao, Jinming Yang and Youbo Huang
Fire 2024, 7(5), 152; https://doi.org/10.3390/fire7050152 - 24 Apr 2024
Abstract
The effect of the mainline slope on the ceiling temperature profile in a branched tunnel has not been clarified nor included in existing models. Thus, in this paper, the numerical code was employed to investigate the induced airflow velocity and gas temperature beneath
[...] Read more.
The effect of the mainline slope on the ceiling temperature profile in a branched tunnel has not been clarified nor included in existing models. Thus, in this paper, the numerical code was employed to investigate the induced airflow velocity and gas temperature beneath the ceiling in a branch tunnel with a sloped upstream mainline. The mainline slope varied from 1% to 7%, with an interval of 1%. Five fire power of 3 MW, 5 MW, 10 MW, 15 MW, and 20 MW are employed on each slope. The airflow velocity and the longitudinal temperature in the mainline tunnel are measured and analyzed. Results show that the stack effect obviously occurred, which caused longitudinal velocity to prevent the smoke reverse flow in the mainline. The induced airflow velocity in the upstream inclined mainline is higher with increasing slope, and the dimensionless velocity is normalized well by the proposed expression. The maximum ceiling temperature is independent of the mainline slope and correlated well by Q*2/3, but the effect of the mainline slope on temperature longitudinal decay is worth considering. Finally, a normalized expression for longitudinal temperature decay in an inclined mainline is proposed by taking the fire power and mainline slope into account.
Full article
(This article belongs to the Special Issue Advance in Tunnel Fire Research)
Open AccessArticle
Deep Learning-Based Forest Fire Risk Research on Monitoring and Early Warning Algorithms
by
Dongfang Shang, Fan Zhang, Diping Yuan, Le Hong, Haoze Zheng and Fenghao Yang
Fire 2024, 7(4), 151; https://doi.org/10.3390/fire7040151 - 22 Apr 2024
Abstract
With the development of image processing technology and video analysis technology, forest fire monitoring technology based on video recognition is more and more important in the field of forest fire prevention and control. The objects currently applied to forest fire video image monitoring
[...] Read more.
With the development of image processing technology and video analysis technology, forest fire monitoring technology based on video recognition is more and more important in the field of forest fire prevention and control. The objects currently applied to forest fire video image monitoring system monitoring are mainly flames and smoke. This paper proposes a forest fire risk monitoring and early warning algorithm, which integrates a deep learning model, infrared monitoring and early warning, and forest fire weather index. The algorithm first obtains the current visible image and infrared image of the same forest area, utilizing a smoke detection model based on deep learning to detect smoke in the visible image, and obtains the confidence level of the occurrence of fire in said visible image. Then, it determines whether the local temperature value of said infrared image exceeds a preset warning value, and obtains a judgment result based on the infrared image. It calculates again a current FWI based on environmental data, and determines a current fire danger level based on the current FWI. Finally, it determines whether or not to carry out a fire warning based on said fire danger level, said confidence level of the occurrence of fire in said visible image, and said judgment result based on the infrared image. The experimental results show that the accuracy of the algorithm in this paper reaches 94.12%, precision is 96.1%, recall is 93.67, and F1-score is 94.87. The algorithm in this paper can improve the accuracy of smoke identification at the early stage of forest fire danger occurrence, especially by excluding the interference caused by clouds, fog, dust, and so on, thus improving the fire danger warning accuracy.
Full article
(This article belongs to the Special Issue Intelligent Forest Fire Prediction and Detection)
►▼
Show Figures
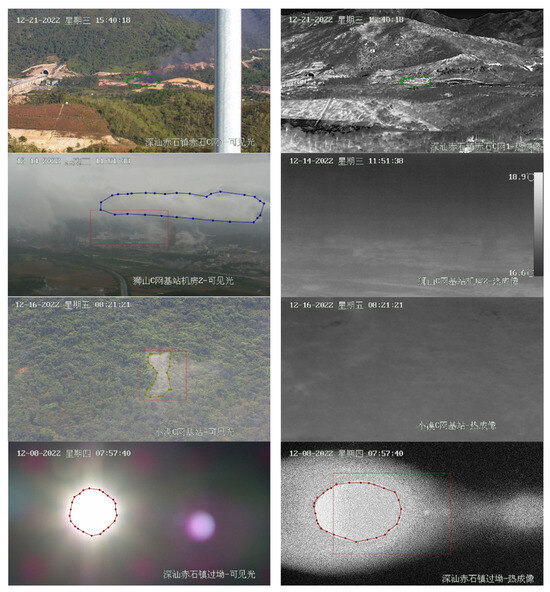
Figure 1
Open AccessArticle
Impact of Seasonal Heating on PM10 and PM2.5 Concentrations in Sučany, Slovakia: A Temporal and Spatial Analysis
by
Dusan Jandacka, Daniela Durcanska, Miriam Nicolanska and Michal Holubcik
Fire 2024, 7(4), 150; https://doi.org/10.3390/fire7040150 - 21 Apr 2024
Abstract
Complying with strict PM10 and PM2.5 limit values poses challenges in many European regions, influenced by diverse factors such as natural, regional, and local anthropogenic sources. Urban air pollution, exacerbated by road transport, local industry, and dust resuspension, contrasts with rural
[...] Read more.
Complying with strict PM10 and PM2.5 limit values poses challenges in many European regions, influenced by diverse factors such as natural, regional, and local anthropogenic sources. Urban air pollution, exacerbated by road transport, local industry, and dust resuspension, contrasts with rural areas affected by solid fuel-based local heating and increasing wood burning. This study focuses on village of Sučany, located in Slovakia, analysing PM concentrations during non-heating and heating seasons. The method of analysis relies on the use of the MP101M air quality analyser that utilises beta radiation absorption method. One set of measurements was conducted at five distinct locations during the heating season (18/01/2019 to 28/02/2019) and non-heating season (14/08/2018 to 1/10/2018). Significant differences emerged during the non-heating season with corresponding PM10 averages of 23.0 µg/m3 and PM2.5 at 19.3 µg/m3. In contrast, the PM10 averaged 53.9 µg/m3 and 52.8 µg/m3 during the heating season. The heating season shows PM2.5 contributing up to 98% of total PM10. The distribution of PM10 and PM2.5 pollution and the location of the potential source obtained using polar plots differed during the heating and non-heating seasons. This research underscores the impact of local heating on air quality in a typical Slovak village. The key recommendation for targeted interventions is supporting up-to-date air quality data, education, and financial incentives for citizens in order to implement cleaner and modern heating solutions.
Full article
(This article belongs to the Special Issue Solid Fuels – Analysis, Burning and Emissions)
►▼
Show Figures
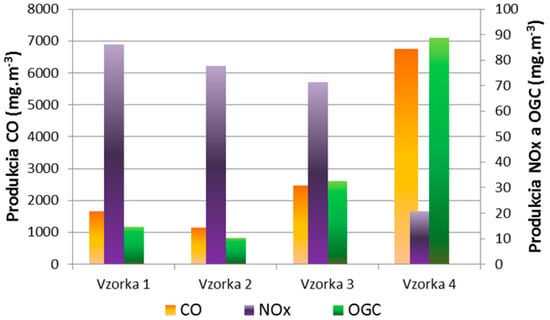
Figure 1
Open AccessArticle
A New Method for the Determination of Fire Risk Zones in High-Bay Warehouses
by
Goran Bošković, Marko Todorović, Dejan Ubavin, Borivoj Stepanov, Višnja Mihajlović, Marija Perović and Zoran Čepić
Fire 2024, 7(4), 149; https://doi.org/10.3390/fire7040149 - 21 Apr 2024
Abstract
Considering that the determination of fire hazard zones in warehouses is not sufficiently researched and studied, this paper aims to present a new methodological approach concerning the mentioned issue. Based on the COPRAS multi-criteria decision-making method, a new method was developed for the
[...] Read more.
Considering that the determination of fire hazard zones in warehouses is not sufficiently researched and studied, this paper aims to present a new methodological approach concerning the mentioned issue. Based on the COPRAS multi-criteria decision-making method, a new method was developed for the precise determination of potential zones where there is a risk of fire. The advantage of the described method is that it allows the quick and easy determination of all-orientation fire risk zones. The method requires fewer hardware resources compared to the existing ones and enables the display of the warehouse space in the form of a 3D model with calculated fire hazard zones. The mentioned procedure represents the first step when planning the layout and arrangement in the warehouse itself. The effectiveness of the proposed method was confirmed through a suitable numerical example.
Full article
(This article belongs to the Special Issue Fire Safety Management and Risk Assessment)
►▼
Show Figures
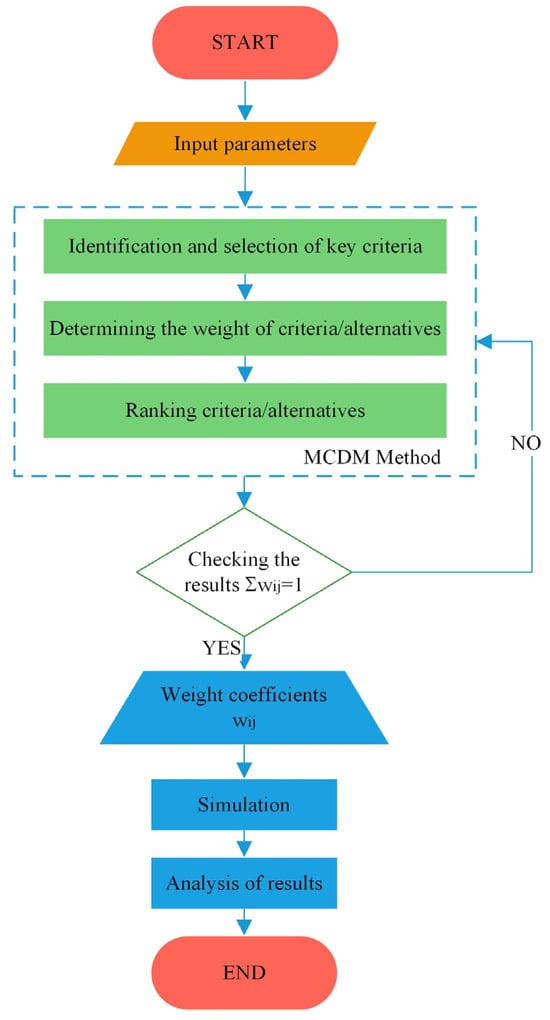
Figure 1
Open AccessArticle
Short-Interval, High-Severity Wildfire Depletes Diversity of Both Extant Vegetation and Soil Seed Banks in Fire-Tolerant Eucalypt Forests
by
Sabine Kasel, Thomas A. Fairman and Craig R. Nitschke
Fire 2024, 7(4), 148; https://doi.org/10.3390/fire7040148 - 19 Apr 2024
Abstract
Many plant species are well-adapted to historical fire regimes. An increase in the severity, frequency, and extent of wildfires could compromise the regenerative capacity of species, resulting in permanent shifts in plant diversity. We surveyed extant vegetation and soil seed banks across two
[...] Read more.
Many plant species are well-adapted to historical fire regimes. An increase in the severity, frequency, and extent of wildfires could compromise the regenerative capacity of species, resulting in permanent shifts in plant diversity. We surveyed extant vegetation and soil seed banks across two forest types with contrasting historical fire regimes—Shrubby Dry Forest (fire return interval: 10–20 years) and Sub-Alpine Woodland (50–100 years). Over the past 20 years, both forests have been subject to repeated, high-severity wildfires at intervals significantly shorter than their historical return intervals. We examined the soil seed bank response to fire-cued germination, and whether the plant diversity in soil seed banks and extant vegetation demonstrated similar responses to short-interval, high-severity wildfires. The soil seed bank demonstrated a positive response to heat in combination with smoke, and for the Sub-Alpine Woodland, this was limited to sites more frequently burnt by fire. With an increase in fire frequency, there was a decline in species richness and Shannon’s Diversity and a shift in species composition in both extant vegetation and the soil seed bank. The fire frequency effects on the relative richness of trait associations were restricted to the Shrubby Dry Forest, and included an increase in short-lived obligate seeders, wind-dispersed species, and ant-dispersed shrubs in burnt relative to long unburnt sites in both extant vegetation and the soil seed bank. Graminoids were the most abundant component of the soil seed banks of Sub-Alpine Woodlands, and this increased with more frequent fire, with a similar trend (p = 0.06) in extant vegetation. Clear shifts in plant diversity in both soil seed banks and extant vegetation in forest types with contrasting historical fire regimes suggest that emerging fire regimes are pushing ecosystems beyond their historical range of variability, including potentially more flammable states and a decline in the buffering capacity of soil seed banks.
Full article
(This article belongs to the Special Issue Effects of Fires on Forest Ecosystems)
►▼
Show Figures
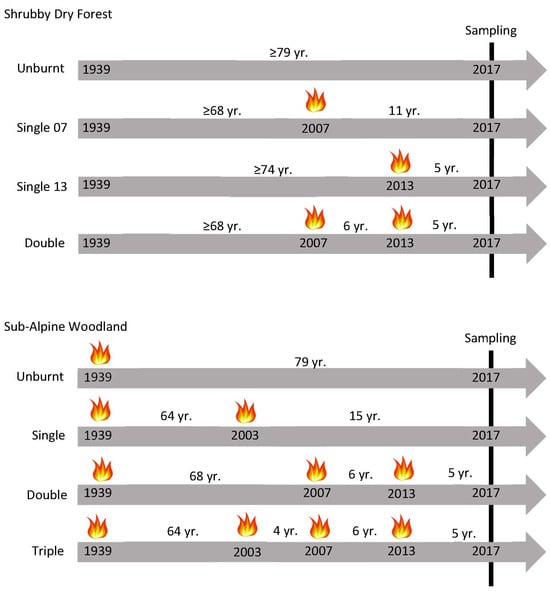
Figure 1
Open AccessArticle
Simulation of Damage Caused by Oil Fire in Cable Passage to Tunnel Cable
by
Feng Liu, Jiaqing Zhang, Mengfei Gu, Yushun Liu, Tao Sun and Liangpeng Ye
Fire 2024, 7(4), 147; https://doi.org/10.3390/fire7040147 - 19 Apr 2024
Abstract
In order to evaluate the damage to tunnel cables caused by fire caused by leakage of transformer oil into a cable channel, the fire characteristics of different volumes of transformer oil flowing into a cable channel were analyzed by numerical simulation. The results
[...] Read more.
In order to evaluate the damage to tunnel cables caused by fire caused by leakage of transformer oil into a cable channel, the fire characteristics of different volumes of transformer oil flowing into a cable channel were analyzed by numerical simulation. The results show that when the total leakage of transformer oil is less than or equal to 3 L, the fire will end within 120 s, and when the total leakage is greater than or equal to 5 L, the fire duration will exceed 900 s. When the leakage amount is 1 L, the cable only burns slightly, and when the leakage amount is 3~12 L, the cable burns obviously. The combustion of the cable is mainly concentrated between 15 s and 75 s, and the overall combustion rate of the cable increases first and then decreases. When the total leakage is greater than or equal to 8 L, the damage distance of the middle and lower layer cable is the smallest. When the total leakage is less than or equal to 5 L, the damage distance of the lower layer cable is the smallest, and the damage distance of the lower layer cable, middle and lower layer cable, and middle and upper layer cable is less than half of the length of the cable channel.
Full article
(This article belongs to the Special Issue Cable and Electrical Fires)
►▼
Show Figures
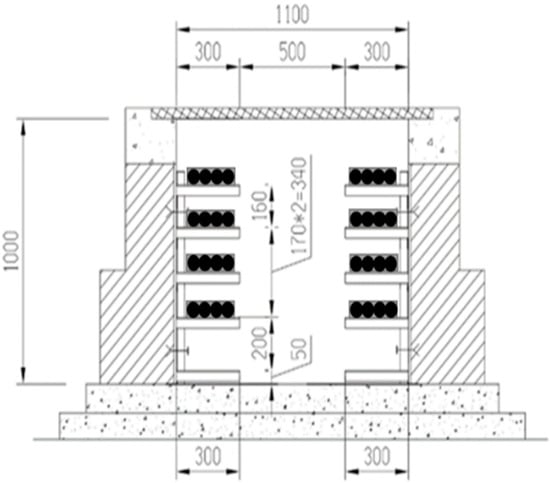
Figure 1
Open AccessArticle
Study on Flowability Enhancement and Performance Testing of Ultrafine Dry Powder Fire Extinguishing Agents Based on Application Requirements
by
Guangbin Lu, Junchao Zhao, Yanting Zhou, Yangyang Fu, Song Lu and Heping Zhang
Fire 2024, 7(4), 146; https://doi.org/10.3390/fire7040146 - 18 Apr 2024
Abstract
Flowability greatly affects the application of ultrafine dry powder fire extinguishing systems, while hydrophobicity and acute inhalation toxicity are concerns for fire extinguishing agents. In the present study, we examined the impact of hydrophobic fumed silica on the hydrophobicity and flow properties of
[...] Read more.
Flowability greatly affects the application of ultrafine dry powder fire extinguishing systems, while hydrophobicity and acute inhalation toxicity are concerns for fire extinguishing agents. In the present study, we examined the impact of hydrophobic fumed silica on the hydrophobicity and flow properties of ammonium dihydrogen phosphate as the base. Our findings revealed that incorporating 6 wt.% of hydrophobic fumed silica resulted in optimal flowability, accompanied by a hydrophobicity angle of 126.48°. The excessive inclusion of hydrophobic fumed silica impeded powder flow within the ammonium dihydrogen phosphate particles. Furthermore, the investigations indicated that the incorporation of a small quantity of bentonite (0.5 wt.%) amongst the three functional additives—bentonite, magnesium stearate, and perlite—offered further enhancements in powder flowability. In fire extinguishing experiments’ total flooding conditions (1 m3), the designed UDPA exhibited a minimum required extinguishing concentration of merely 41.5 g/m3, which is better than the publicly reported value. Moreover, observations on the well-being of mice subjected to nearly three times the extinguishing concentration at 60 s, 10 min, and 3 days, respectively, demonstrated the absence of acute inhalation toxicity associated with the designed UDPA. Collectively, the developed ultrafine dry powder fire extinguishing agent displayed promising performance and possesses broad applicability.
Full article
(This article belongs to the Special Issue Advances in New Energy Materials and Fire Safety)
►▼
Show Figures
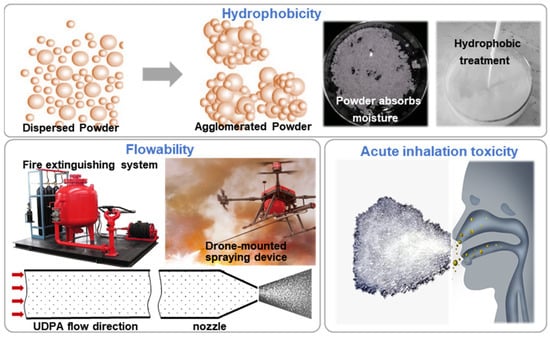
Figure 1
Open AccessArticle
Characterization of Wildland Fuels Based on Topography and Forest Attributes in North-Central Appalachia
by
Ziyu Dong and Roger A. Williams
Fire 2024, 7(4), 145; https://doi.org/10.3390/fire7040145 - 17 Apr 2024
Abstract
►▼
Show Figures
Forest ecosystem attributes and their spatial variation across the landscape have the potential to subsequently influence variations in fire behavior. Understanding this variation is critical to fire managers in their ability to predict fire behavior and rate of spread. However, a fine-scale description
[...] Read more.
Forest ecosystem attributes and their spatial variation across the landscape have the potential to subsequently influence variations in fire behavior. Understanding this variation is critical to fire managers in their ability to predict fire behavior and rate of spread. However, a fine-scale description of fuel patterns and their relationship with overstory and understory attributes for north-central Appalachia is lacking due to the complicated quantification of variations in topography, forest attributes, and their interactions. To better understand the fire environment in north-central Appalachia and provide a comprehensive evaluation based on fine-scale topography, ninety-four plots were established across different aspects and slope positions within an oak–hickory forest located in southeast Ohio, USA, which historically fell within fire regime group I with a fire return interval ranging from 7 to 26 years. The data collected from these plots were analyzed by four components of the fire environment, which include the overstory, understory, shrub and herbaceous layers, surface fuels, and fuel conditions. The results reveal that fuel bed composition changed across aspects and slope position, and it is a primary factor that influences the environment where fire occurs. Specifically, the oak fuel load was highest on south-facing slopes and in upper slope positions, while maple fuel loads were similar across all aspects and slope positions. Oak and maple basal areas were the most significant factors in predicting the oak and maple fuel load, respectively. In the shrub and undergrowth layers, woody plant coverage was higher in upper slope positions compared to lower slope positions. Overstory canopy closure displayed a significant negative correlation with understory trees/ha and woody plant variables. The findings in this study can provide a better understanding of fine-scale fuel bed and vegetation characteristics, which can subsequently feed into fire behavior modeling research in north-central Appalachia based on the different characterizations of the fire environment by landscape position.
Full article
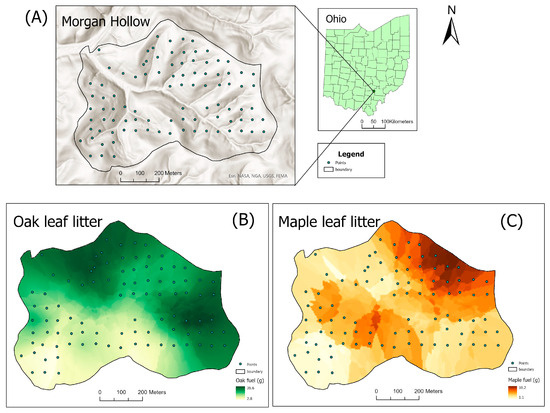
Figure 1
Open AccessArticle
Anticipating Future Risks of Climate-Driven Wildfires in Boreal Forests
by
Shelby Corning, Andrey Krasovskiy, Pavel Kiparisov, Johanna San Pedro, Camila Maciel Viana and Florian Kraxner
Fire 2024, 7(4), 144; https://doi.org/10.3390/fire7040144 - 17 Apr 2024
Abstract
Extreme forest fires have historically been a significant concern in Canada, the Russian Federation, the USA, and now pose an increasing threat in boreal Europe. This paper deals with application of the wildFire cLimate impacts and Adaptation Model (FLAM) in boreal forests. FLAM
[...] Read more.
Extreme forest fires have historically been a significant concern in Canada, the Russian Federation, the USA, and now pose an increasing threat in boreal Europe. This paper deals with application of the wildFire cLimate impacts and Adaptation Model (FLAM) in boreal forests. FLAM operates on a daily time step and utilizes mechanistic algorithms to quantify the impact of climate, human activities, and fuel availability on wildfire probabilities, frequencies, and burned areas. In our paper, we calibrate the model using historical remote sensing data and explore future projections of burned areas under different climate change scenarios. The study consists of the following steps: (i) analysis of the historical burned areas over 2001–2020; (ii) analysis of temperature and precipitation changes in the future projections as compared to the historical period; (iii) analysis of the future burned areas projected by FLAM and driven by climate change scenarios until the year 2100; (iv) simulation of adaptation options under the worst-case scenario. The modeling results show an increase in burned areas under all Representative Concentration Pathway (RCP) scenarios. Maintaining current temperatures (RCP 2.6) will still result in an increase in burned area (total and forest), but in the worst-case scenario (RCP 8.5), projected burned forest area will more than triple by 2100. Based on FLAM calibration, we identify hotspots for wildland fires in the boreal forest and suggest adaptation options such as increasing suppression efficiency at the hotspots. We model two scenarios of improved reaction times—stopping a fire within 4 days and within 24 h—which could reduce average burned forest areas by 48.6% and 79.2%, respectively, compared to projected burned areas without adaptation from 2021–2099.
Full article
(This article belongs to the Special Issue Patterns, Drivers, and Multiscale Impacts of Wildland Fires)
►▼
Show Figures
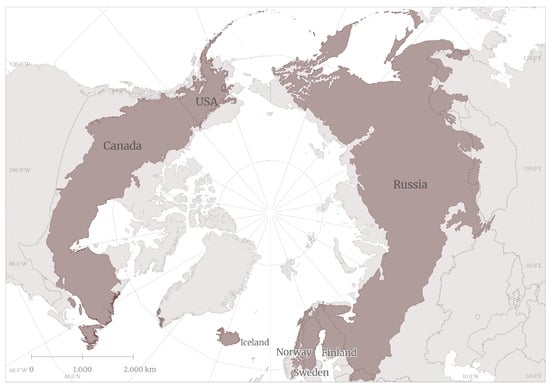
Figure 1
Open AccessArticle
Prediction of DC Breakdown Voltage of Rod–Plate Gaps under Full-Flame Bridging Conditions
by
Ziheng Pu, Yuan Li, Peng Li, Kuan Ye, Kai Zhou and Ruizhe Zhang
Fire 2024, 7(4), 143; https://doi.org/10.3390/fire7040143 - 16 Apr 2024
Abstract
►▼
Show Figures
In order to evaluate the risk of transmission line tripping due to wildfires, it is necessary to predict the breakdown voltage of the insulation gap under the flame. Firstly, this paper studies the breakdown prediction of rod–plane gaps under the full-flame bridging of
[...] Read more.
In order to evaluate the risk of transmission line tripping due to wildfires, it is necessary to predict the breakdown voltage of the insulation gap under the flame. Firstly, this paper studies the breakdown prediction of rod–plane gaps under the full-flame bridging of wooden cribs; it then obtains the breakdown voltage and the leakage current values of full-flame bridging considering different sizes of wooden cribs and different gap distances. Then, a multi-physical field simulation is carried out to obtain the flame gap characteristic parameters, such as spatial temperature. The feature quantity is normalized and reduced in dimension, and a prediction model for gap breakdown voltage under flame conditions based on a support vector machine (SVM) is established. Finally, the DC withstand voltage values and corresponding characteristic quantities under different flame gap conditions are used as sample sets to test the prediction model. The results show that the prediction error for small gap breakdown voltage is less than 2.6%. The samples were tested under different flame intensities for training and prediction, and the error was less than 3.3%. The small gap data for 30~60 cm is used to predict the breakdown voltage of the long gap for 100~140 cm, and the error is less than 3.2%. Compared with the fitting correction formula method proposed in existing research, the error is reduced by 11.5% and 4.4%, respectively, which verifies the effectiveness of the SVM prediction model.
Full article
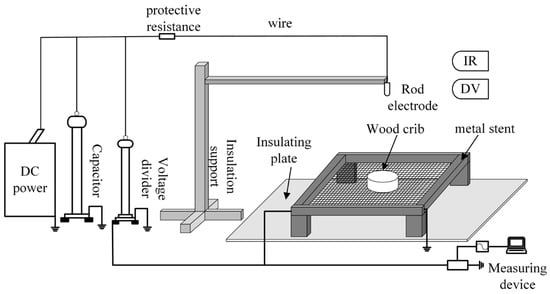
Figure 1
Open AccessArticle
Applicability of an Ionising Radiation Measuring System for Real-Time Effective-Dose-Optimised Route Finding Solution during Nuclear Accidents
by
Attila Zsitnyányi, János Petrányi, Jácint Jónás, Zoltán Garai, Lajos Kátai-Urbán, Iván Zádori and István Kobolka
Fire 2024, 7(4), 142; https://doi.org/10.3390/fire7040142 - 16 Apr 2024
Abstract
The reduction in the effective dose of evacuated injured persons through contaminated areas of nuclear accidents is an essential emergency services requirement. In this context, there appeared a need to develop a dose-optimised route finding method for firefighting rescue vehicles, which includes the
[...] Read more.
The reduction in the effective dose of evacuated injured persons through contaminated areas of nuclear accidents is an essential emergency services requirement. In this context, there appeared a need to develop a dose-optimised route finding method for firefighting rescue vehicles, which includes the development of a real-time decision support measurement and evaluation system. This determines and visualises the radiation exposure of possible routes in a tested area. The system inside and outside of the vehicle measures the ambient dose equivalent rate, the gamma spectra, and also the airborne radioactive aerosol and iodine levels. The method uses gamma radiation measuring NaI(Tl) scintillation detectors mounted on the outside of the vehicle, to determine the dose rate inside the vehicle using the previously recorded attenuation conversation function, while continuously collecting the air through a filter and using an alpha, beta, and gamma radiation measuring NaI(Tl)+ PVT + ZnS(Ag) scintillator to determine the activity concentration in the air, using these measured values to determine the effective dose for all routes and all kinds of vehicles. The energy-dependent shielding effect of the vehicle, the filtering efficiency of the collective protection equipment, and the vehicle’s speed and travel time were taken into account. The results were validated by using gamma point sources with different activity and energy levels. The measurement results under real conditions and available real accident data used in our simulations for three different vehicles and pedestrians proved the applicability of the system. During a nuclear accident based on our model calculations, the inhalation of radioactive aerosols causes a dose almost an order of magnitude higher than the external gamma radiation caused by the fallout contamination. The selection of the appropriate vehicle and its route is determined by the spectrum that can be measured at the accident site but especially by the radioactive aerosol concentration in the air that can be measured in the area. In the case of radiation measuring detectors, the shielding effect of the carrier vehicle must be taken into account, especially in the case of heavy shielding vehicles. The method provides an excellent opportunity to reduce the damage to the health of accident victims and first responders during rescue operations.
Full article
(This article belongs to the Section Mathematical Modelling and Numerical Simulation of Combustion and Fire)
►▼
Show Figures
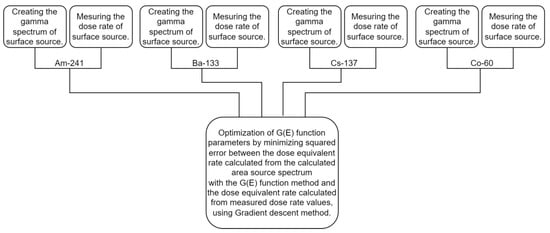
Figure 1
Open AccessArticle
Comparing Accuracy of Wildfire Spread Prediction Models under Different Data Deficiency Conditions
by
Jiahao Zhou, Wenyu Jiang, Fei Wang, Yuming Qiao and Qingxiang Meng
Fire 2024, 7(4), 141; https://doi.org/10.3390/fire7040141 - 16 Apr 2024
Abstract
Wildfire is one of the most severe natural disasters globally, profoundly affecting natural ecology, economy, and health and safety. Precisely predicting the spread of wildfires has become an important research topic. Current fire spread prediction models depend on inputs from a variety of
[...] Read more.
Wildfire is one of the most severe natural disasters globally, profoundly affecting natural ecology, economy, and health and safety. Precisely predicting the spread of wildfires has become an important research topic. Current fire spread prediction models depend on inputs from a variety of geographical and environmental variables. However, unlike the ideal conditions simulated in the laboratory, data gaps often occur in real wildfire scenarios, posing challenges to the accuracy and robustness of predictions. It is necessary to explore the extent to which different missing items affect prediction accuracy, thereby providing rational suggestions for emergency decision-making. In this paper, we tested how different conditions of missing data affect the prediction accuracy of existing wildfire spread models and quantified the corresponding errors. The final experimental results suggest that it is necessary to judge the potential impact of data gaps based on the geographical conditions of the study area appropriately, as there is no significant pattern of behavior yet identified. This study aims to simulate the impact of data scarcity on the accuracy of wildfire spread prediction models in real scenarios, thereby enabling researchers to better understand the priority of different environmental variables for the model and identify the acceptable degree of missing data and the indispensable data attributes. It offers new insights for developing spread prediction models applicable to real-world scenarios and rational assessment of the effectiveness of model outcomes.
Full article
(This article belongs to the Special Issue Intelligent Fire Protection)
►▼
Show Figures
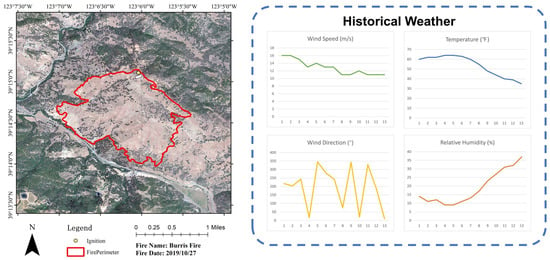
Figure 1
Open AccessArticle
YOLO-Based Models for Smoke and Wildfire Detection in Ground and Aerial Images
by
Leon Augusto Okida Gonçalves, Rafik Ghali and Moulay A. Akhloufi
Fire 2024, 7(4), 140; https://doi.org/10.3390/fire7040140 - 14 Apr 2024
Abstract
Wildland fires negatively impact forest biodiversity and human lives. They also spread very rapidly. Early detection of smoke and fires plays a crucial role in improving the efficiency of firefighting operations. Deep learning techniques are used to detect fires and smoke. However, the
[...] Read more.
Wildland fires negatively impact forest biodiversity and human lives. They also spread very rapidly. Early detection of smoke and fires plays a crucial role in improving the efficiency of firefighting operations. Deep learning techniques are used to detect fires and smoke. However, the different shapes, sizes, and colors of smoke and fires make their detection a challenging task. In this paper, recent YOLO-based algorithms are adopted and implemented for detecting and localizing smoke and wildfires within ground and aerial images. Notably, the YOLOv7x model achieved the best performance with an mAP (mean Average Precision) score of 80.40% and fast detection speed, outperforming the baseline models in detecting both smoke and wildfires. YOLOv8s obtained a high mAP of 98.10% in identifying and localizing only wildfire smoke. These models demonstrated their significant potential in handling challenging scenarios, including detecting small fire and smoke areas; varying fire and smoke features such as shape, size, and colors; the complexity of background, which can include diverse terrain, weather conditions, and vegetation; and addressing visual similarities among smoke, fog, and clouds and the the visual resemblances among fire, lighting, and sun glare.
Full article
(This article belongs to the Special Issue New Advances in Spatial Analysis of Wildfire Planning)
►▼
Show Figures
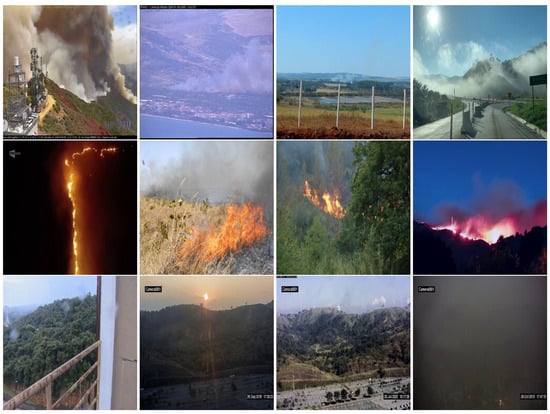
Figure 1
Open AccessArticle
Parameters Affecting the Explosion Characteristics of Hybrid Mixtures Arising from the Use of Alternative Energy Sources
by
Matous Helegda, Jiri Pokorny, Iris Helegda, Jan Skrinsky and Juraj Sinay
Fire 2024, 7(4), 139; https://doi.org/10.3390/fire7040139 - 14 Apr 2024
Abstract
Explosions of hybrid mixtures are an interesting theoretical and experimental problem in explosion sciences, because they combine the physicochemical properties of flammable gases and dusts. A hybrid mixture is composed of at least two substances in two or more states. The influence of
[...] Read more.
Explosions of hybrid mixtures are an interesting theoretical and experimental problem in explosion sciences, because they combine the physicochemical properties of flammable gases and dusts. A hybrid mixture is composed of at least two substances in two or more states. The influence of the common presence of flammable gas on the explosiveness parameters of the combustible dust itself is proven. In this study, we present the effect of higher initiation temperatures, different initial sources of initiation with different energies, and the effect of the volume of explosion chambers on the explosions of hybrid mixtures arising from the use of alternative energy sources. The experiments were carried out in 20 L and 1.00 m3 explosion chambers (according to EN 14034-1+A1:2011–EN 14034-4+A1:2011). The accredited method of the Energy Research Centre, VSB-TU Ostrava, for tests was used. The goal is to approximate the behaviour of these systems under different initiation conditions so that it is possible to avoid excessively conservative or overly optimistic results, which then affect the determination of explosion parameters for practical use. It was found that the volume of the explosion chambers in combination with the used initiation source has a fundamental influence on the course of the explosion characteristics.
Full article
(This article belongs to the Special Issue Fire and Explosions Risk in Industrial Processes)
►▼
Show Figures
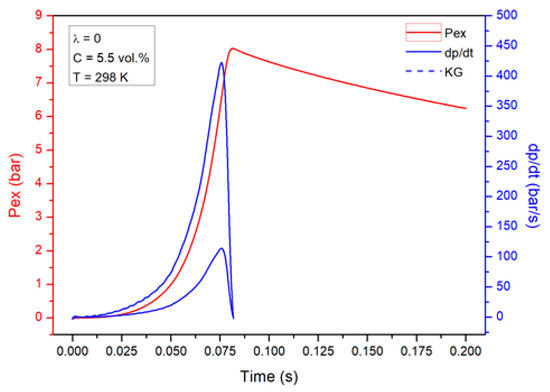
Figure 1
Open AccessArticle
Feasibility of Using Combustion-Based Methods to Quantify Saline-Based Anti-Stripping Agent in Modified Asphalt Binders
by
Riyadul Hashem Riyad, Ji Wu and Junan Shen
Fire 2024, 7(4), 138; https://doi.org/10.3390/fire7040138 - 14 Apr 2024
Abstract
►▼
Show Figures
“Anti-stripping Agents” or “adhesion promoters” can enhance the chemical affinity between asphalt and aggregate by increasing their mutual attraction. Various forms of anti-stripping agents have been proposed to mitigate pavement stripping, and siloxane-based Zychotherm is one of them. Choosing the appropriate type and
[...] Read more.
“Anti-stripping Agents” or “adhesion promoters” can enhance the chemical affinity between asphalt and aggregate by increasing their mutual attraction. Various forms of anti-stripping agents have been proposed to mitigate pavement stripping, and siloxane-based Zychotherm is one of them. Choosing the appropriate type and dose of anti-stripping additives is no doubt vital to the intended performance. Therefore, it is critically important to determine the dose of the additives used in the modification of asphalt binders. This research developed a feasible detection method that can closely measure the dose (0.05% and 0.1%) of siloxane-based anti-stripping liquid agents. Related test methods, including heat combustion test, residue visualization, burning, and ignition, were implemented. The heat combustion results showed that with the addition of the Zychotherm anti-stripping additive, the average heat combustion value decreased by 1.34% and 1.72% for 0.05% and 0.1% Zychotherm-modified binder, respectively. In the burning and ignition process, the modified binder left yellowish substances in the residue, which is an indication of the presence of Zychotherm. The weight of the yellowish residue related more to the quantity of Zychotherm in the asphalt binder.
Full article
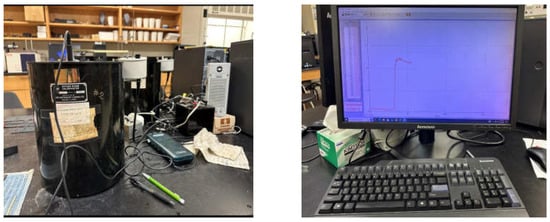
Figure 1
Open AccessArticle
The Influence of the Heat Transfer Mode on the Stability of Foam Extinguishing Agents
by
Xia Zhou, Zhihao An, Ziheng Liu, Hongjie Ha, Yixuan Li and Renming Pan
Fire 2024, 7(4), 137; https://doi.org/10.3390/fire7040137 - 12 Apr 2024
Abstract
The mass loss mechanisms of an aqueous film-forming foam (AF foam), an AR/AFFF water-soluble film-forming foam extinguishing agent (AR foam), and a Class A foam extinguishing agent (A foam) at different levels of thermal radiation, thermal convection, and heat conduction intensity were studied.
[...] Read more.
The mass loss mechanisms of an aqueous film-forming foam (AF foam), an AR/AFFF water-soluble film-forming foam extinguishing agent (AR foam), and a Class A foam extinguishing agent (A foam) at different levels of thermal radiation, thermal convection, and heat conduction intensity were studied. At a relatively low thermal radiation intensity, the liquid separation rate of the AF, AR, and A foams is related to the properties of the foam itself, such as viscosity and surface/interface tension, which are relatively independent of the external radiation heat flux of the foam. At low radiation intensity (15 kW/m2 and 25 kW/m2), the liquid separation rate of the AF and A foams is relatively stable. When the heat flux intensity is 35 kW/m2, the liquid separation rate of the AF and A foams increases notably, which may be mainly due to the rapid decrease in foam viscosity. And the mass loss behavior is dominated by liquid separation in the AF, AR, and A foams under the influence of thermal radiation and thermal convection. Under the same experimental conditions, the liquid separation rate of AF is the fastest. There is no significant difference in the evaporation rates of the three kinds of foam in the same heat conduction condition. In addition, the AR and A foams usually have a 25% longer liquid separation time (t) under thermal radiation and thermal convection, and the thermal stability is better than AF foam. The temperature reached by the AF foam layer under thermal convection was lower than that of the AR and A foams, and the time for the foam layer to reach the highest temperature under heat conduction was longer than that of the AR and A foams.
Full article
(This article belongs to the Special Issue Fire Extinguishing Agent and Application)
►▼
Show Figures
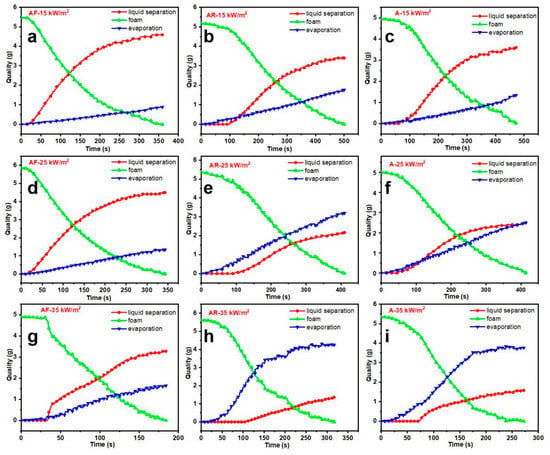
Figure 1
Open AccessArticle
Predictive Modeling of Fire Incidence Using Deep Neural Networks
by
Cheng-Yu Ku and Chih-Yu Liu
Fire 2024, 7(4), 136; https://doi.org/10.3390/fire7040136 - 12 Apr 2024
Abstract
To achieve successful prevention of fire incidents originating from human activities, it is imperative to possess a thorough understanding. This paper introduces a machine learning approach, specifically utilizing deep neural networks (DNN), to develop predictive models for fire occurrence in Keelung City, Taiwan.
[...] Read more.
To achieve successful prevention of fire incidents originating from human activities, it is imperative to possess a thorough understanding. This paper introduces a machine learning approach, specifically utilizing deep neural networks (DNN), to develop predictive models for fire occurrence in Keelung City, Taiwan. It investigates ten factors across demographic, architectural, and economic domains through spatial analysis and thematic maps generated from geographic information system data. These factors are then integrated as inputs for the DNN model. Through 50 iterations, performance indices including the coefficient of determination (R2), root mean square error (RMSE), variance accounted for (VAF), prediction interval (PI), mean absolute error (MAE), weighted index (WI), weighted mean absolute percentage error (WMAPE), Nash–Sutcliffe efficiency (NS), and the ratio of performance to deviation (RPD) are computed, with average values of 0.89, 7.30 10−2, 89.21, 1.63, 4.90 10−2, 0.97, 2.92 10−1, 0.88, and 4.84, respectively. The model’s predictions, compared with historical data, demonstrate its efficacy. Additionally, this study explores the impact of various urban renewal strategies using the DNN model, highlighting the significant influence of economic factors on fire incidence. This underscores the importance of economic factors in mitigating fire incidents and emphasizes their consideration in urban renewal planning.
Full article
(This article belongs to the Special Issue Machine Learning (ML) and Deep Learning (DL) Applications in Wildfire Science: Principles, Progress and Prospects)
►▼
Show Figures
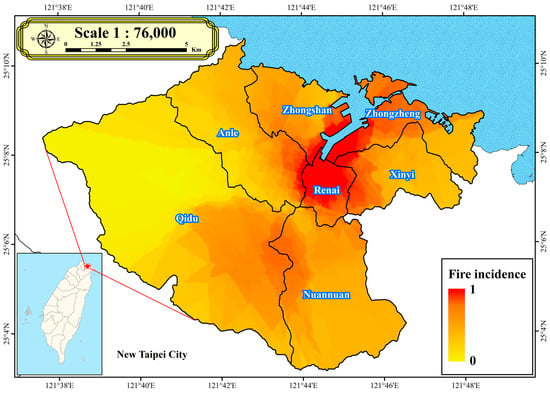
Figure 1
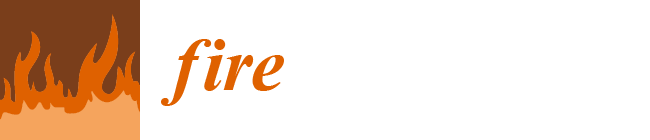
Journal Menu
► ▼ Journal Menu-
- Fire Home
- Aims & Scope
- Editorial Board
- Reviewer Board
- Topical Advisory Panel
- Instructions for Authors
- Special Issues
- Topics
- Sections & Collections
- Article Processing Charge
- Indexing & Archiving
- Editor’s Choice Articles
- Most Cited & Viewed
- Journal Statistics
- Journal History
- Journal Awards
- Society Collaborations
- Editorial Office
Journal Browser
► ▼ Journal BrowserHighly Accessed Articles
Latest Books
E-Mail Alert
News
Topics
Topic in
AI, BDCC, Fire, GeoHazards, Remote Sensing
AI for Natural Disasters Detection, Prediction and Modeling
Topic Editors: Moulay A. Akhloufi, Mozhdeh ShahbaziDeadline: 25 July 2025

Conferences
Special Issues
Special Issue in
Fire
Effects of Wildfire on the Biota
Guest Editor: Neil BurrowsDeadline: 20 May 2024
Special Issue in
Fire
Firefighting Approaches and Extreme Wildfires
Guest Editor: Fantina TedimDeadline: 31 May 2024
Topical Collections
Topical Collection in
Fire
Technical Forum for Fire Science Laboratory and Field Methods
Collection Editors: Claire Belcher, David M.J.S. Bowman, Evan Ellicott, Peter Hamlington, Chad Hoffman, William M. Jolly, Rodman Linn, Sara McAllister, Joseph O'Brien, Albert Simeoni, Alistair M. S. Smith, Wojciech Węgrzyński
Topical Collection in
Fire
Diversity Leaders in Fire Science
Collection Editors: Alistair M. S. Smith, Tiago Miguel Ferreira, Natasha Ribeiro, Grant Williamson, Christine Eriksen