Topic Menu
► Topic MenuTopic Editors


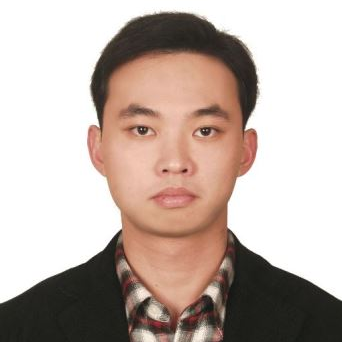
Applications and Development of Underwater Robotics and Underwater Vision Technology
Topic Information
Dear Colleagues,
In today’s world, the ocean is one of the most important areas for human exploration and development. Underwater vision, as a cross-disciplinary field related to underwater environments, has a wide range of applications in marine resource development, marine biology research, underwater detection and control, and other fields.
In terms of marine resource development, underwater vision technology is a valuable tool for marine oil exploration and deep-sea mineral resource development. For example, high-precision underwater vision systems on underwater robots can facilitate oil exploration and development in deep-sea environments. Moreover, these robots can be utilized for the exploration and development of seabed mineral resources, enabling the development and utilization of deep-sea resources.
Regarding marine biology research, underwater vision technology is useful for observing and studying marine organisms. High-definition underwater cameras on underwater robots can capture and observe marine organisms in the ocean. Furthermore, these cameras can aid in the study of deep-sea organisms, which enables scientists to comprehend the distribution of biological communities and ecosystems in deep-sea environments.
As for underwater detection and control, underwater vision technology plays a vital role in underwater target detection, underwater 3D modeling, and more. For example, underwater robots equipped with underwater sonars and cameras can detect and identify underwater targets in underwater environments. Moreover, these robots can create 3D models of underwater scenes, providing a visualization tool for the detection and study of underwater environments.
Therefore, the application of underwater vision in the marine field has broad prospects and great significance in promoting the development of the marine field. In order to promote the development of the underwater vision field, we will edit a Special Issue on underwater vision, inviting experts and scholars to share both their research results and the latest developments in the field.
- We welcome submissions of papers related to the following areas:
- Underwater robot vision systems;
- Underwater image enhancement and processing techniques;
- Underwater object detection and recognition;
- Underwater 3D reconstruction techniques;
- Underwater optical imaging and laser scanning technologies;
- Underwater physical environment modeling and simulation;
- Underwater acoustic imaging and sonar technologies;
- Underwater communication and networking technologies.
Dr. Jingchun Zhou
Dr. Wenqi Ren
Dr. Qiuping Jiang
Dr. Yan-Tsung Peng
Topic Editors
Keywords
- computer vision
- image processing
- underwater vision
- underwater image enhancement/restoration
- underwater robot
- underwater imaging
Participating Journals
Journal Name | Impact Factor | CiteScore | Launched Year | First Decision (median) | APC |
---|---|---|---|---|---|
![]()
Journal of Marine Science and Engineering
|
2.7 | 4.4 | 2013 | 16.4 Days | CHF 2600 |
![]()
Machines
|
2.1 | 3.0 | 2013 | 15.5 Days | CHF 2400 |
![]()
Remote Sensing
|
4.2 | 8.3 | 2009 | 23.9 Days | CHF 2700 |
![]()
Robotics
|
2.9 | 6.7 | 2012 | 21 Days | CHF 1800 |
![]()
Sensors
|
3.4 | 7.3 | 2001 | 18.6 Days | CHF 2600 |
Preprints.org is a multidisciplinary platform offering a preprint service designed to facilitate the early sharing of your research. It supports and empowers your research journey from the very beginning.
MDPI Topics is collaborating with Preprints.org and has established a direct connection between MDPI journals and the platform. Authors are encouraged to take advantage of this opportunity by posting their preprints at Preprints.org prior to publication:
- Share your research immediately: disseminate your ideas prior to publication and establish priority for your work.
- Safeguard your intellectual contribution: Protect your ideas with a time-stamped preprint that serves as proof of your research timeline.
- Boost visibility and impact: Increase the reach and influence of your research by making it accessible to a global audience.
- Gain early feedback: Receive valuable input and insights from peers before submitting to a journal.
- Ensure broad indexing: Web of Science (Preprint Citation Index), Google Scholar, Crossref, SHARE, PrePubMed, Scilit and Europe PMC.